Joint Multi-Person Pose Estimation and Semantic Part Segmentation
30TH IEEE CONFERENCE ON COMPUTER VISION AND PATTERN RECOGNITION (CVPR 2017)(2017)
摘要
Human pose estimation and semantic part segmentation are two complementary tasks in computer vision. In this paper, we propose to solve the two tasks jointly for natural multi-person images, in which the estimated pose provides object-level shape prior to regularize part segments while the part-level segments constrain the variation of pose locations. Specifically, we first train two fully convolutional neural networks (FCNs), namely Pose FCN and Part FCN, to provide initial estimation of pose joint potential and semantic part potential. Then, to refine pose joint location, the two types of potentials are fused with a fully-connected conditional random field (FCRF), where a novel segment-joint smoothness term is used to encourage semantic and spatial consistency between parts and joints. To refine part segments, the refined pose and the original part potential are integrated through a Part FCN, where the skeleton feature from pose serves as additional regularization cues for part segments. Finally, to reduce the complexity of the FCRF, we induce human detection boxes and infer the graph inside each box, making the inference forty times faster. Since there's no dataset that contains both part segments and pose labels, we extend the PASCAL VOC part dataset with human pose joints and perform extensive experiments to compare our method against several most recent strategies. We show that on this dataset our algorithm surpasses competing methods by a large margin in both tasks.
更多查看译文
关键词
human pose estimation,segment-joint smoothness,fully convolutional neural networks,pose joint potential,fully-connected conditional random field,joint location,semantic part potential,Pose FCN,pose locations,part-level segments,natural multiperson images,joint multiperson,semantic part segmentation,pose estimation,human pose joints,PASCAL VOC part dataset,Part FCN
AI 理解论文
溯源树
样例
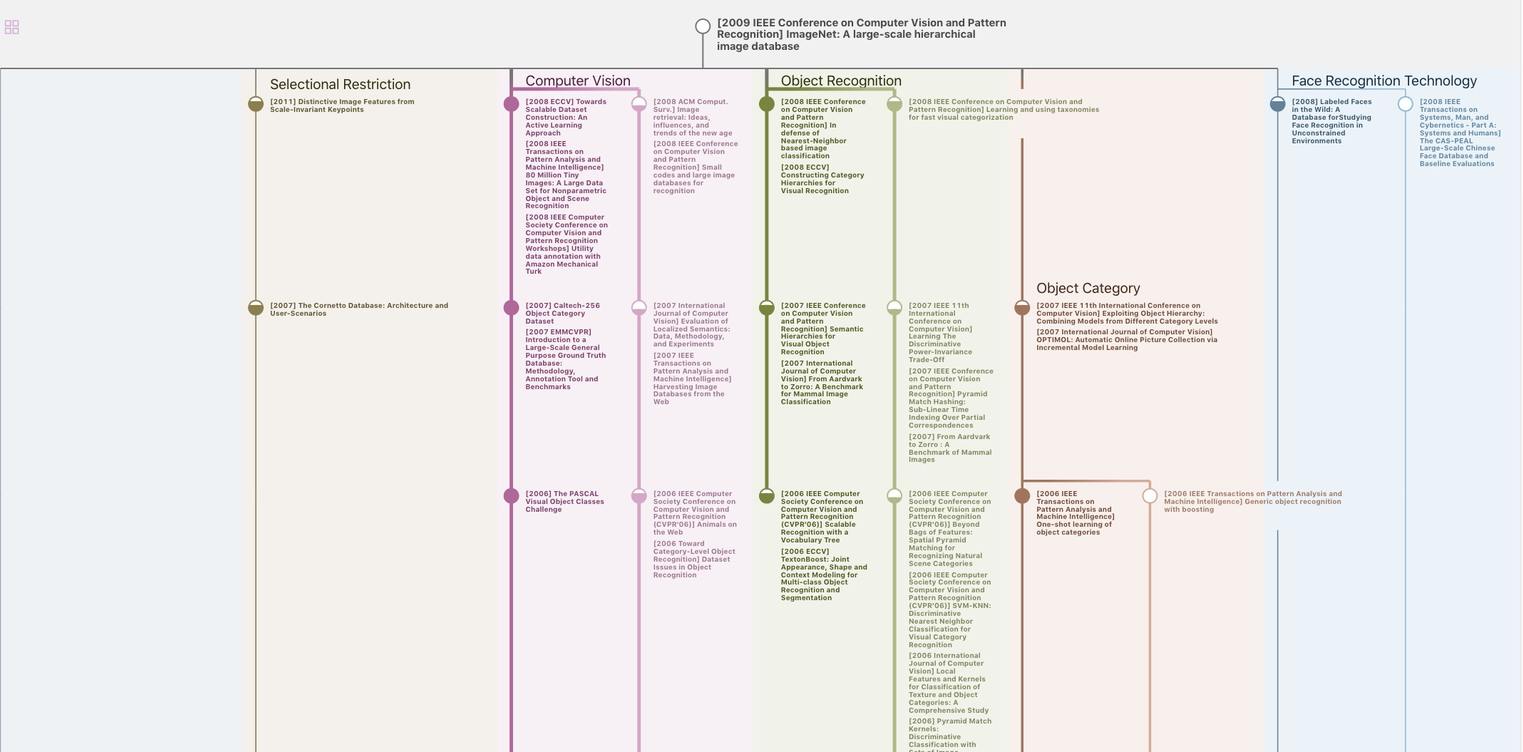
生成溯源树,研究论文发展脉络
Chat Paper
正在生成论文摘要