Combining Node Identifier Features And Community Priors For Within-Network Classification
WEB AND BIG DATA, APWEB-WAIM 2017, PT II(2017)
摘要
With widely available large-scale network data, one hot topic is how to adopt traditional classification algorithms to predict the most probable labels of nodes in a partially labeled network. In this paper, we propose a new algorithm called identifier based relational neighbor classifier (IDRN) to solve the within-network multi-label classification problem. We use the node identifiers in the egocentric networks as features and propose a within-network classification model by incorporating community structure information to predict the most probable classes for unlabeled nodes. We demonstrate the effectiveness of our approach on several publicly available datasets. On average, our approach can provide Hamming score, Micro-F-1 score and Macro-F-1 score up to 14%, 21% and 14% higher than competing methods respectively in sparsely labeled networks. The experiment results show that our approach is quite efficient and suitable for large-scale real-world classification tasks.
更多查看译文
关键词
Within-network classification, Node classification, Collective classification, Relational learning
AI 理解论文
溯源树
样例
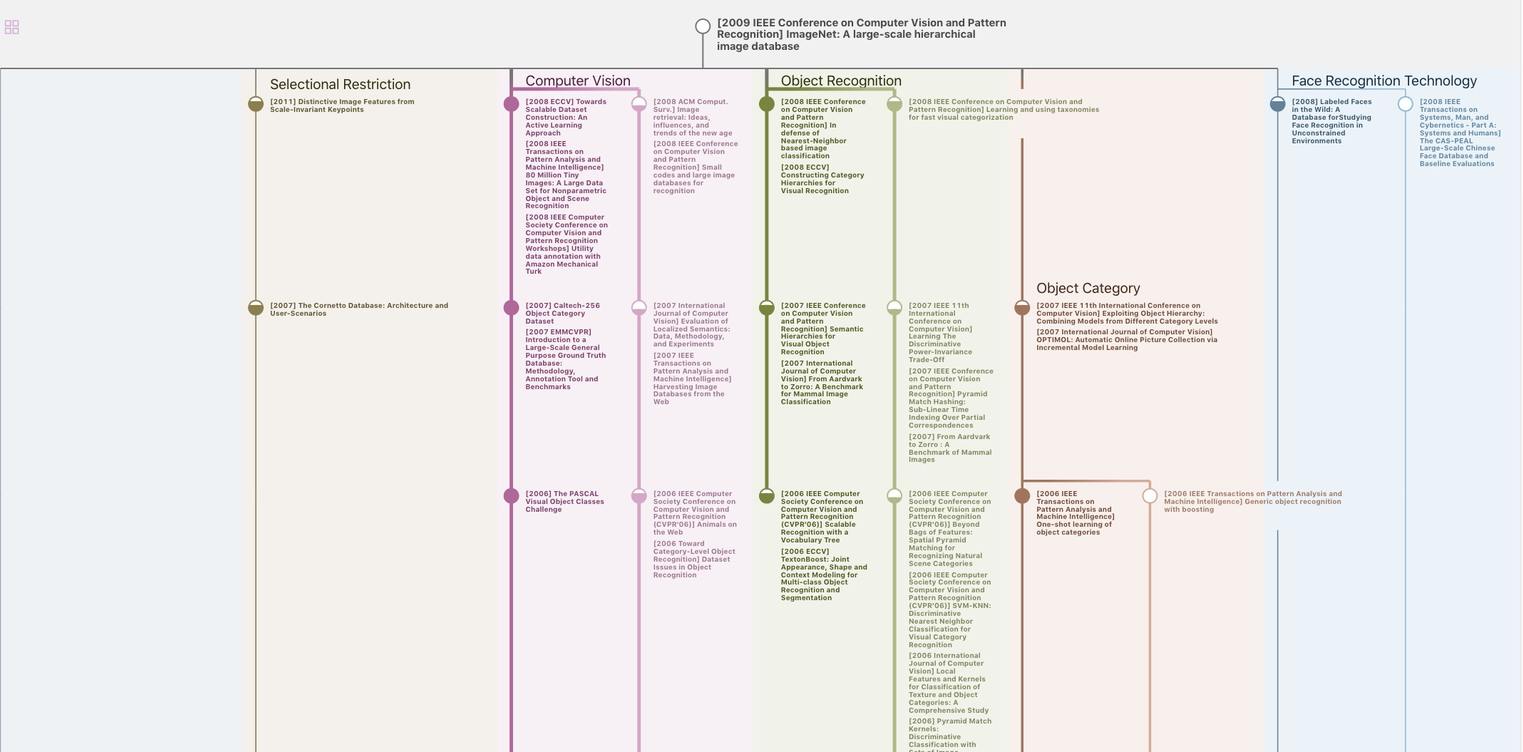
生成溯源树,研究论文发展脉络
Chat Paper
正在生成论文摘要