Feature Analysis and Selection for Training an End-to-End Autonomous Vehicle Controller Using the Deep Learning Approach.
2017 28TH IEEE INTELLIGENT VEHICLES SYMPOSIUM (IV 2017)(2017)
摘要
Deep learning-based approaches have been widely used for training controllers for autonomous vehicles due to their powerful ability to approximate nonlinear functions or policies. However, the training process usually requires large labeled data sets and takes a lot of time. In this paper, we analyze the influences of features on the performance of controllers trained using the convolutional neural networks (CNNs), which gives a guideline of feature selection to reduce computation cost. We collect a large set of data using The Open Racing Car Simulator (TORCS) and classify the image features into three categories (sky-related, roadside-related, and road-related features). We then design two experimental frameworks to investigate the importance of each single feature for training a CNN controller. The first framework uses the training data with all three features included to train a controller, which is then tested with data that has one feature removed to evaluate the feature's effects. The second framework is trained with the data that has one feature excluded, while all three features are included in the test data. Different driving scenarios are selected to test and analyze the trained controllers using the two experimental frameworks. The experiment results show that (1) the road-related features are indispensable for training the controller, (2) the roadside-related features are useful to improve the generalizability of the controller to scenarios with complicated roadside information, and (3) the sky-related features have limited contribution to train an end-to-end autonomous vehicle controller.
更多查看译文
关键词
feature analysis,feature selection,end-to-end autonomous vehicle controller,deep learning,autonomous vehicles,nonlinear functions,convolutional neural networks,CNNs,computation cost reduction,the open racing car simulator,TORCS,image feature classification,CNN controller training
AI 理解论文
溯源树
样例
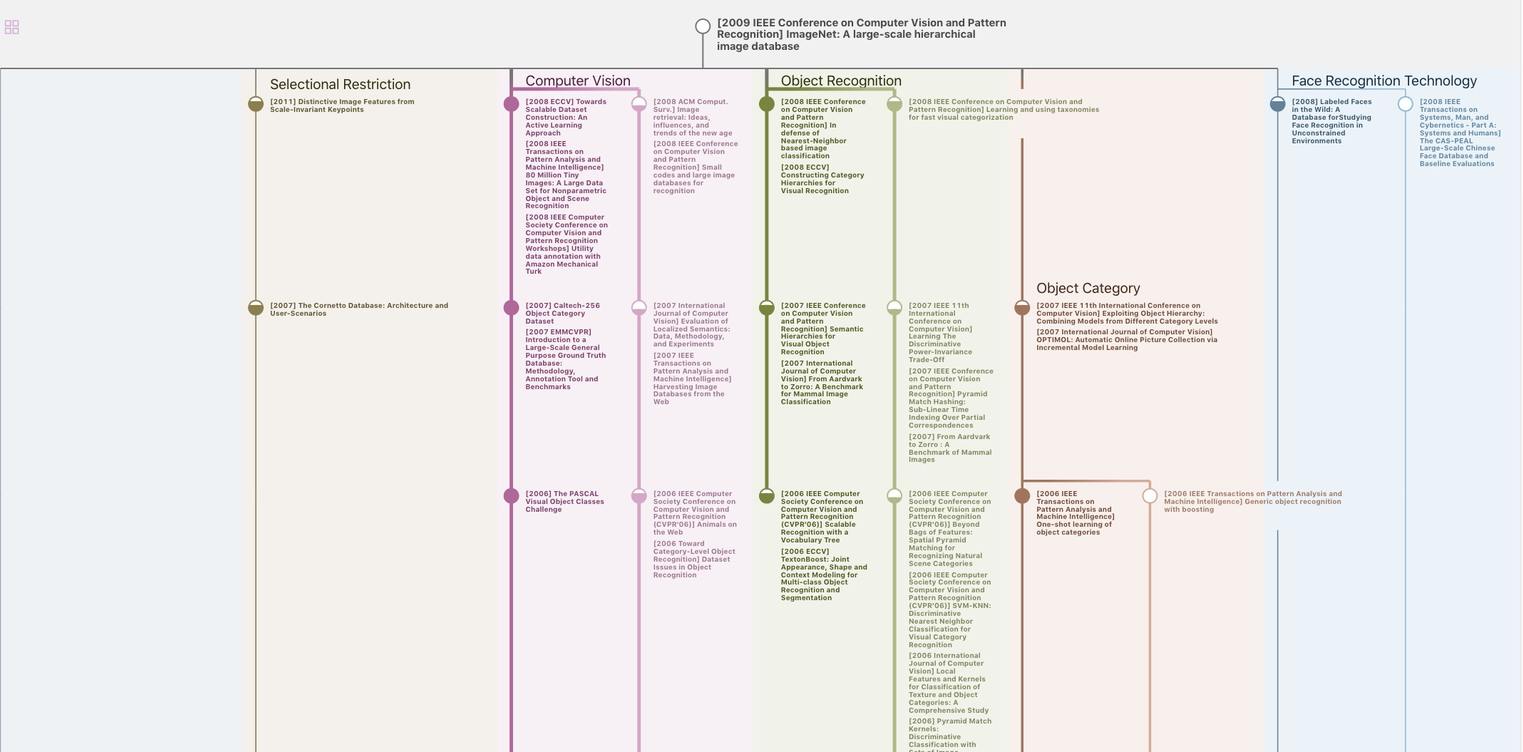
生成溯源树,研究论文发展脉络
Chat Paper
正在生成论文摘要