Chrs: Cold Start Recommendation Across Multiple Heterogeneous Information Networks
IEEE ACCESS(2017)
摘要
Nowadays, people are overwhelmingly exposed to various kinds of information from different information networks. In order to recommend users with the information entities that match their interests, many recommendation methods have been proposed so far. And some of these methods have explored different ways to utilize different kinds of auxiliary information to deal with the information sparsity problem of user feedbacks. However, as a special kind of information sparsity problem, the cold start problem is still a big challenge not well-solved yet in the recommendation problem. In order to tackle the cold start challenge, in this paper, we propose a novel recommendation model, which integrates the auxiliary information in multiple heterogeneous information networks (HINs), namely the Cross-HIN Recommendation System (CHRS). By utilizing the rich heterogeneous information from meta-paths, the CHRS is able to calculate the similarities of information entities and apply the calculated similarity scores in the recommendation process. For the information entities shared among multiple information networks, CHRS transfers item latent information from other networks to help the recommendation task in a given network. During the information transfer process, CHRS applies a domain adaptation matrix to tackle the domain difference problem. We conduct experiments to compare our CHRS method with several widely employed or the state-of-art recommendation models, and the experimental results demonstrate that our method outperforms the baseline methods in addressing the cold start recommendation problem.
更多查看译文
关键词
Recommendation system,"cold start" problem,heterogeneous information networks,information transfer
AI 理解论文
溯源树
样例
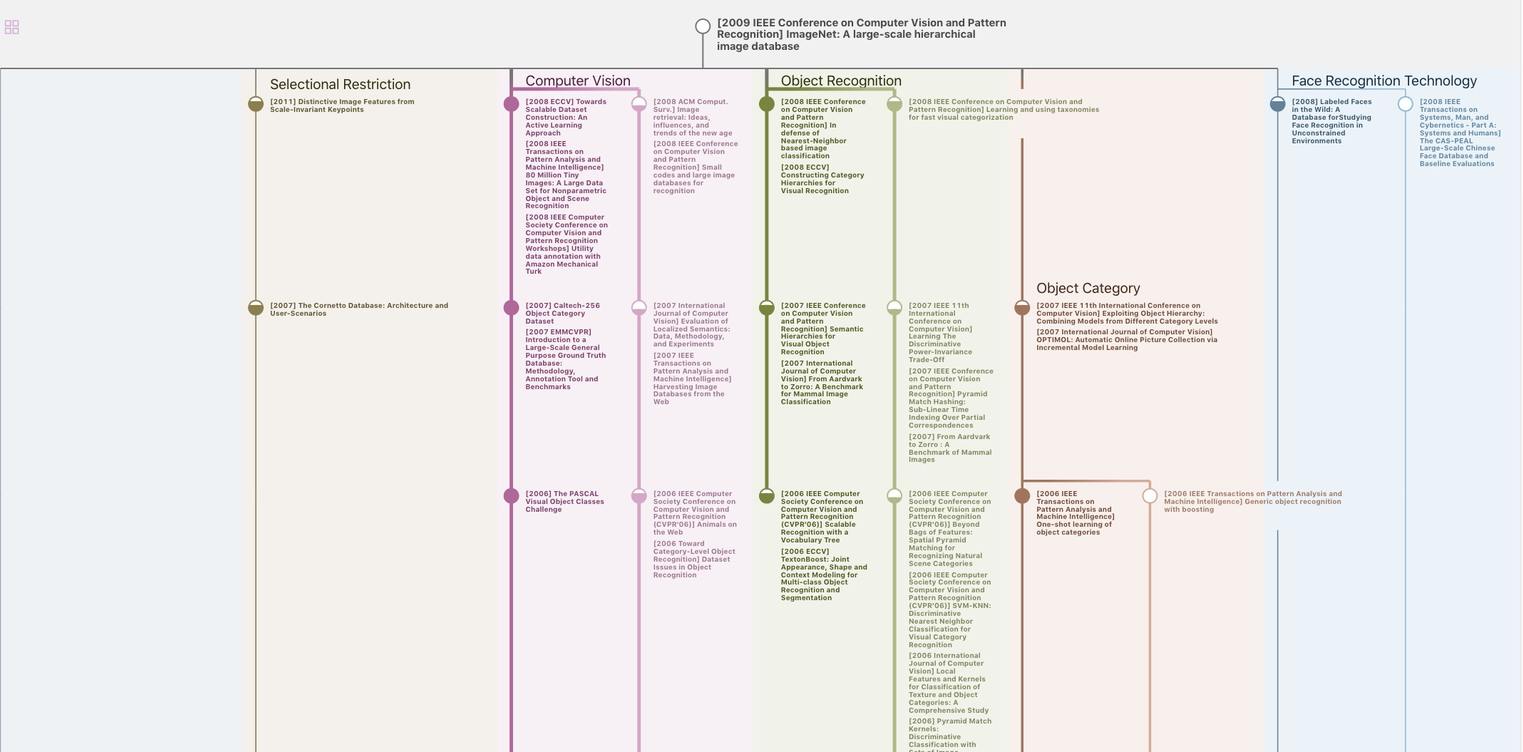
生成溯源树,研究论文发展脉络
Chat Paper
正在生成论文摘要