Factorized Variational Autoencoders for Modeling Audience Reactions to Movies
30TH IEEE CONFERENCE ON COMPUTER VISION AND PATTERN RECOGNITION (CVPR 2017)(2017)
摘要
Matrix and tensor factorization methods are often used for finding underlying low-dimensional patterns from noisy data. In this paper, we study non-linear tensor factorization methods based on deep variational autoencoders. Our approach is well-suited for settings where the relationship between the latent representation to be learned and the raw data representation is highly complex. We apply our approach to a large dataset of facial expressions of movie-watching audiences (over 16 million faces). Our experiments show that compared to conventional linear factorization methods, our method achieves better reconstruction of the data, and further discovers interpretable latent factors.
更多查看译文
关键词
movie-watching,linear factorization,audience reaction modeling,movies,factorized variational autoencoders,raw data representation,deep variational autoencoders
AI 理解论文
溯源树
样例
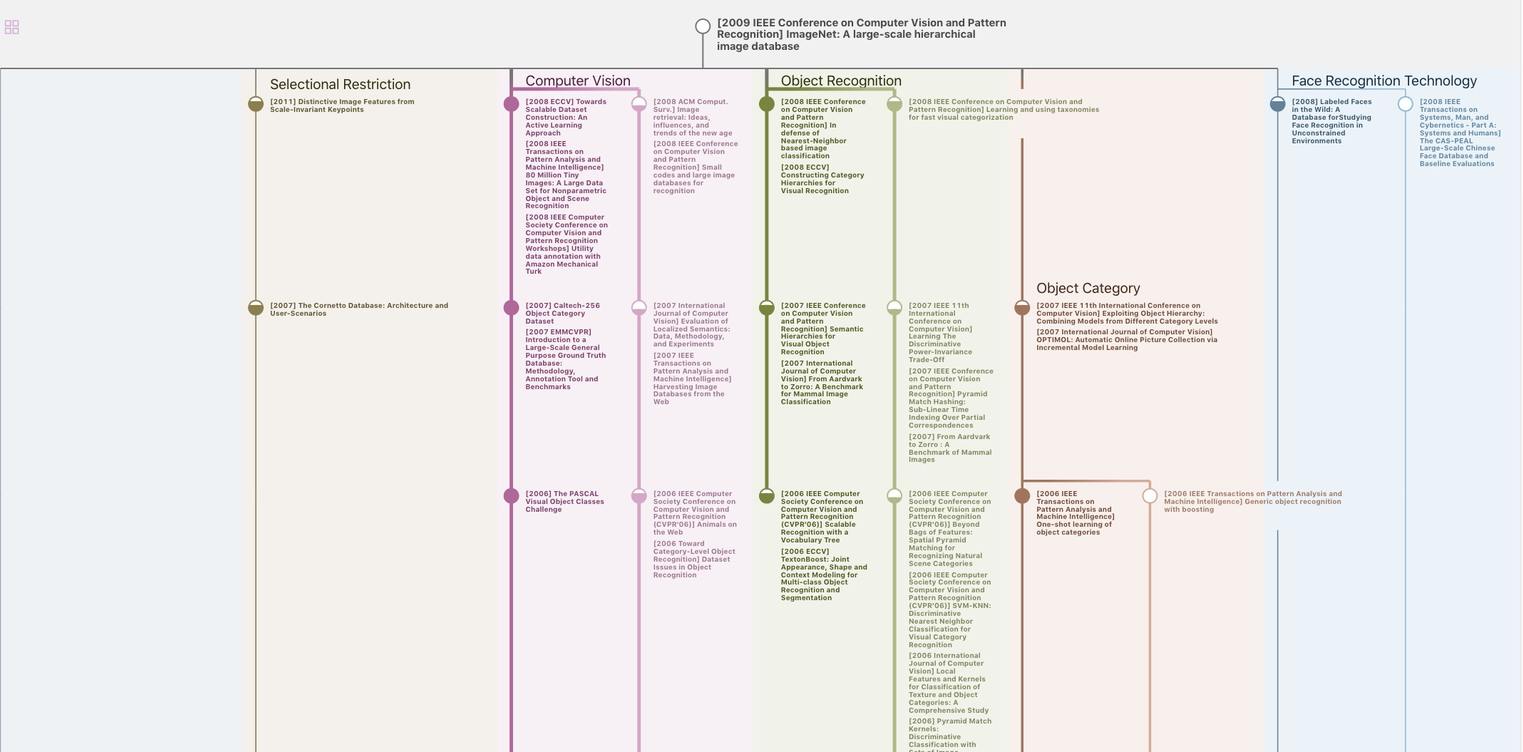
生成溯源树,研究论文发展脉络
Chat Paper
正在生成论文摘要