Robust Hand Detection and Classification in Vehicles and in the Wild.
IEEE Computer Society Conference on Computer Vision and Pattern Recognition Workshops(2017)
摘要
Robust hand detection and classification is one of the most crucial pre-processing steps to support human computer interaction, driver behavior monitoring, virtual reality, etc. This problem, however, is very challenging due to numerous variations of hand images in real-world scenarios. This work presents a novel approach named Multiple Scale Region-based Fully Convolutional Networks (MS-RFCN) to robustly detect and classify human hand regions under various challenging conditions, e.g. occlusions, illumination, low-resolutions. In this approach, the whole image is passed through the proposed fully convolutional network to compute score maps. Those score maps with their position-sensitive properties can help to efficiently address a dilemma between translation-invariance in classification and detection. The method is evaluated on the challenging hand databases, i.e. the Vision for Intelligent Vehicles and Applications (VIVA) Challenge, Oxford hand dataset and compared against various recent hand detection methods. The experimental results show that our proposed MS-FRCN approach consistently achieves the state-of-the-art hand detection results, i.e. Average Precision (AP) / Average Recall (AR) of 95.1% / 94.5% at level 1 and 86.0% / 83.4% at level 2, on the VIVA challenge. In addition, the proposed method achieves the state-of-the-art results for left/right hand and driver/passenger classification tasks on the VIVA database with a significant improvement on AP/AR of 7% and 13% for both classification tasks, respectively. The hand detection performance of MS-RFCN reaches to 75.1% of AP and 77.8% of AR on Oxford database.
更多查看译文
关键词
robust human hand region detection,human hand region classification,multiple scale region-based fully convolutional networks,MSRFCN,fully convolutional network,score maps,position-sensitive properties,translation-invariance dilemma,vision for intelligent vehicles and applications,VIVA,MS-FRCN approach,average precision,average recall,AP,AR,driver/passenger classification tasks,left/right hand classification tasks,Oxford database
AI 理解论文
溯源树
样例
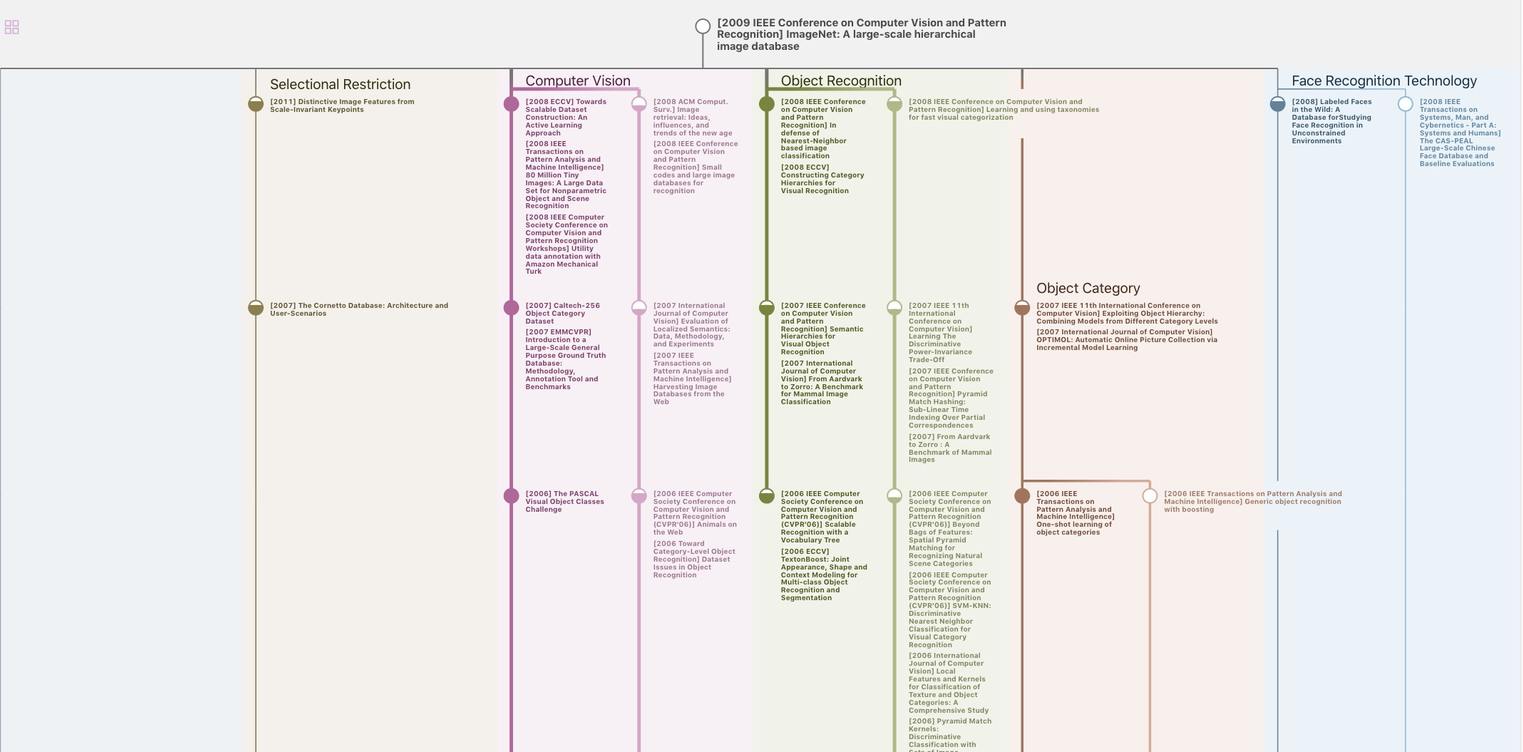
生成溯源树,研究论文发展脉络
Chat Paper
正在生成论文摘要