Empirical Evaluation Of Gradient Methods For Matrix Learning Vector Quantization
2017 12TH INTERNATIONAL WORKSHOP ON SELF-ORGANIZING MAPS AND LEARNING VECTOR QUANTIZATION, CLUSTERING AND DATA VISUALIZATION (WSOM)(2017)
摘要
Generalized Matrix Learning Vector Quantization (GMLVQ) critically relies on the use of an optimization algorithm to train its model parameters. We test various schemes for automated control of learning rates in gradient-based training. We evaluate these algorithms in terms of their achieved performance and their practical feasibility. We find that some algorithms do indeed perform better than others across multiple benchmark datasets. These algorithms produce GMLVQ models which not only better fit the training data, but also perform better upon validation. In particular, we find that the Variance-based Stochastic Gradient Descent algorithm consistently performs best across all experiments.
更多查看译文
关键词
generalized matrix learning vector quantization,GMLVQ,optimization algorithm,gradient-based training,variance-based stochastic gradient descent algorithm
AI 理解论文
溯源树
样例
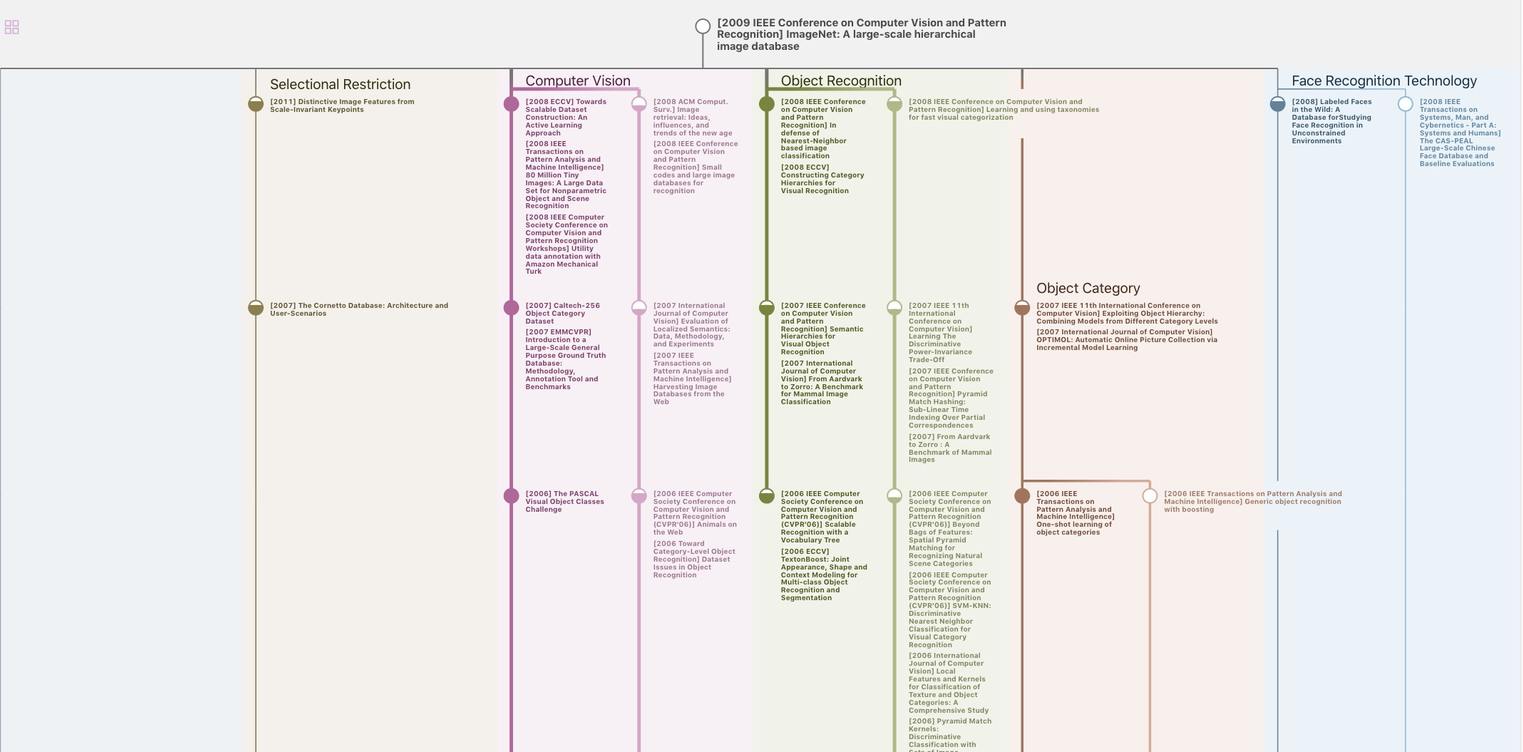
生成溯源树,研究论文发展脉络
Chat Paper
正在生成论文摘要