Seq2seq Fingerprint: An Unsupervised Deep Molecular Embedding for Drug Discovery
BCB(2017)
摘要
Many of today's drug discoveries require expertise knowledge and insanely expensive biological experiments for identifying the chemical molecular properties. However, despite the growing interests of using supervised machine learning algorithms to automatically identify those chemical molecular properties, there is little advancement of the performance and accuracy due to the limited amount of training data. In this paper, we propose a novel unsupervised molecular embedding method, providing a continuous feature vector for each molecule to perform further tasks, e.g., solubility classification. In the proposed method, a multi-layered Gated Recurrent Unit (GRU) network is used to map the input molecule into a continuous feature vector of fixed dimensionality, and then another deep GRU network is employed to decode the continuous vector back to the original molecule. As a result, the continuous encoding vector is expected to contain rigorous and enough information to recover the original molecule and predict its chemical properties. The proposed embedding method could utilize almost unlimited molecule data for the training phase. With sufficient information encoded in the vector, the proposed method is also robust and task-insensitive. The performance and robustness are confirmed and interpreted in our extensive experiments.
更多查看译文
关键词
Unsupervised Learning,Structured Prediction,Learning Representation,Sequence to Sequence Learning,Deep Learning,Drug Discovery,Virtual Screening,Molecular Representation,Imaging,Computational Biology
AI 理解论文
溯源树
样例
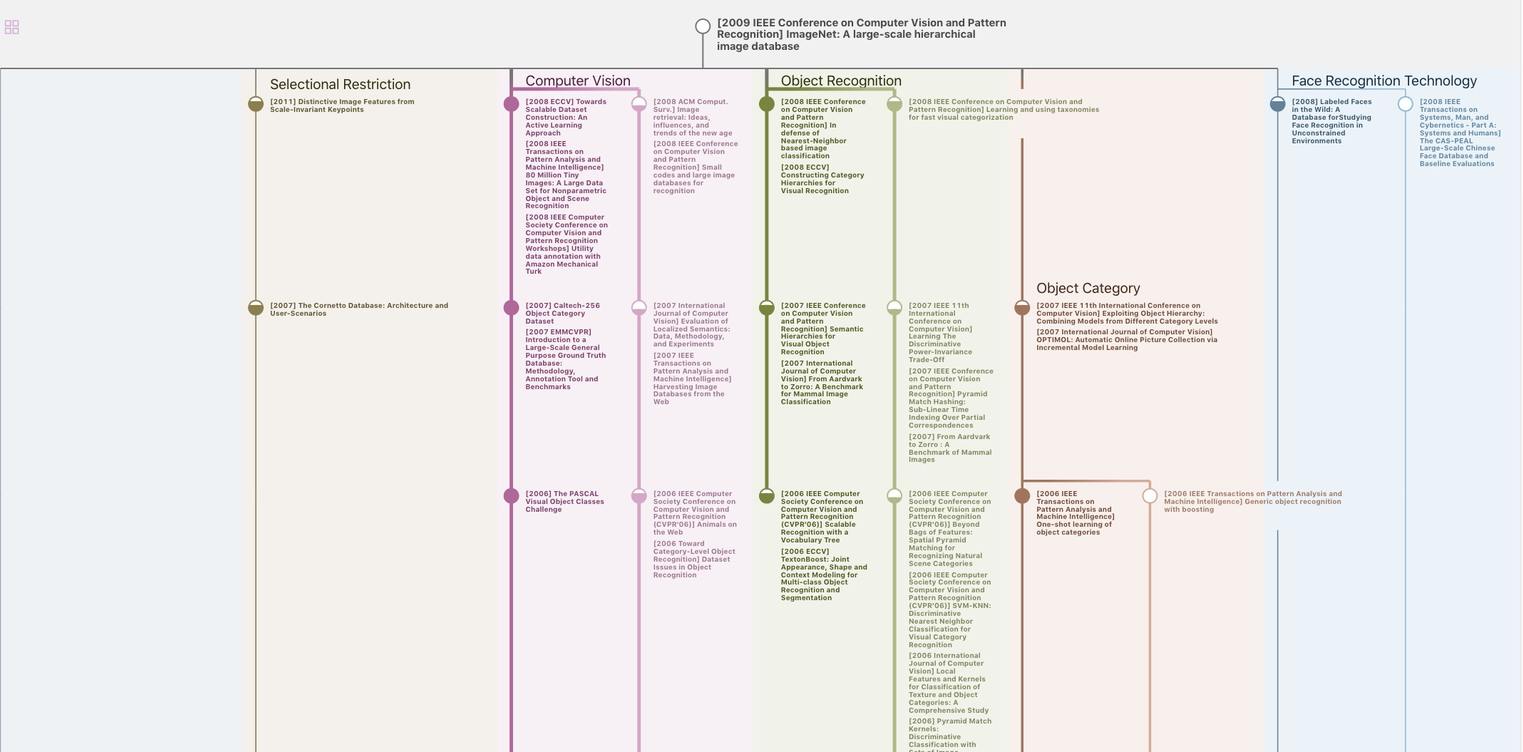
生成溯源树,研究论文发展脉络
Chat Paper
正在生成论文摘要