Propagating Maximum Capacities for Recommendation.
Lecture Notes in Artificial Intelligence(2017)
摘要
Neighborhood-based approaches often fail in sparse scenarios; a direct implication for recommender systems exploiting co-occurring items is often an inappropriately poor performance. As a remedy, we propose to propagate information (e.g., similarities) across the item graph to leverage sparse data. Instead of processing only directly connected items (e.g. co-occurrences), the similarity of two items is defined as the maximum capacity path interconnecting them. Our approach resembles a generalization of neighborhood-based methods that are obtained as special cases when restricting path lengths to one. We present two efficient online computation schemes and report on empirical results.
更多查看译文
关键词
Recommender systems,Information propagation,Maximum capacity paths,Co-occurrence,Sparsity,Cold-start problem
AI 理解论文
溯源树
样例
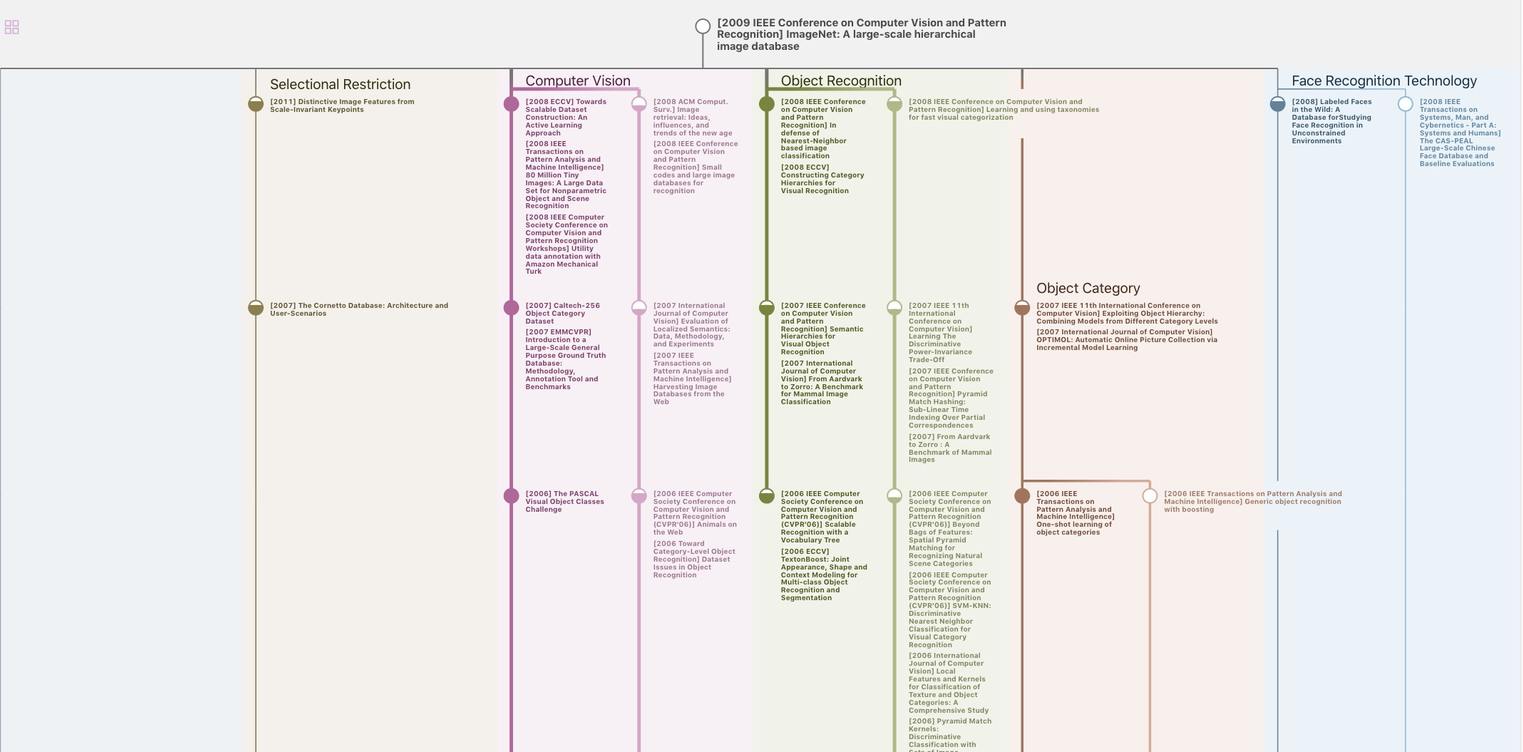
生成溯源树,研究论文发展脉络
Chat Paper
正在生成论文摘要