A Disentangled Recognition and Nonlinear Dynamics Model for Unsupervised Learning
neural information processing systems(2017)
摘要
This paper takes a step towards temporal reasoning in a dynamically changing video, not in the pixel space that constitutes its frames, but in a latent space that describes the non-linear dynamics of the objects in its world. We introduce the Kalman variational auto-encoder, a framework for unsupervised learning of sequential data that disentangles two latent representations: an object's representation, coming from a recognition model, and a latent state describing its dynamics. As a result, the evolution of the world can be imagined and missing data imputed, both without the need to generate high dimensional frames at each time step. The model is trained end-to-end on videos of a variety of simulated physical systems, and outperforms competing methods in generative and missing data imputation tasks.
更多查看译文
关键词
disentangled recognition,unsupervised learning,nonlinear dynamics model
AI 理解论文
溯源树
样例
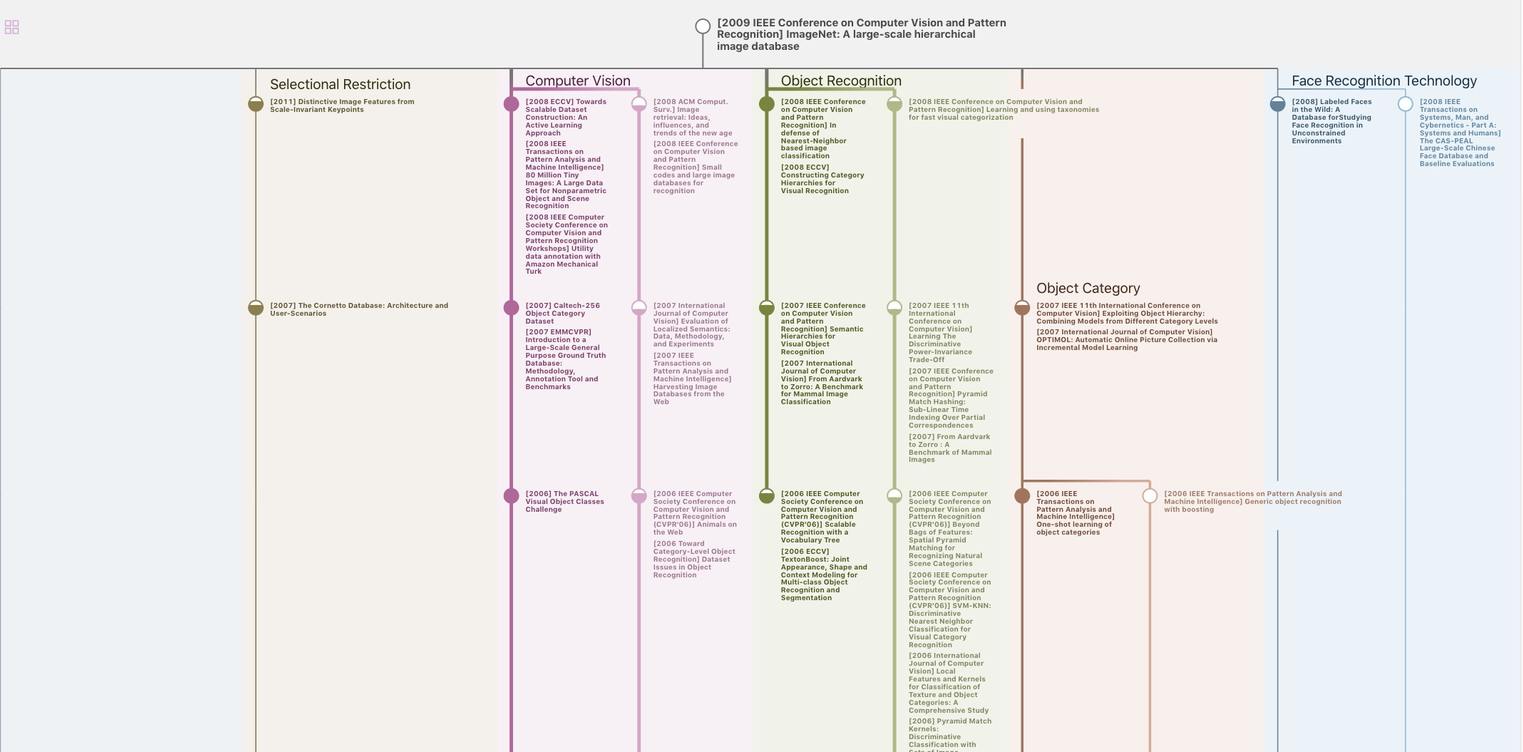
生成溯源树,研究论文发展脉络
Chat Paper
正在生成论文摘要