Benchmarking different clustering algorithms on functional data
Adv. Data Analysis and Classification(2016)
摘要
Theoretical knowledge of clustering functions is still scarce and only few models are available in form of applicable code. In literature, most methods are based on the projection of the functions onto a basis and building fixed or random effects models of the basis coefficients. They involve various parameters, among them number of basis functions, projection dimension, number of iterations etc. They usually work well on the data presented in the articles, but their performance has in most cases not been tested objectively on other data sets, nor against each other. The purpose of this paper is to give an overview of several existing methods to cluster functional data. An outline of their theoretic concepts is given and the meaning of their hyperparameters is explained. A simulation study was set up to analyze the parameters’ efficiency and sensitivity on different types of data sets, that were registered on regular and on irregular grids. For each method, a linear model of the clustering results was evaluated with different parameter levels as predictors. Later, the methods’ performances were compared to each other with the help of a visualization tool, to identify which method works the best on a specific kind of data.
更多查看译文
关键词
46 Functional analysis, 62 Statistics, Benchmarking, Functional clustering, Hyperparameters
AI 理解论文
溯源树
样例
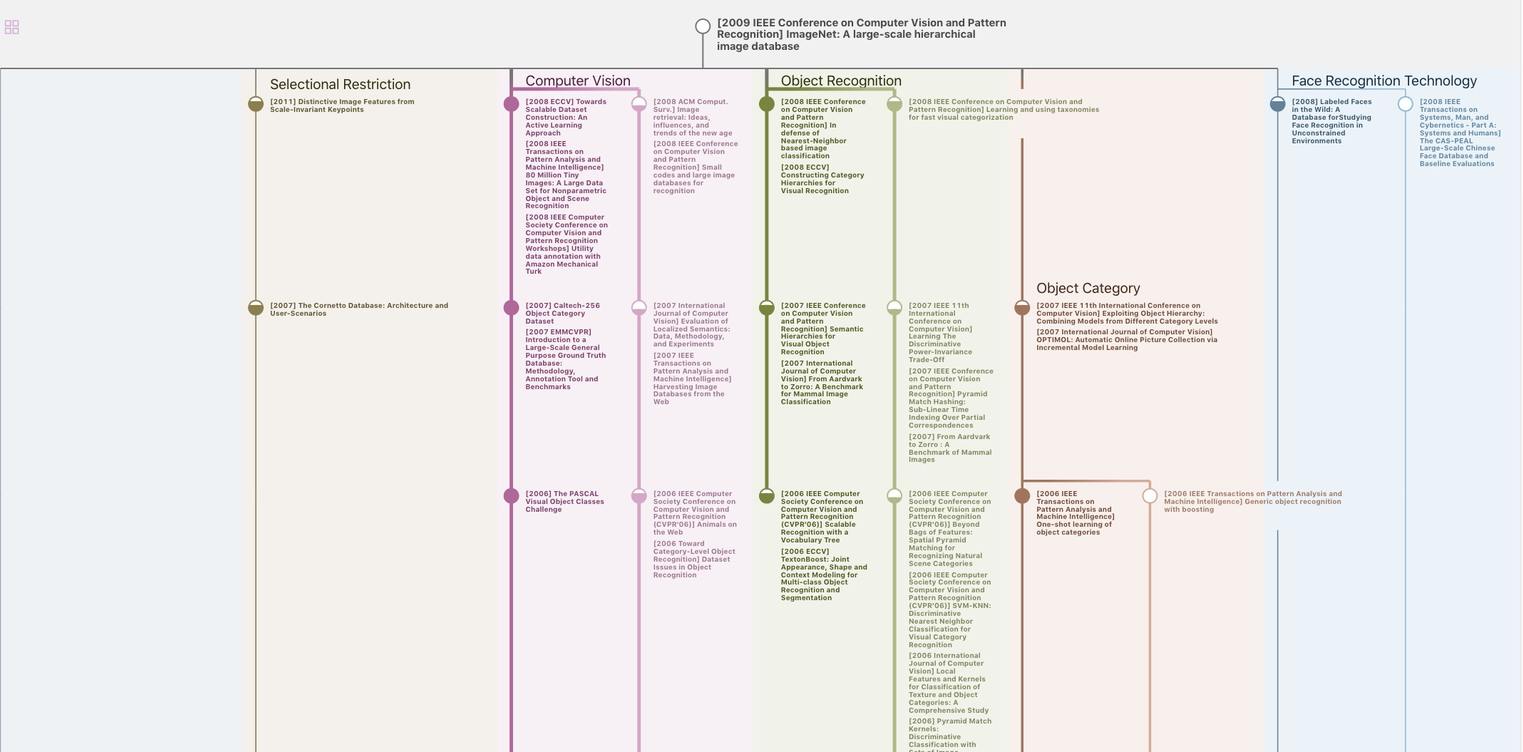
生成溯源树,研究论文发展脉络
Chat Paper
正在生成论文摘要