Geometric proposals for faster R-CNN
2017 14th IEEE International Conference on Advanced Video and Signal Based Surveillance (AVSS)(2017)
摘要
Faster R-CNN has established itself as the de-facto best object detector but it remains strongly limited in two aspects: (i) it is sensitive to background clutter and its classification performance decreases when it is confronted with more noisy proposals; (ii) it suffers when the objects vary largely in scale and specifically for the small objects. We address both issues with our geometric-proposals for Faster R-CNN (GP-FRCNN), whereby we re-rank the generic object proposals with an approximate geometric estimate of the scene. But the devil is in the details: the simple extension requires involved scale adjustments (e.g. anchors, layer resolution) which we detail in this paper. Finally, our GP-FRCNN performs equally well on smaller and larger objects, a long standing challenge for any object detection algorithm. The application of GP-FRCNN to surveillance videos is straightforward and does not require an explicit geometric formulation. We extensively test the model on the UA-DETRAC dataset, where GP-FRCNN outperforms the standard Faster R-CNN by 19%.
更多查看译文
关键词
geometric proposals,de-facto best object detector,background clutter,approximate geometric estimate,GP-FRCNN performs,object detection algorithm,faster R-CNN,object proposals ranking,surveillance videos
AI 理解论文
溯源树
样例
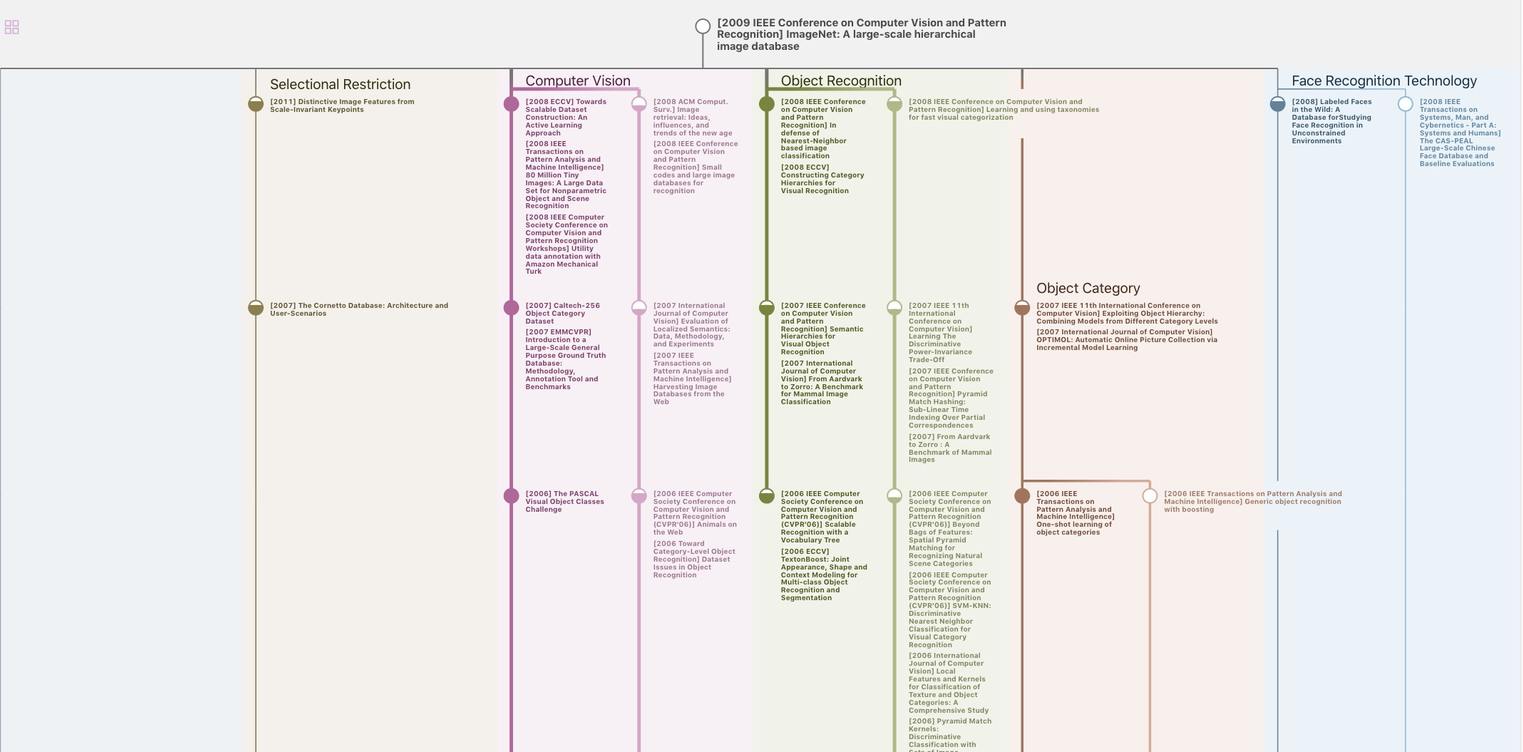
生成溯源树,研究论文发展脉络
Chat Paper
正在生成论文摘要