Optimal Testing of Self-Driving Cars.
arXiv: Artificial Intelligence(2017)
摘要
Automotive manufacturers attempting to bring autonomous vehicles to market must make the case that their product is sufficiently safe for public deployment. Much of this case will likely rely upon outcomes from real-world testing, requiring manufacturers to be strategic about how they allocate testing resources in order to maximize their chances of demonstrating system safety. This work frames the partially observable and belief-dependent problem of autonomous vehicle test scheduling as a Markov decision process, which can be solved efficiently to yield exact, optimal manufacturer testing policies. By solving for policies over a wide range of problem formulations, we are able to provide high-level guidance for manufacturers and regulators on issues relating to the real-world testing of autonomous vehicles. This guidance spans an array of topics, including circumstances under which manufacturers should continue testing despite observed incidents, when manufacturers should test aggressively, and when regulators should increase or reduce the real-world testing requirements for an autonomous vehicle.
更多查看译文
AI 理解论文
溯源树
样例
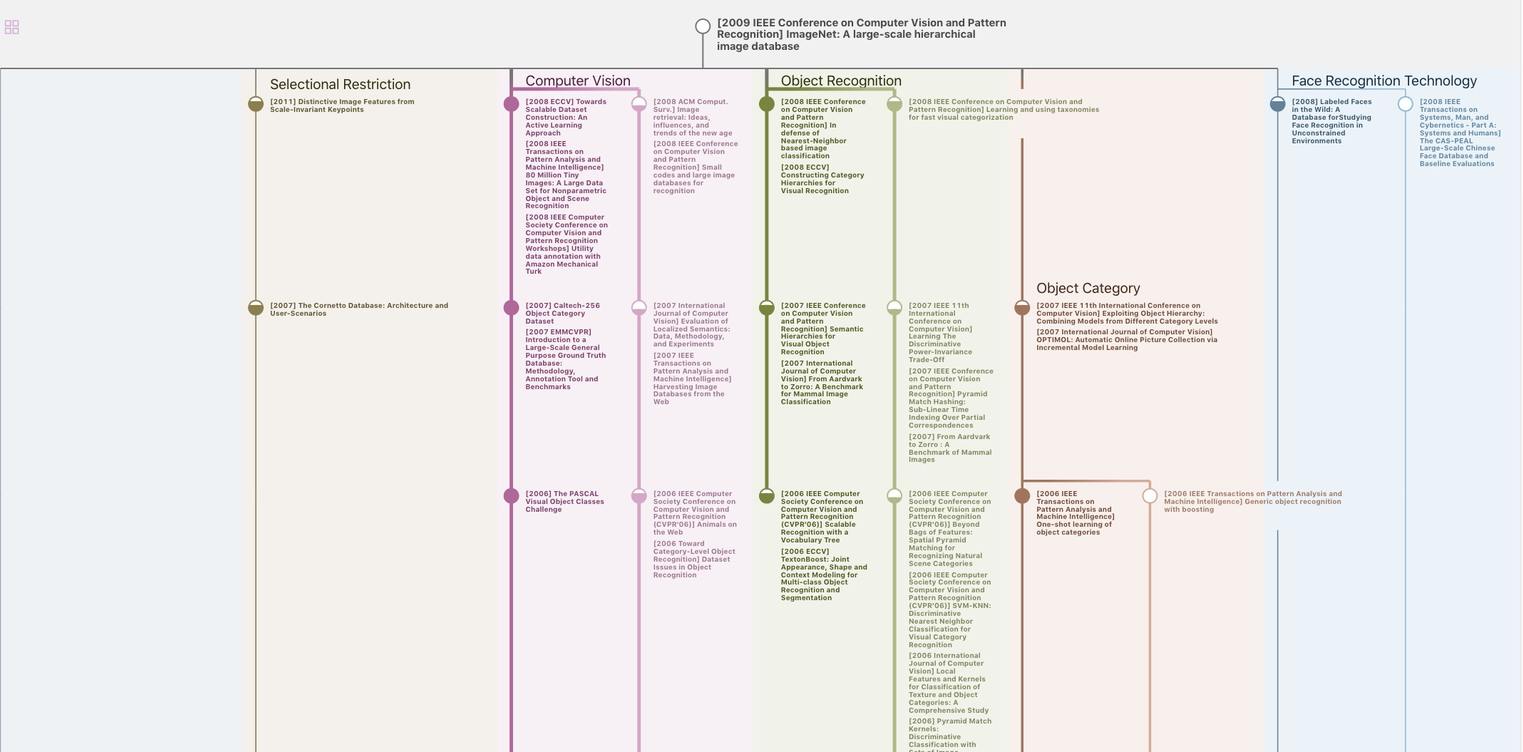
生成溯源树,研究论文发展脉络
Chat Paper
正在生成论文摘要