RGB-D Scene Labeling with Multimodal Recurrent Neural Networks
2017 IEEE Conference on Computer Vision and Pattern Recognition Workshops (CVPRW)(2017)
摘要
Recurrent neural networks (RNNs) are able to capture context in an image by modeling long-range semantic dependencies among image units. However, existing methods only utilize RNNs to model dependencies of a single modality (e.g., RGB) for labeling. In this work we extend this single-modal RNNs to multimodal RNNs (MM-RNNs) and apply it to RGB-D scene labeling. Our MM-RNNs are capable of seamlessly modeling dependencies of both RGB and depth modalities, and allow 'memory' sharing across modalities. By sharing 'memory', each modality possesses multiple properties of itself and other modalities, and becomes more discriminative to distinguish pixels. Moreover, we also analyse two simple extensions of single-modal RNNs and demonstrate that our MM-RNNs perform better than both of them. Integrating with convolutional neural networks (CNNs), we build an end-to-end network for RGB-D scene labeling. Extensive experiments on NYU depth V1 and V2 demonstrate the effectiveness of MM-RNNs.
更多查看译文
关键词
RGB-D scene labeling,multimodal recurrent neural networks,multimodal RNN,single-modal RNN,image units,MM-RNN,depth modalities,RGB modalities,convolutional neural networks,CNN,NYU depth V1,NYU depth V2
AI 理解论文
溯源树
样例
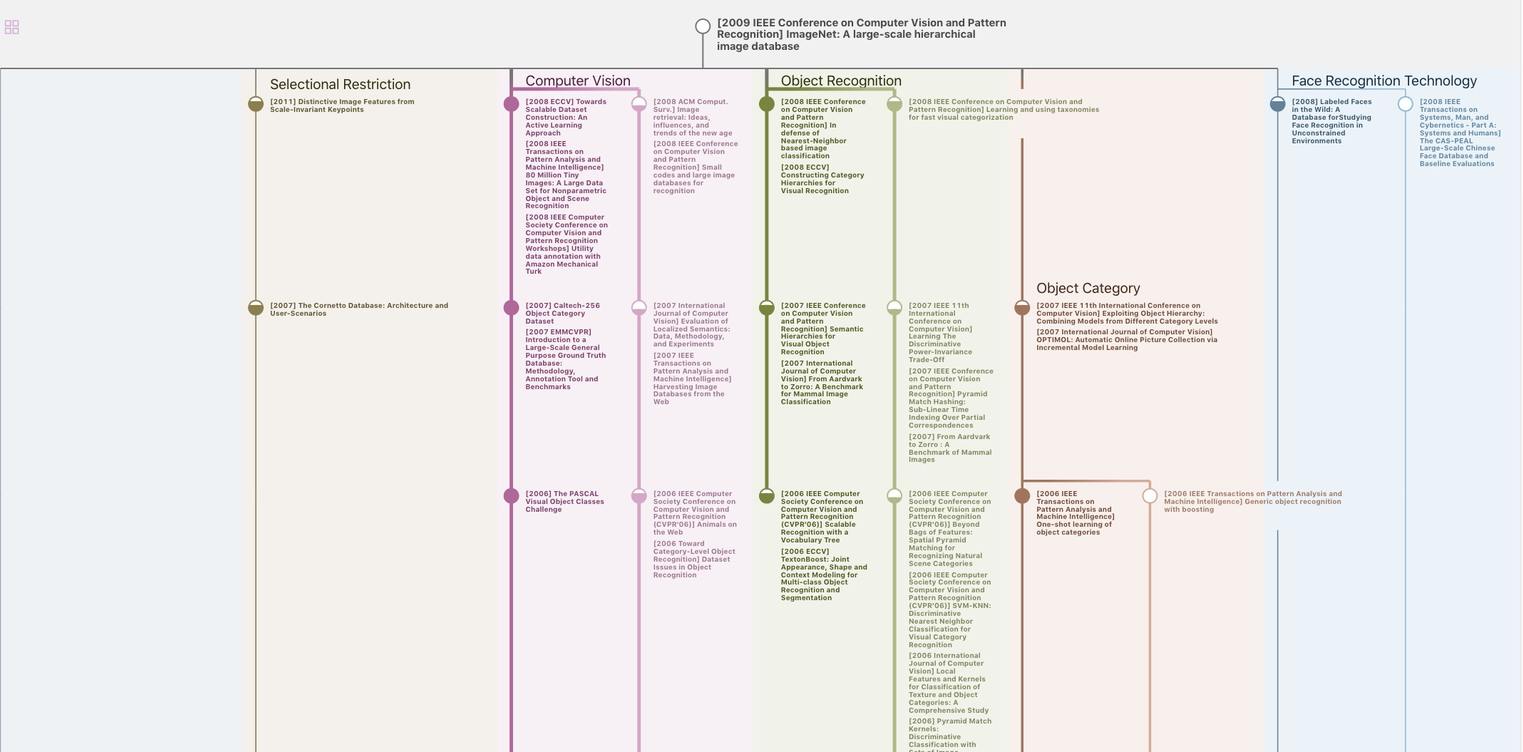
生成溯源树,研究论文发展脉络
Chat Paper
正在生成论文摘要