Empirical Bayes Estimators For High-Dimensional Sparse Vectors
INFORMATION AND INFERENCE-A JOURNAL OF THE IMA(2020)
摘要
The problem of estimating a high-dimensional sparse vector theta epsilon R-n from an observation in i.i.d. Gaussian noise is considered. The performance is measured using squared-error loss. An empirical Bayes shrinkage estimator, derived using a Bernoulli-Gaussian prior, is analyzed and compared with the well-known softthresholding estimator. We obtain concentration inequalities for the Stein's unbiased risk estimate and the loss function of both estimators. The results show that for large n, both the risk estimate and the loss function concentrate on deterministic values close to the true risk. Depending on the underlying theta, either the proposed empirical Bayes (eBayes) estimator or soft thresholding may have smaller loss. We consider a hybrid estimator that attempts to pick the better of the soft-thresholding estimator and the eBayes estimator by comparing their risk estimates. It is shown that (i) the loss of the hybrid estimator concentrates on the minimum of the losses of the two competing estimators, and (ii) the risk of the hybrid estimator is within order 1/root n of the minimum of the two risks. Simulation results are provided to support the theoretical results. Finally, we use the eBayes and hybrid estimators as denoisers in the approximate message passing algorithm for compressed sensing, and show that their performance is superior to the soft-thresholding denoiser in a wide range of settings.
更多查看译文
关键词
sparse estimation, shrinkage estimators, Stein's unbiased risk estimate, soft-thresholding, large deviations, concentration inequalities
AI 理解论文
溯源树
样例
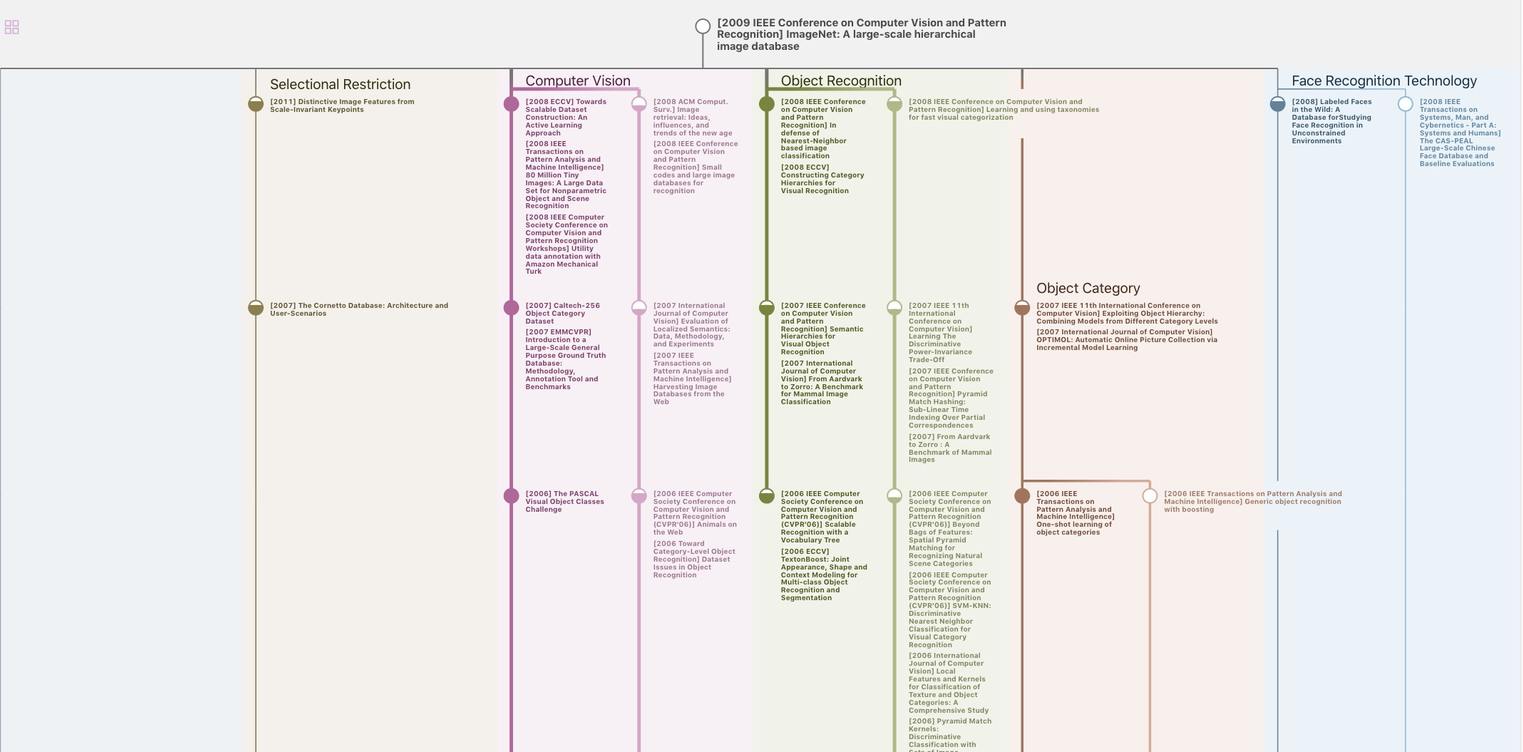
生成溯源树,研究论文发展脉络
Chat Paper
正在生成论文摘要