Twin SVM-Based Classification of Alzheimer's Disease Using Complex Dual-Tree Wavelet Principal Coefficients and LDA.
JOURNAL OF HEALTHCARE ENGINEERING(2017)
摘要
Alzheimer's disease (AD) is a leading cause of dementia, which causes serious health and socioeconomic problems. A progressive neurodegenerative disorder, Alzheimer's causes the structural change in the brain, thereby affecting behavior, cognition, emotions, and memory. Numerous multivariate analysis algorithms have been used for classifying AD, distinguishing it from healthy controls (HC). Efficient early classification of AD and mild cognitive impairment (MCI) from HC is imperative as early preventive care could help to mitigate risk factors. Magnetic resonance imaging (MRI), a noninvasive biomarker, displays morphometric differences and cerebral structural changes. A novel approach for distinguishing AD from HC using dual-tree complex wavelet transforms (DTCWT), principal coefficients from the transaxial slices of MRI images, linear discriminant analysis, and twin support vector machine is proposed here. The prediction accuracy of the proposed method yielded up to 92.65 +/- 1.18 over the Alzheimer's Disease Neuroimaging Initiative (ADNI) dataset, with a specificity of 92.19 +/- 1.56 and sensitivity of 93.11 +/- 1.29, and 96.68 +/- 1.44 over the Open Access Series of Imaging Studies (OASIS) dataset, with a sensitivity of 97.72 +/- 2.34 and specificity of 95.61 +/- 1.67. The accuracy, sensitivity, and specificity achieved using the proposed method are comparable or superior to those obtained by various conventional AD prediction methods.
更多查看译文
AI 理解论文
溯源树
样例
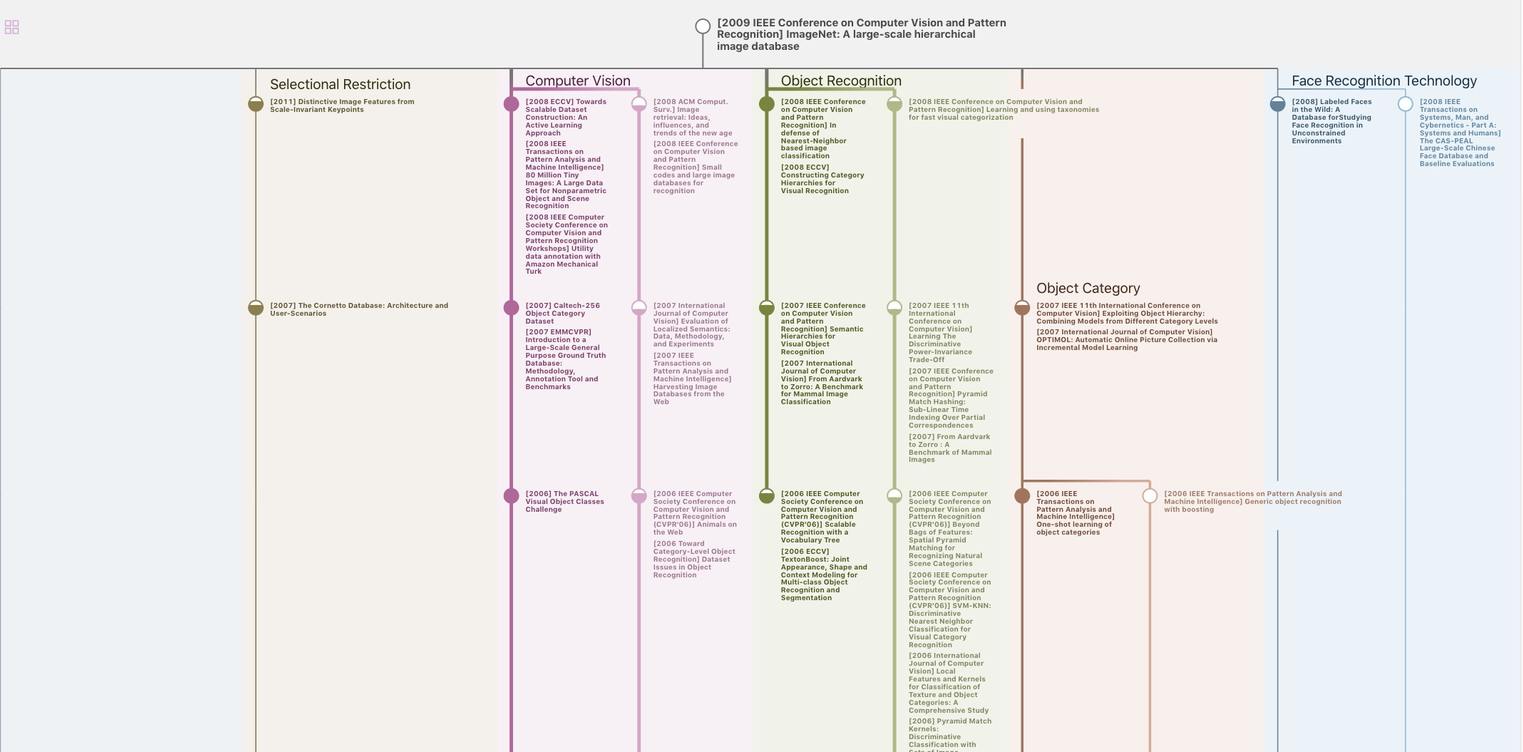
生成溯源树,研究论文发展脉络
Chat Paper
正在生成论文摘要