Story Generation from Sequence of Independent Short Descriptions
arXiv: Computation and Language(2017)
摘要
Existing Natural Language Generation (NLG) systems are weak AI systems and exhibit limited capabilities when language generation tasks demand higher levels of creativity, originality and brevity. Effective solutions or, at least evaluations of modern NLG paradigms for such creative tasks have been elusive, unfortunately. This paper introduces and addresses the task of coherent story generation from independent descriptions, describing a scene or an event. Towards this, we explore along two popular text-generation paradigms – (1) Statistical Machine Translation (SMT), posing story generation as a translation problem and (2) Deep Learning, posing story generation as a sequence to sequence learning problem. In SMT, we chose two popular methods such as phrase based SMT (PB-SMT) and syntax based SMT (SYNTAX-SMT) to `translate' the incoherent input text into stories. We then implement a deep recurrent neural network (RNN) architecture that encodes sequence of variable length input descriptions to corresponding latent representations and decodes them to produce well formed comprehensive story like summaries. The efficacy of the suggested approaches is demonstrated on a publicly available dataset with the help of popular machine translation and summarization evaluation metrics.
更多查看译文
AI 理解论文
溯源树
样例
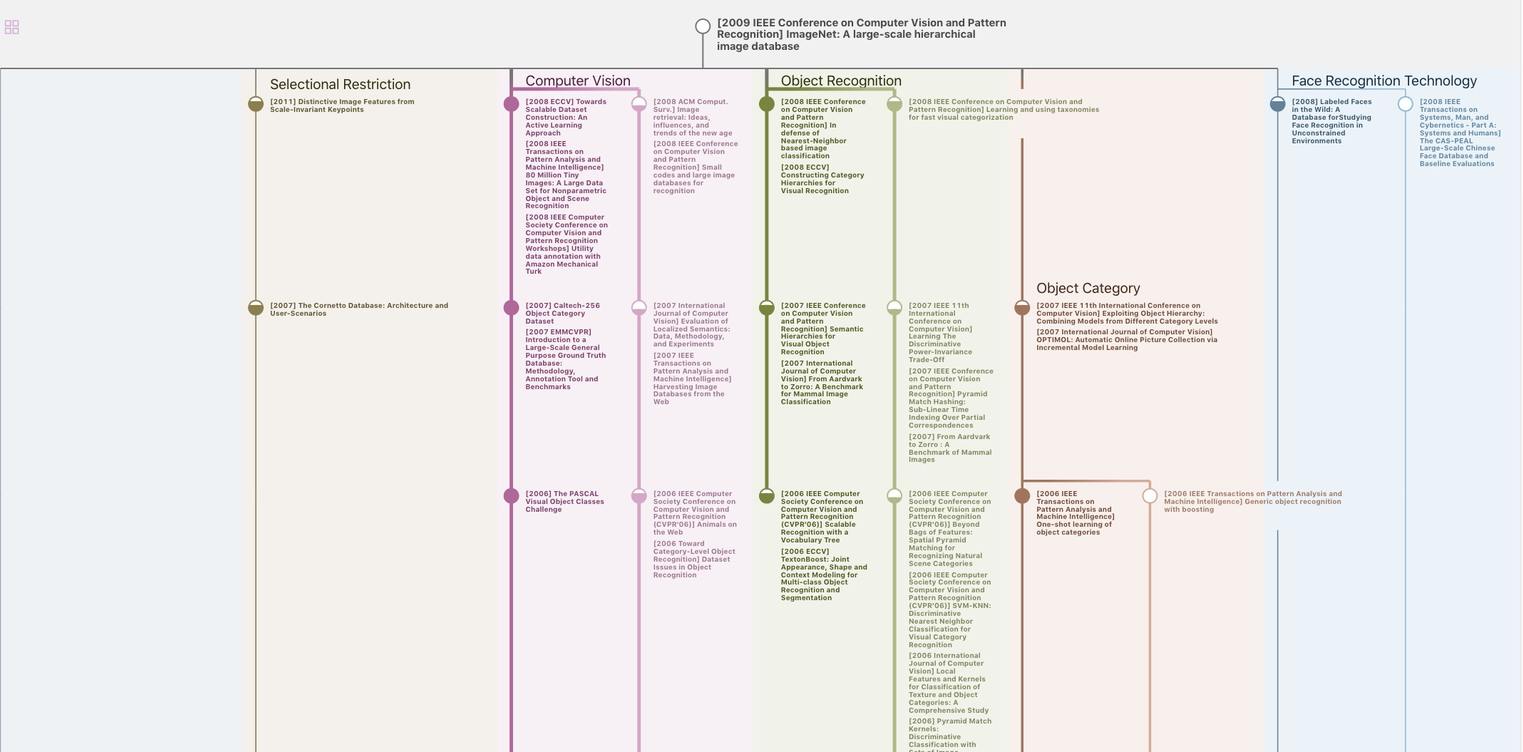
生成溯源树,研究论文发展脉络
Chat Paper
正在生成论文摘要