Motion-Coherent Affinities For Hypergraph Based Motion Segmentation
COMPUTER ANALYSIS OF IMAGES AND PATTERNS(2017)
摘要
Motion segmentation is the task of classifying the feature trajectories in an image sequence to different motions. Hypergraph based approaches use a specific graph to incorporate higher order similarities for the estimation of motion clusters. They follow the concept of hypothesis generation and validation. For the sampling of hypotheses, a high probability of selecting clean samples, i.e. samples consisting of points from the same cluster, is desired. Many approaches use spatial proximity to build an auxiliary graph for the sampling. But, spatial proximity is often not sufficient to capture the main affinities for motion segmentation. Thus, we introduce a simple but effective model for incorporating motion-coherent affinities into the auxiliary graph. The evaluation on two state of the art benchmarks shows that the hypotheses generated from the resulting hypergraphs lead to a significant decrease of the segmentation error. Additionally, less computation time is required due to a reduced hypergraph complexity.
更多查看译文
AI 理解论文
溯源树
样例
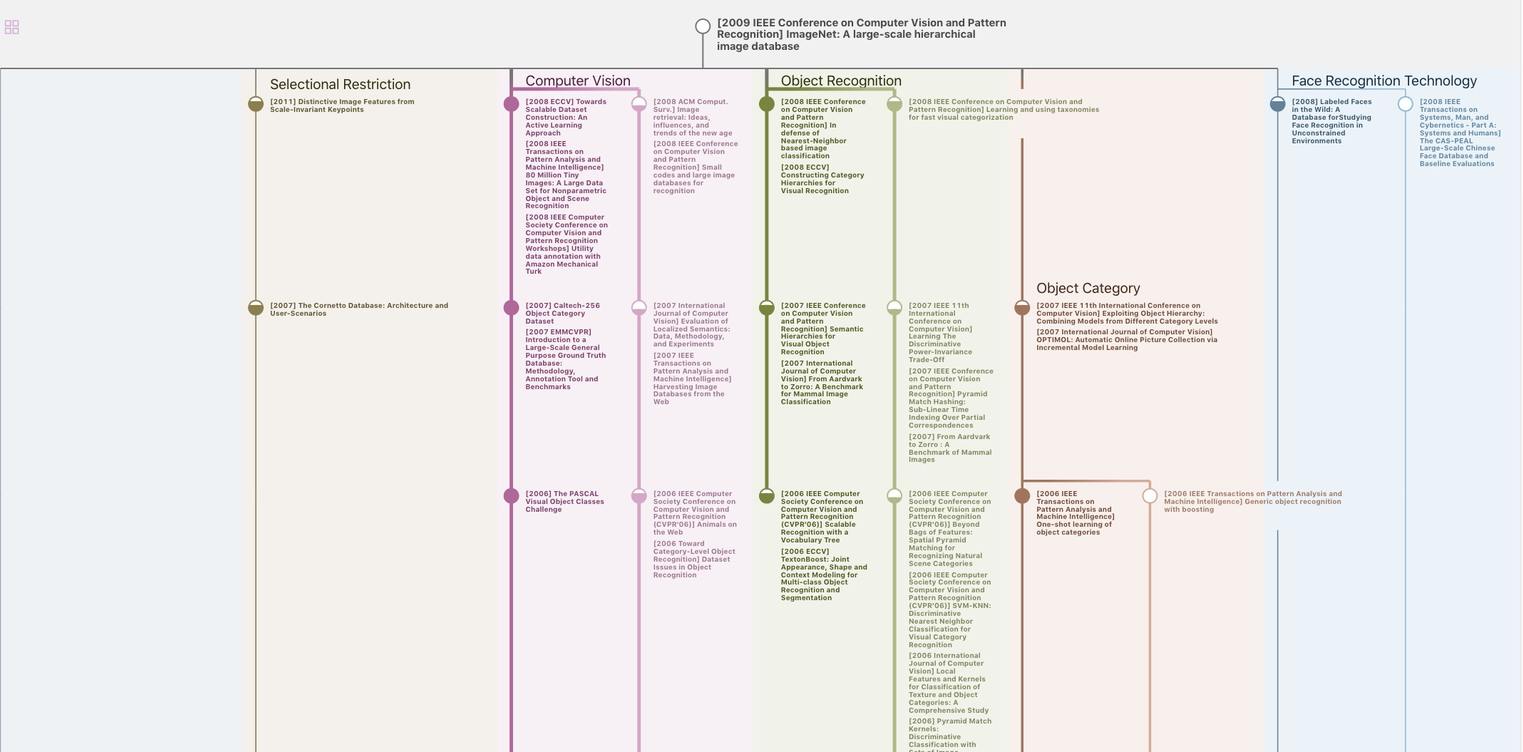
生成溯源树,研究论文发展脉络
Chat Paper
正在生成论文摘要