Edge datastore for distributed vision analytics: poster.
SEC(2017)
摘要
Autonomous machine vision is a powerful tool to address challenges in multiple domains including national security (for example, video surveillance), health care (for example, patient monitoring), and transportation (for example, autonomous vehicles). Distributed vision, where multiple cameras observe a specific geographic area 24/7, enables smart understanding of events in a physical environment with minimal human intervention. We observe that the cloud paradigm alone does not offer a pathway to real-time distributed vision processing. With potentially thousands of cameras, hundreds of gigabytes data per second needs to be transferred to the cloud, saturating the bandwidth of the network. More importantly, vision applications are inherently latency-critical with a high demand for real-time scene analysis (for example, feature extraction and object tracking). To meet latency requirements, computation - including both processing of raw video streams to identify objects, and analytics on this data, needs to be brought to the edge of the network. While object recognition may be done locally at the end node (next to the camera), vision analytics requires access to data generated across different nodes. For example, a subject of interest may need to be tracked across multiple cameras to identify the nature of activities. This creates a need for a low latency distributed data store communicating over a dynamic communication network (most often wireless), to be implemented at the edge. Moreover, the data store must be able to address the limited storage at the end nodes (typically gigabytes). Additionally, privacy and security are prime concerns in the design of such a distributed edge storage.
更多查看译文
AI 理解论文
溯源树
样例
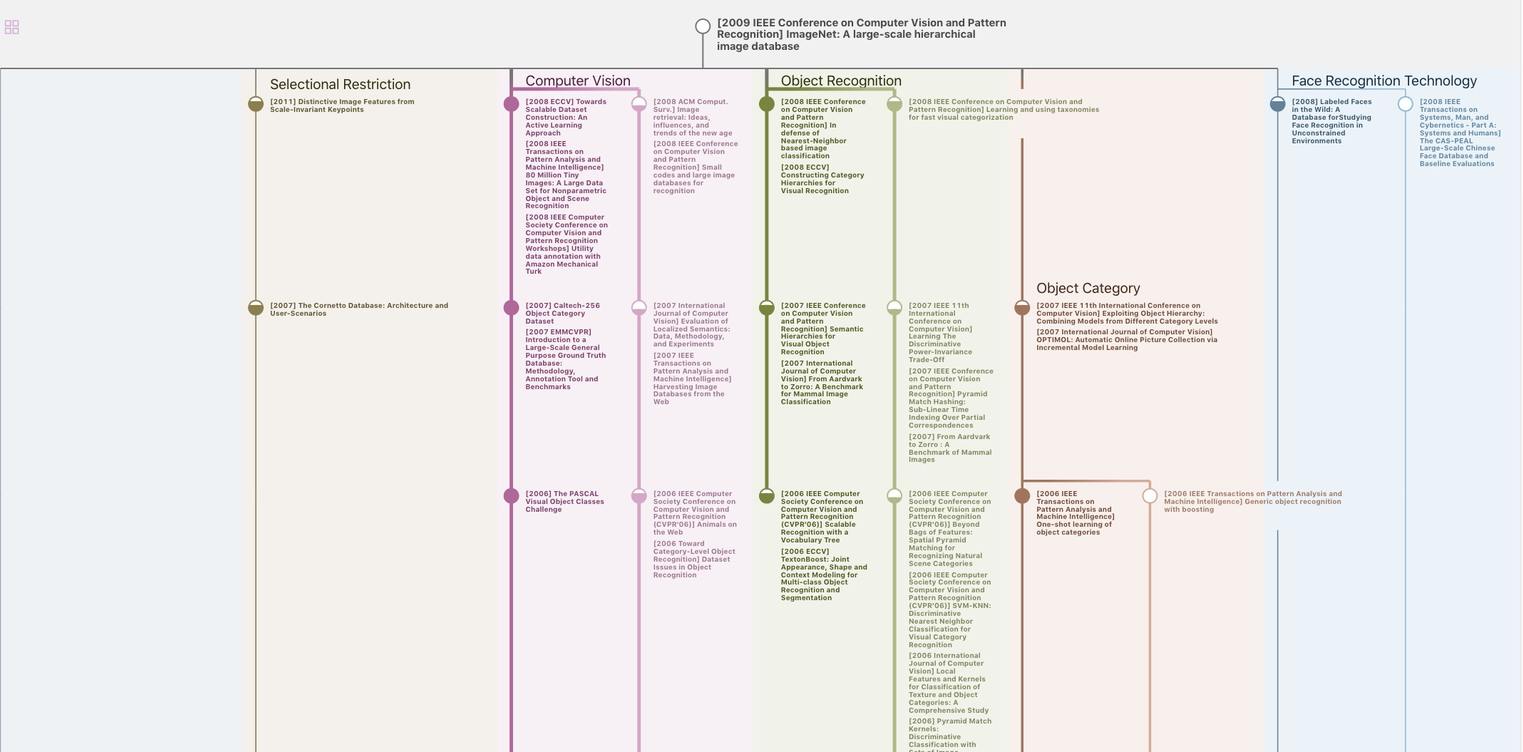
生成溯源树,研究论文发展脉络
Chat Paper
正在生成论文摘要