Analysis of Part-Of-Speech Tagging of Historical German Texts
DATeCH(2017)
摘要
The amount of data in contemporary digital corpora is too large to be processed manually, which increases the necessity for computer linguistic tools in humanities. However, the processing of natural languages is a challenge for automatic tools, because languages are used heterogeneously. To process a text, often taggers are used that are trained on a standardized language variety (e.g. recent newspaper articles). Unfortunately, these training data often differ from the target texts (i.e. the text on which a trained model later is applied) in terms of language variety and register, which is especially the case for historical texts. Therefore, additional, manual analyses are usually inevitable. Training tools on the target language variety, however, can improve the results of these tools so that the manual prost-processing could be avoided. Thus, the need to process large datasets of diachronic texts and to obtain accurate results in a short time-span requires an adaptable approach. The present paper suggests this adaptable approach, by training taggers on a target language variety, to improve the accuracy of the structure of historical German corpora at the level of part-of-speech-tagging (hereafter POS-tagging). We trained four taggers (Perceptron tagger [26], Hidden Markov Model (HMM) [1], Conditional Random Fields (CRF) [13], and Unigram [21]) each on data from three different literary periods: Baroque (1600-1700), Romanticism (1790-1840) and Modernism (1880-1930). Compared with pre-tagged data, we obtained a maximum accuracy in POS-tagging of 98.3% for a single period (Modernism with Perceptron trained on Modernism) and a maximum mean accuracy for all three periods of 94.3% (Perceptron trained on Romanticism). Compared with manually tagged data, we obtained a maximum accuracy for one period of 96.8% (Romanticism with CRF and HMM trained on Romanticism) and a maximum mean accuracy for all three periods of 92.3% (Perceptron trained on Romanticism). In spite of the heterogeneity of literary data, these results demonstrate a high performance of the POS-taggers if the models are trained on target language varieties. Therefore, this adaptable approach provides reliable data allowing the use of taggers for analysis of different historical texts.
更多查看译文
AI 理解论文
溯源树
样例
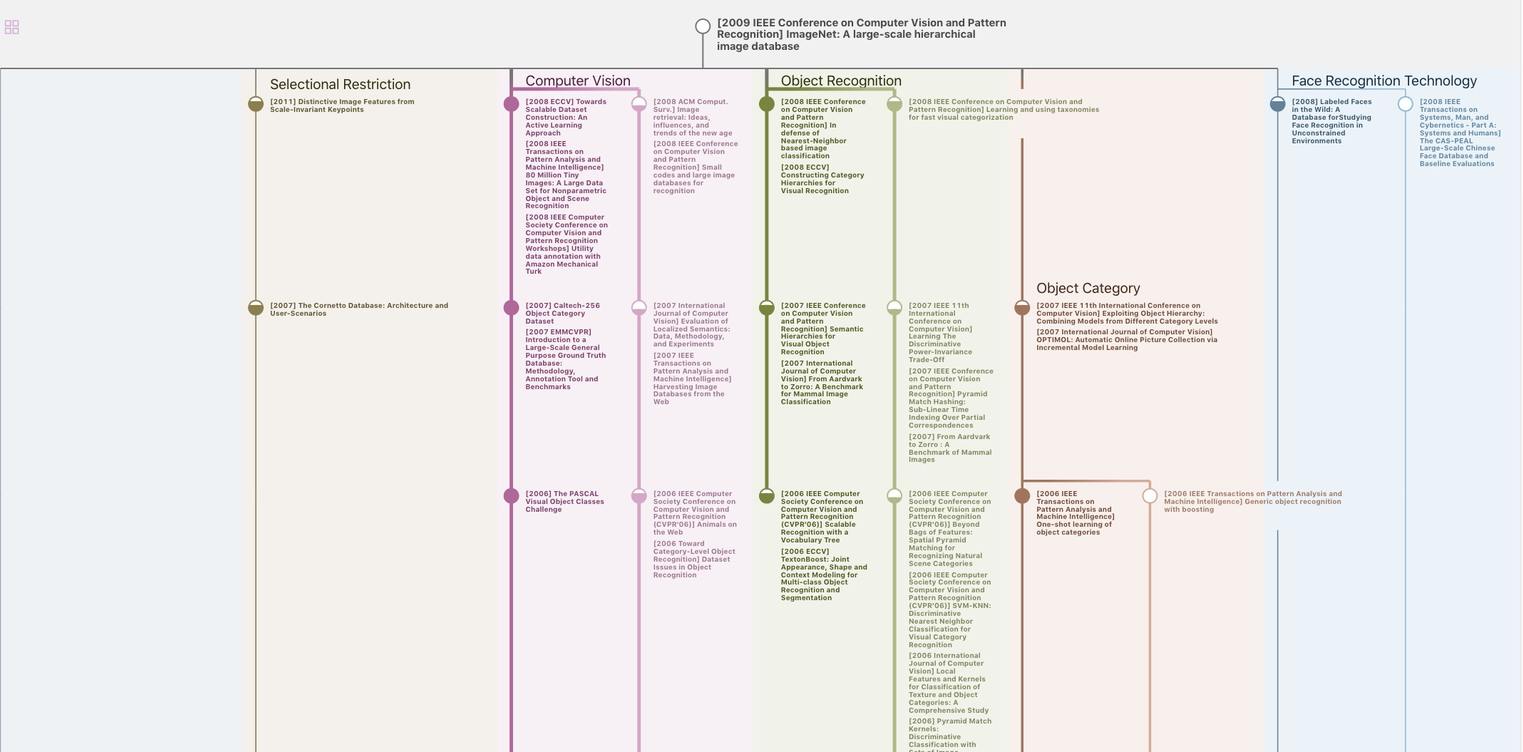
生成溯源树,研究论文发展脉络
Chat Paper
正在生成论文摘要