Identifying Subnetwork Fingerprints In Structural Connectomes: A Data-Driven Approach
CONNECTOMICS IN NEUROIMAGING(2017)
摘要
Identifying white matter connectivity patterns in the human brain derived from neuroimaging data is an important area of research in computational medicine. Recently, machine learning techniques typically use region-to-region or hub-base connectivity features to understand how the brain is organized, and then use this information to predict the clinical outcome. Unfortunately, computational models that are trained with these types of features are very localized to a particular region in the brain, i.e. one particular brain region or two connected brain regions, and may not provide the level of information needed to understand more complex relationships that span multiple connected brain regions. To overcome this limitation a new subnetwork feature is introduced that combine region-to-region and hub-based delay information using the shortest path algorithm. The proposed feature is then used to construct a deep learning model to recognize the identity of 20 different subjects. The results show person identification models trained with our feature are approximately 30% and 50% more accurate than models trained only using hub-based features and region-to-region features, respectively. Lastly, a connectome fingerprint is identified using a neural network backtrack approach that selects the subnetwork features that are responsible for classification performance.
更多查看译文
AI 理解论文
溯源树
样例
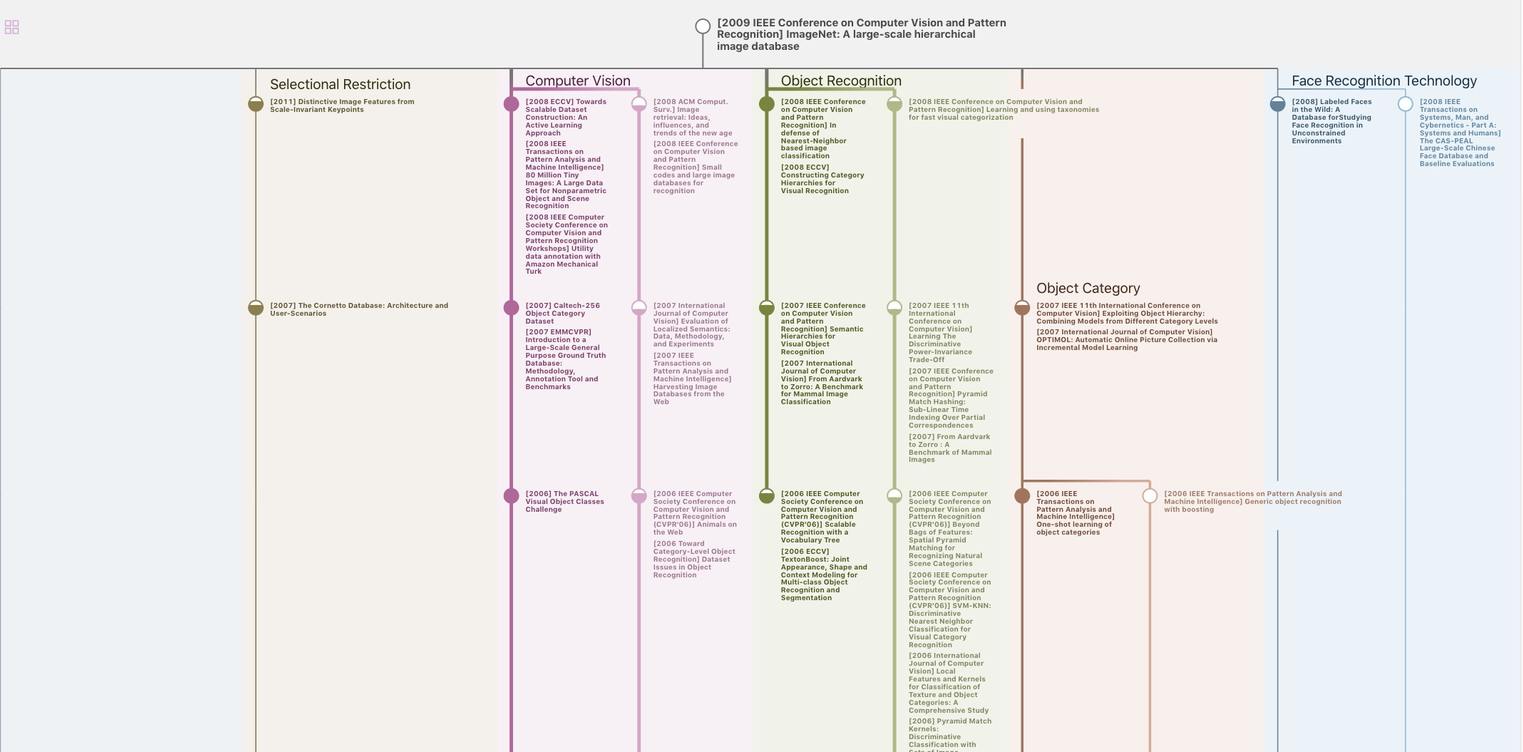
生成溯源树,研究论文发展脉络
Chat Paper
正在生成论文摘要