Semantic Monte-Carlo Localization In Changing Environments Using Rgb-D Cameras
2017 EUROPEAN CONFERENCE ON MOBILE ROBOTS (ECMR)(2017)
摘要
The localization with respect to a prior map is a fundamental requirement for mobile robots. The commonly used adaptive monte carlo localization (AMCL) can be found on most of the mobile robots ranging from small cleaning robots to large AGVs. While achieving accurate pose estimates in static environments, this algorithm tends to fail in the presence of significant changes. Recently published extensions and alternatives to AMCL observe the environment over longer times while building complex spatio-temporal models. Our approach, in contrast, utilizes object recognition and prior semantic maps to enable robust localization. It exploits the fact that putative changes in the environment can be predicted based on prior semantic knowledge. Our system is experimentally evaluated in a warehouse environment being subject to frequent changes. This emphasizes the importance of our algorithm for challenging industrial applications.
更多查看译文
关键词
robust localization,prior semantic knowledge,warehouse environment,changing environments,RGB-d cameras,mobile robots,AMCL,cleaning robots,static environments,complex spatio-temporal models,prior semantic maps,semantic Monte-carlo localization,adaptive Monte Carlo localization
AI 理解论文
溯源树
样例
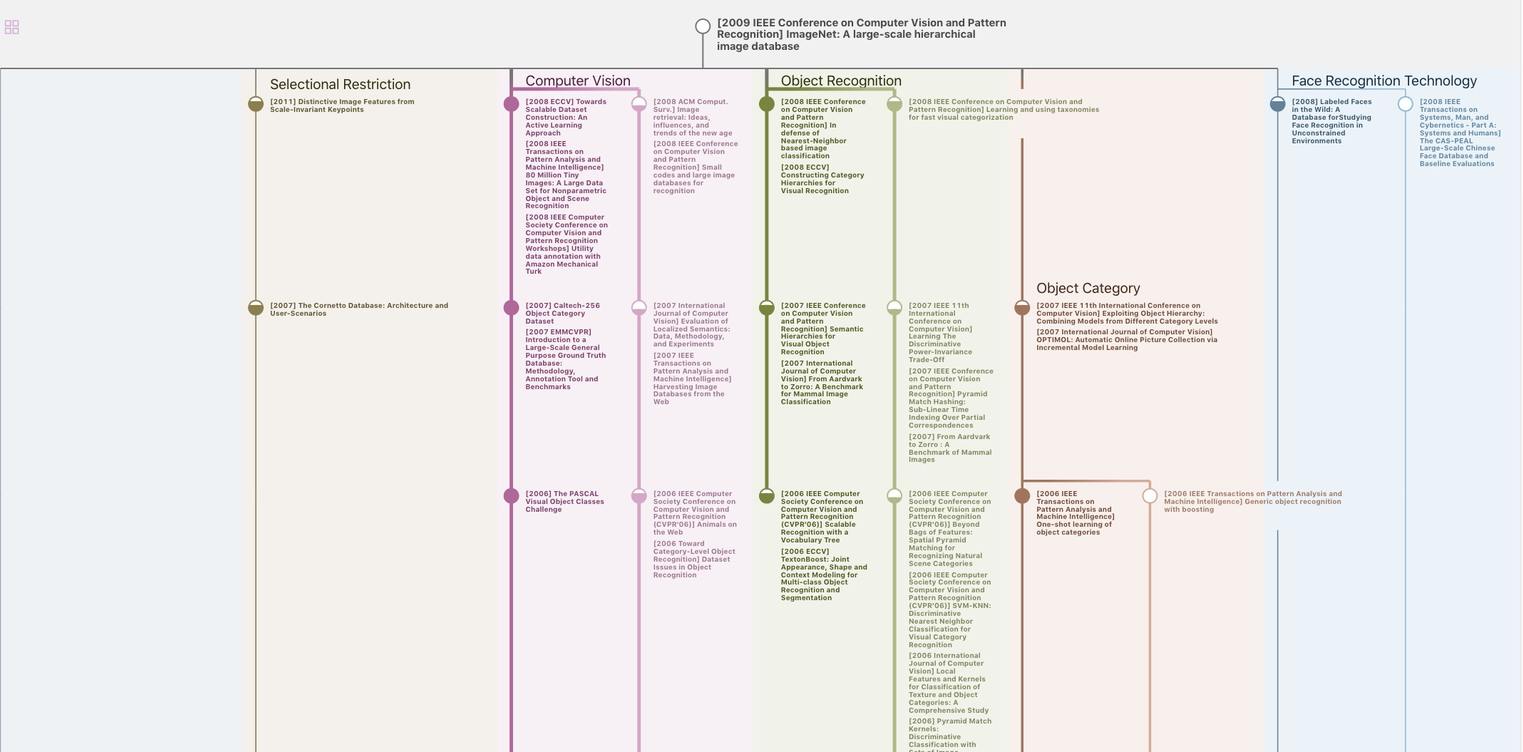
生成溯源树,研究论文发展脉络
Chat Paper
正在生成论文摘要