Cognitive Load Classification In Learning Tasks From Hemodynamic Responses Using Type-2 Fuzzy Sets
2017 IEEE INTERNATIONAL CONFERENCE ON FUZZY SYSTEMS (FUZZ-IEEE)(2017)
摘要
Although there exist recent works on fMRI based cognitive learning, there is a dearth of literature on fNIRs based studies on learning and memory. This paper provides a novel study on the cognitive load detection of subjects engaged in symbol-meaning associative learning tasks from the direct measurement of the hemodynamic response of the brain. The hemodynamic response collected during symbol-meaning associative learning tasks by subjects are pre-processed (filtered from artifacts) for extraction of 112-dimensional features, which are reduced to 20 dimensions by a meta-heuristic optimization algorithm for subsequent transfer to a interval type-2 fuzzy classifier to classify three levels of cognitive loads (High, Low and Moderate) borne by the subjects at different time slots of the learning task. Analysis undertaken reveals that the type-2 fuzzy classifier with the proposed feature selection mechanism has a high performance in classification of the cognitive loads over 89%. Experimental analysis further reveals that the transfer of brain activation from orbitofrontal to ventrolateral prefrontal cortex takes place during transition of cognitive load from high to low. In addition, the activation of dorsolateral prefrontal cortex is also reduced during low cognitive load of subjects. These findings would offer justification of inability to handle high cognitive loads by people with under-developed/damaged orbitofrontal and dorsolateral prefrontal cortex.
更多查看译文
关键词
fNIRs,Hemodynamic response,Type-2 fuzzy set induced classifier,Meta-heuristic feature selection
AI 理解论文
溯源树
样例
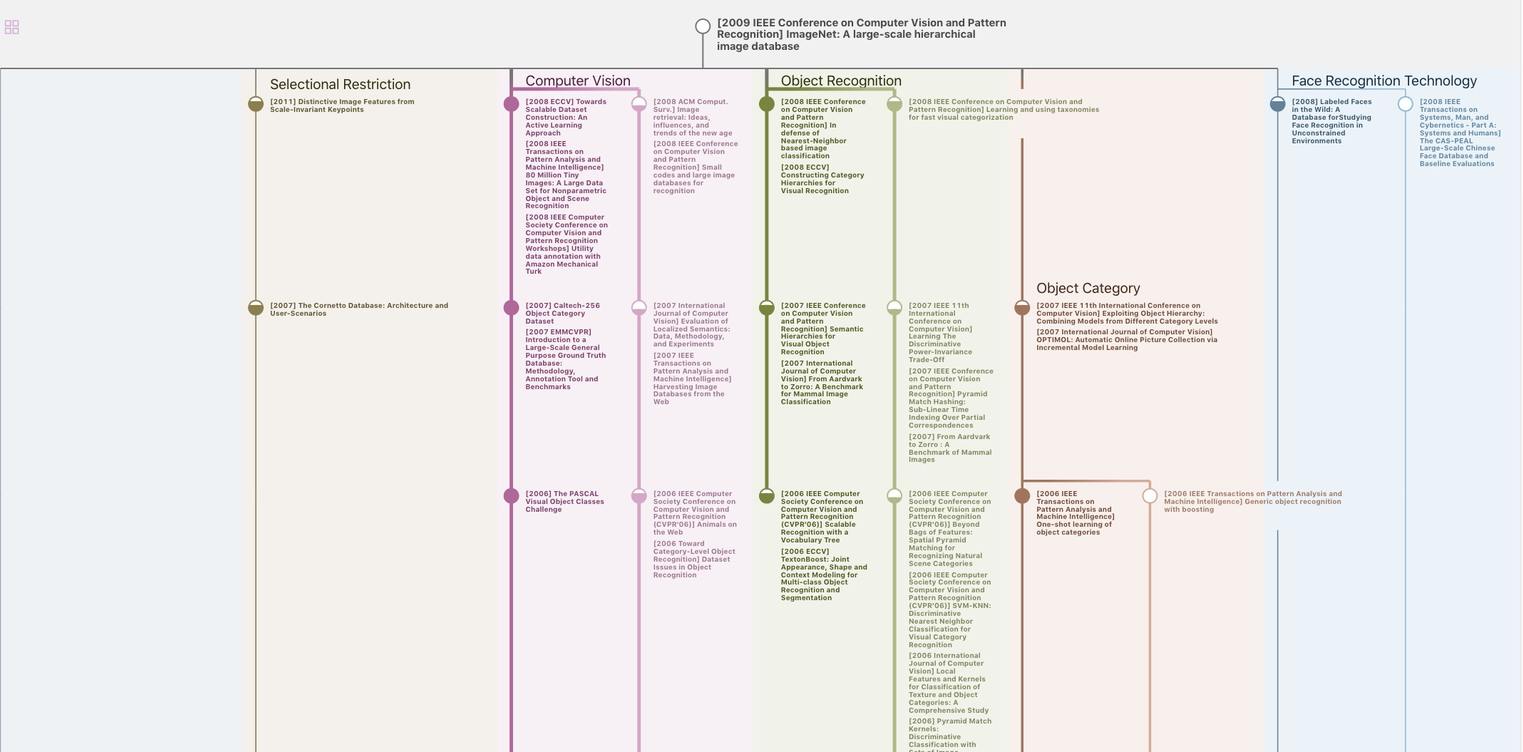
生成溯源树,研究论文发展脉络
Chat Paper
正在生成论文摘要