Stylized Adversarial AutoEncoder for Image Generation.
MM '17: ACM Multimedia Conference Mountain View California USA October, 2017(2017)
摘要
In this paper, we propose an autoencoder-based generative adversarial network (GAN) for automatic image generation, which is called "stylized adversarial autoencoder". Different from existing generative autoencoders which typically impose a prior distribution over the latent vector, the proposed approach splits the latent variable into two components: style feature and content feature, both encoded from real images. The split of the latent vector enables us adjusting the content and the style of the generated image arbitrarily by choosing different exemplary images. In addition, a multiclass classifier is adopted in the GAN network as the discriminator, which makes the generated images more realistic. We performed experiments on hand-writing digits, scene text and face datasets, in which the stylized adversarial autoencoder achieves superior results for image generation as well as remarkably improves the corresponding supervised recognition task.
更多查看译文
关键词
Image Generation, Generative Adversarial Network, AutoEncoder
AI 理解论文
溯源树
样例
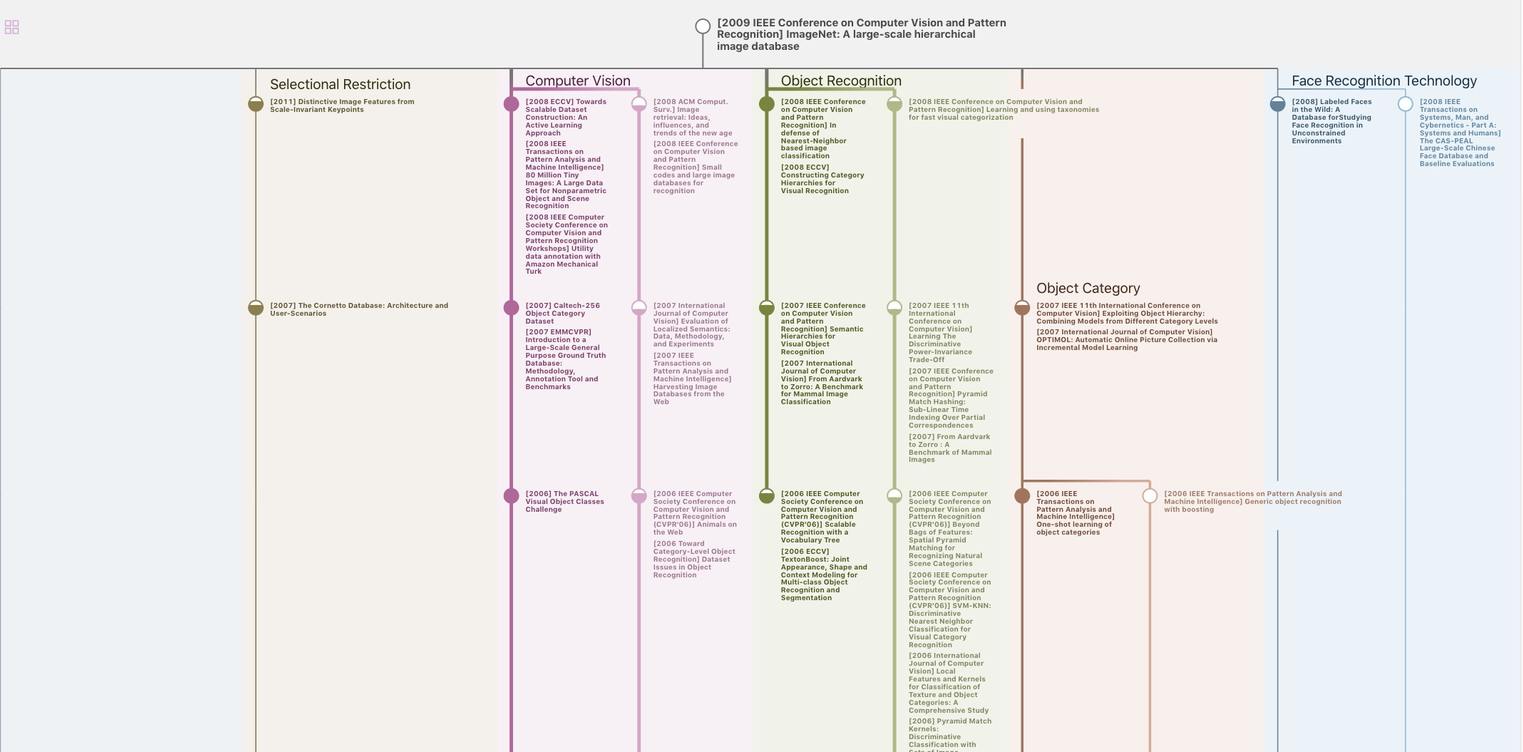
生成溯源树,研究论文发展脉络
Chat Paper
正在生成论文摘要