Big data transfer optimization based on offline knowledge discovery and adaptive sampling
arXiv: Distributed, Parallel, and Cluster Computing(2017)
摘要
The amount of data moved over dedicated and non-dedicated network links increases much faster than the increase in the network capacity, but the current solutions fail to guarantee even the promised achievable transfer throughputs. In this paper, we propose a novel dynamic throughput optimization model based on mathematical modeling with offline knowledge discovery/analysis and adaptive online decision making. In offline analysis, we mine historical transfer logs to perform knowledge discovery about the transfer characteristics. Online phase uses the discovered knowledge from the offline analysis along with real-time investigation of the network condition to optimize the protocol parameters. As real-time investigation is expensive and provides partial knowledge about the current network status, our model uses historical knowledge about the network and data to reduce the real-time investigation overhead while ensuring near optimal throughput for each transfer. Our novel approach is tested over different networks with different datasets and outperformed its closest competitor by 1.7× and the default case by 5×. It also achieved up to 93% accuracy compared with the optimal achievable throughput possible on those networks.
更多查看译文
关键词
big data transfer optimization,adaptive sampling,nondedicated network links,network capacity,mathematical modeling,adaptive online decision making,offline analysis,transfer characteristics,transfer throughputs,offline knowledge discovery,dynamic throughput optimization,historical transfer log mining,protocol parameters
AI 理解论文
溯源树
样例
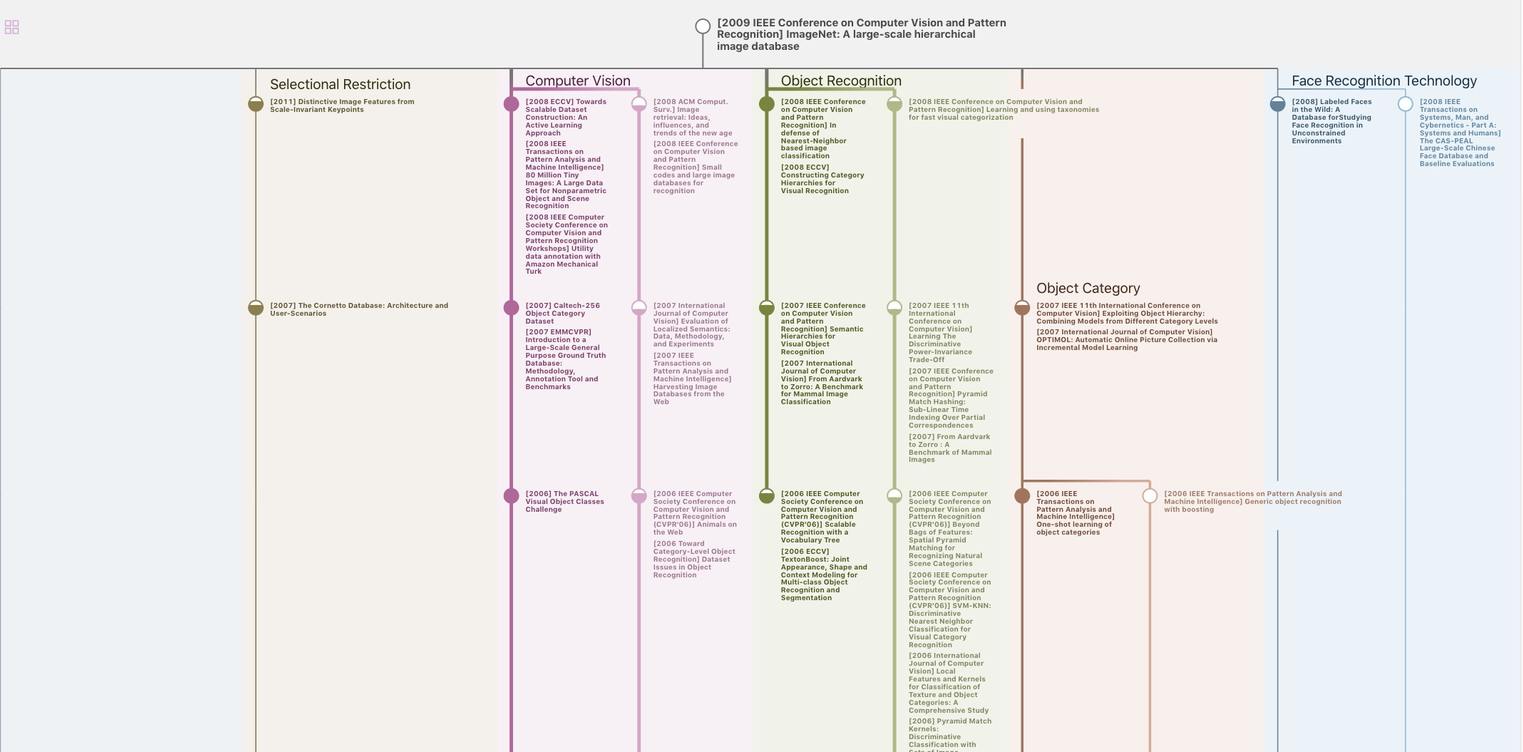
生成溯源树,研究论文发展脉络
Chat Paper
正在生成论文摘要