Real-Time Neural Style Transfer For Videos
30TH IEEE CONFERENCE ON COMPUTER VISION AND PATTERN RECOGNITION (CVPR 2017)(2017)
摘要
Recent research endeavors have shown the potential of using feed-forward convolutional neural networks to accomplish fast style transfer for images. In this work, we take one step further to explore the possibility of exploiting a feed-forward network to perform style transfer for videos and simultaneously maintain temporal consistency among stylized video frames. Our feed-forward network is trained by enforcing the outputs of consecutive frames to be both well stylized and temporally consistent. More specifically, a hybrid loss is proposed to capitalize on the content information of input frames, the style information of a given style image, and the temporal information of consecutive frames. To calculate the temporal loss during the training stage, a novel two-frame synergic training mechanism is proposed. Compared with directly applying an existing image style transfer method to videos, our proposed method employs the trained network to yield temporally consistent stylized videos which are much more visually pleasant. In contrast to the prior video style transfer method which relies on time-consuming optimization on the fly, our method runs in real time while generating competitive visual results.
更多查看译文
关键词
input frames,style information,temporal information,temporal loss,training stage,two-frame synergic training mechanism,trained network,temporally consistent stylized videos,feed-forward convolutional neural networks,fast style transfer,feed-forward network,temporal consistency,stylized video frames,hybrid loss,content information,image style,real-time neural style transfer,video style transfer method
AI 理解论文
溯源树
样例
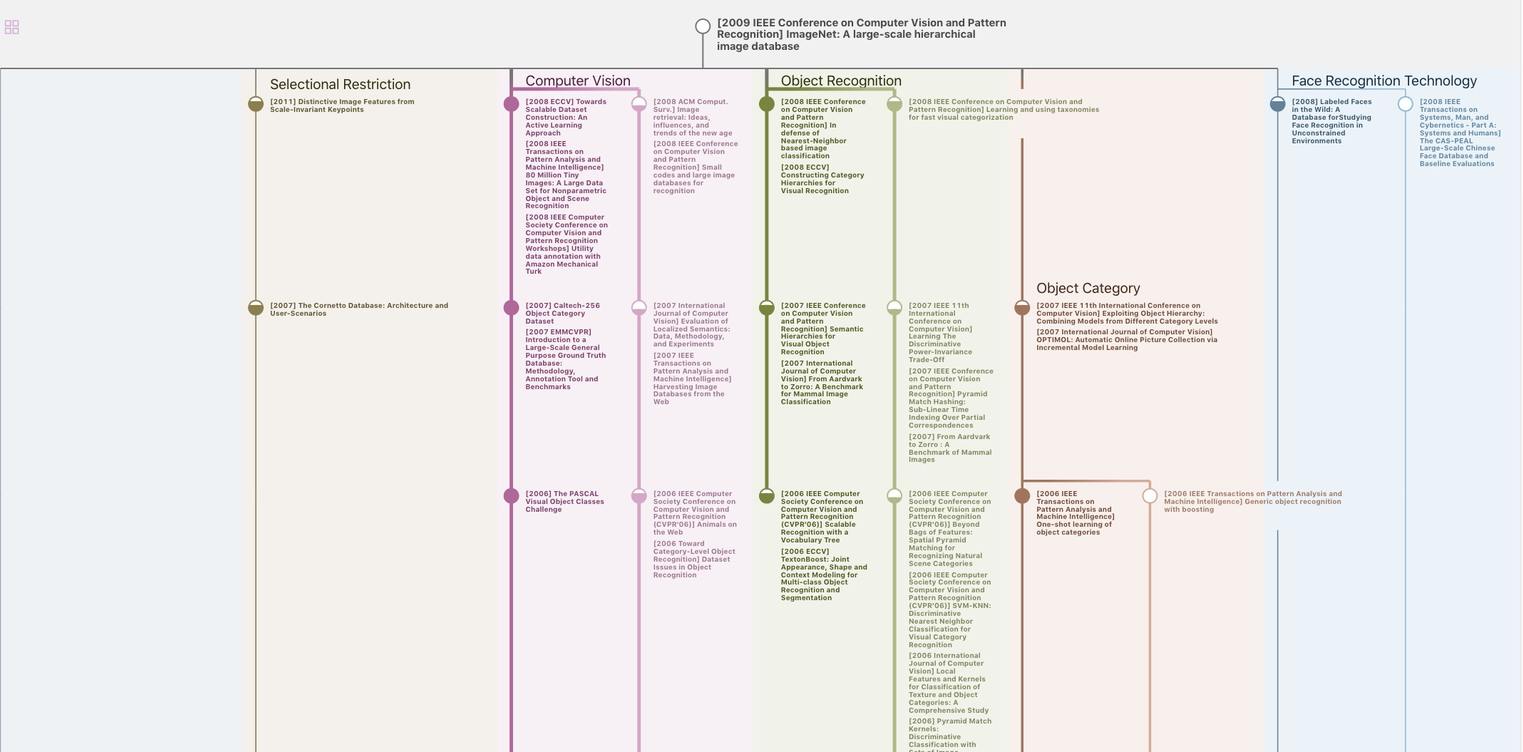
生成溯源树,研究论文发展脉络
Chat Paper
正在生成论文摘要