Visual Relationship Detection with Internal and External Linguistic Knowledge Distillation
2017 IEEE International Conference on Computer Vision (ICCV)(2017)
摘要
Understanding visual relationships involves identifying the subject, the object, and a predicate relating them. We leverage the strong correlations between the predicate and the (subj,obj) pair (both semantically and spatially) to predict the predicates conditioned on the subjects and the objects. Modeling the three entities jointly more accurately reflects their relationships, but complicates learning since the semantic space of visual relationships is huge and the training data is limited, especially for the long-tail relationships that have few instances. To overcome this, we use knowledge of linguistic statistics to regularize visual model learning. We obtain linguistic knowledge by mining from both training annotations (internal knowledge) and publicly available text, e.g., Wikipedia (external knowledge), computing the conditional probability distribution of a predicate given a (subj,obj) pair. Then, we distill the knowledge into a deep model to achieve better generalization. Our experimental results on the Visual Relationship Detection (VRD) and Visual Genome datasets suggest that with this linguistic knowledge distillation, our model outperforms the state-of-the-art methods significantly, especially when predicting unseen relationships (e.g., recall improved from 8.45% to 19.17% on VRD zero-shot testing set).
更多查看译文
关键词
semantic space,linguistic statistics,visual model learning,Wikipedia,conditional probability distribution,deep model,linguistic knowledge distillation,visual relationship detection,visual genome datasets,training annotation
AI 理解论文
溯源树
样例
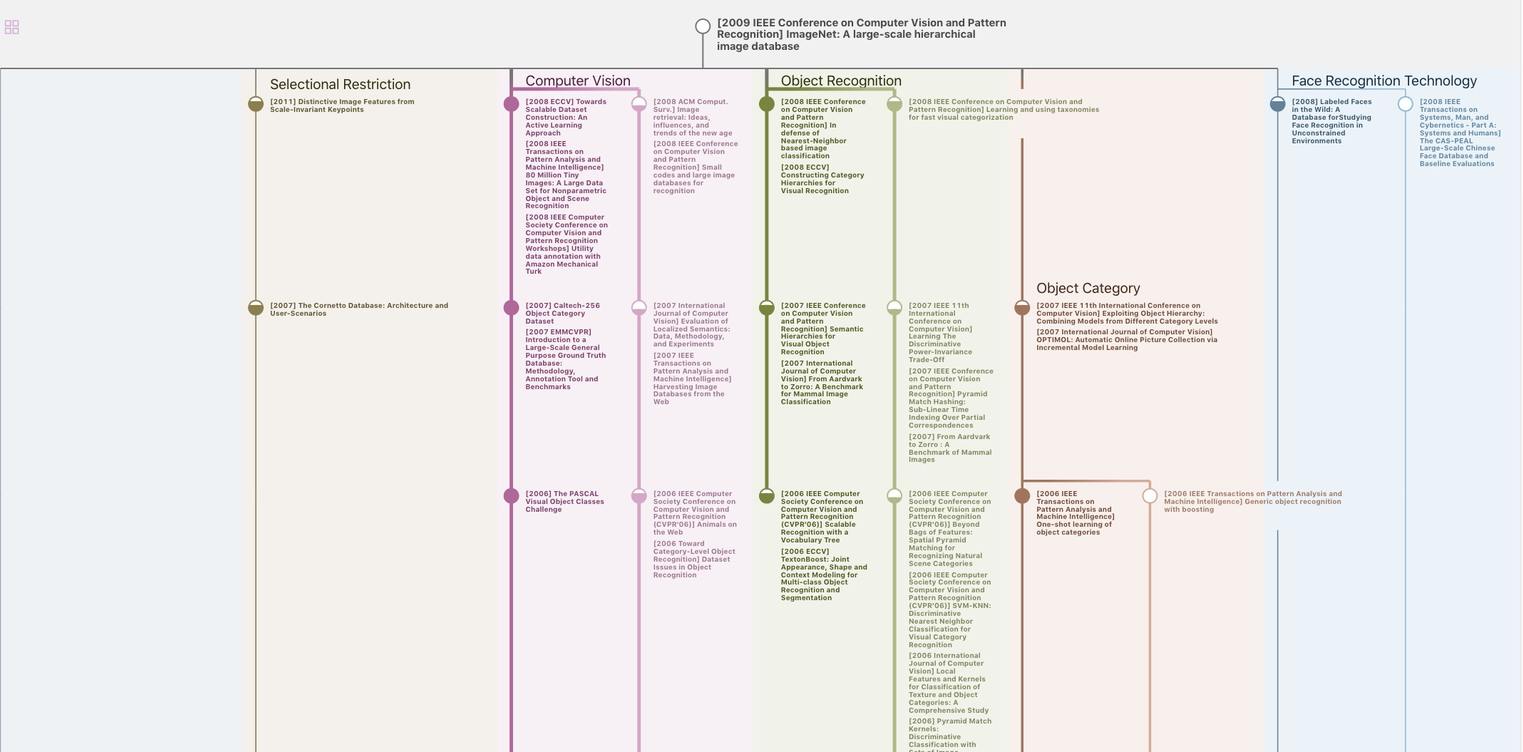
生成溯源树,研究论文发展脉络
Chat Paper
正在生成论文摘要