Generative Hierarchical Learning Of Sparse Frame Models
30TH IEEE CONFERENCE ON COMPUTER VISION AND PATTERN RECOGNITION (CVPR 2017)(2017)
摘要
This paper proposes a method for generative learning of hierarchical random field models. The resulting model, which we call the hierarchical sparse FRAME (Filters, Random field, And Maximum Entropy) model, is a generalization of the original sparse FRAME model by decomposing it into multiple parts that are allowed to shift their locations, scales and rotations, so that the resulting model becomes a hierarchical deformable template. The model can be trained by an EM-type algorithm that alternates the following two steps: (1) Inference: Given the current model, we match it to each training image by inferring the unknown locations, scales, and rotations of the object and its parts by recursive sum-max maps, and (2) Re-learning: Given the inferred geometric configurations of the objects and their parts, we re-learn the model parameters by maximum likelihood estimation via stochastic gradient algorithm. Experiments show that the proposed method is capable of learning meaningful and interpretable templates that can be used for object detection, classification and clustering.
更多查看译文
关键词
maximum entropy model,inference,EM-type algorithm,maximum likelihood estimation,stochastic gradient algorithm,interpretable templates,learning meaningful templates,inferred geometric configurations,hierarchical deformable template,hierarchical sparse FRAME,hierarchical random field models,sparse FRAME models,generative hierarchical learning
AI 理解论文
溯源树
样例
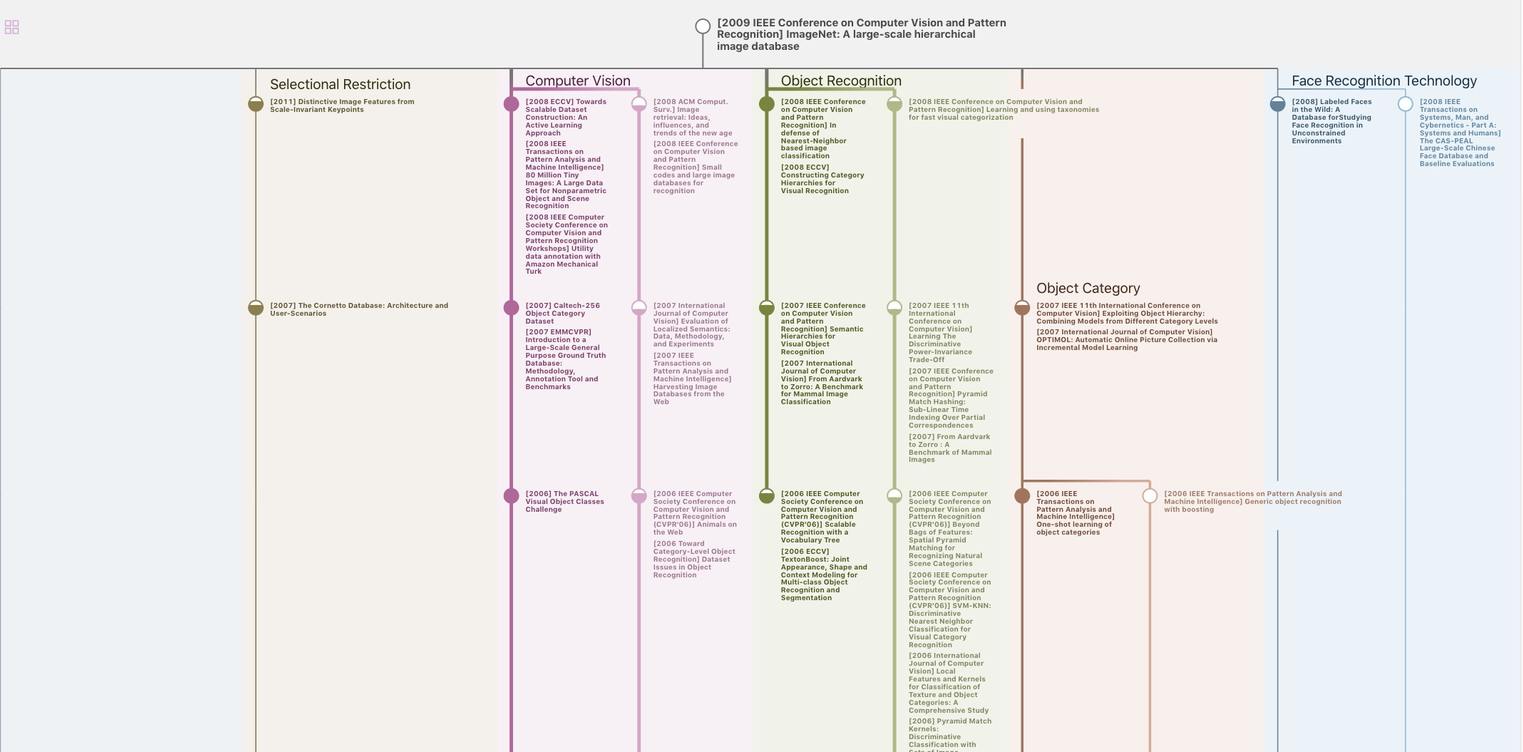
生成溯源树,研究论文发展脉络
Chat Paper
正在生成论文摘要