An Approach for Inferring Causal Directions from Multi-Dimensional Networks
2017 IEEE International Conference on Computational Science and Engineering (CSE) and IEEE International Conference on Embedded and Ubiquitous Computing (EUC)(2017)
摘要
Inferring causal directions from observed variables is one of the fundamental problems in many scientific fields. In this paper, a new approach for causal-direction inference from multi-dimensional networks is proposed based on a split-and-merge strategy. The method first decomposes an n-dimensional network into induced subnetworks, each of which corresponds to a node in the network. It shows that each induced subnetwork can be subsumed to one of the three substructures: one-degree, non-triangle and triangle-existence substructures. Three effective algorithms are developed to infer causalities from the three substructures. The whole causal structure of the multi-dimensional network is obtained by learning these induced subnetworks separately. Experimental results demonstrate that our method is more general and effective than the state-of-the-art methods.
更多查看译文
关键词
Inferring,Causal Directions,Multi-dimensional Networks,Split-and-merge,nduced Subnetworks
AI 理解论文
溯源树
样例
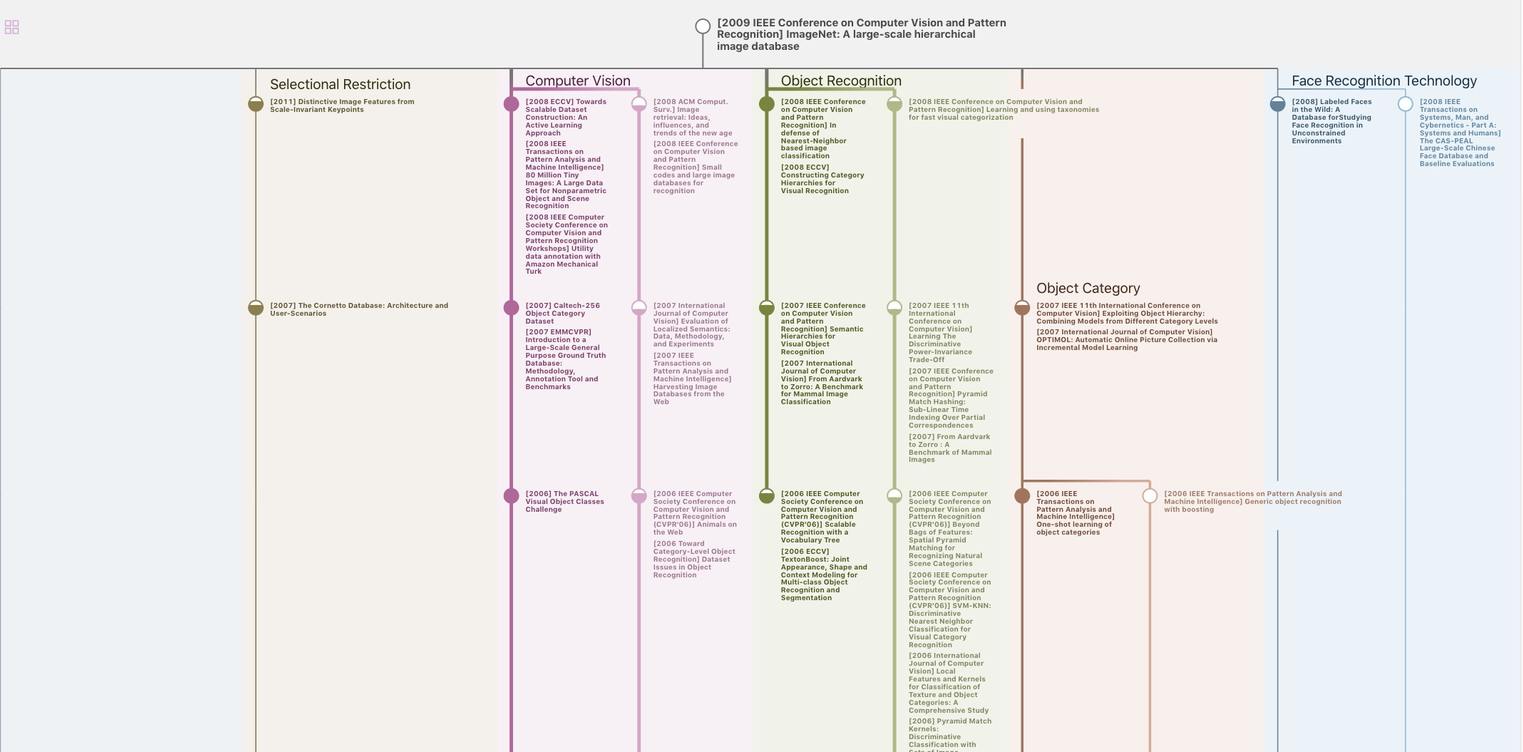
生成溯源树,研究论文发展脉络
Chat Paper
正在生成论文摘要