A Deep Convolutional Neural Network Approach For Complexity Reduction On Intra-Mode Hevc
2017 IEEE INTERNATIONAL CONFERENCE ON MULTIMEDIA AND EXPO (ICME)(2017)
摘要
The High Efficiency Video Coding (HEVC) standard significantly saves coding bit-rate over the proceeding H.264 standard, but at the expense of extremely high encoding complexity. In fact, the coding tree unit (CTU) partition consumes a large proportion of HEVC encoding complexity, due to the brute-force search for rate-distortion optimization (RDO). Therefore, we propose in this paper a complexity reduction approach for intra-mode HEVC, which learns a deep convolutional neural network (CNN) model to predict CTU partition instead of RDO. Firstly, we establish a large-scale database with diversiform patterns of CTU partition. Secondly, we model the partition as a three-level classification problem. Then, for solving the classification problem, we develop a deep CNN structure with various sizes of convolutional kernels and extensive trainable parameters, which can be learnt from the established database. Finally, experimental results show that our approach reduces intra-mode encoding time by 62.25% and 69.06% with negligible Bjontegaard delta bit-rate of 2.12% and 1.38%, over the test sequences and images respectively, superior to other state-of-the-art approaches.
更多查看译文
关键词
High efficiency video coding, intra-mode coding, complexity reduction, convolutional neural network
AI 理解论文
溯源树
样例
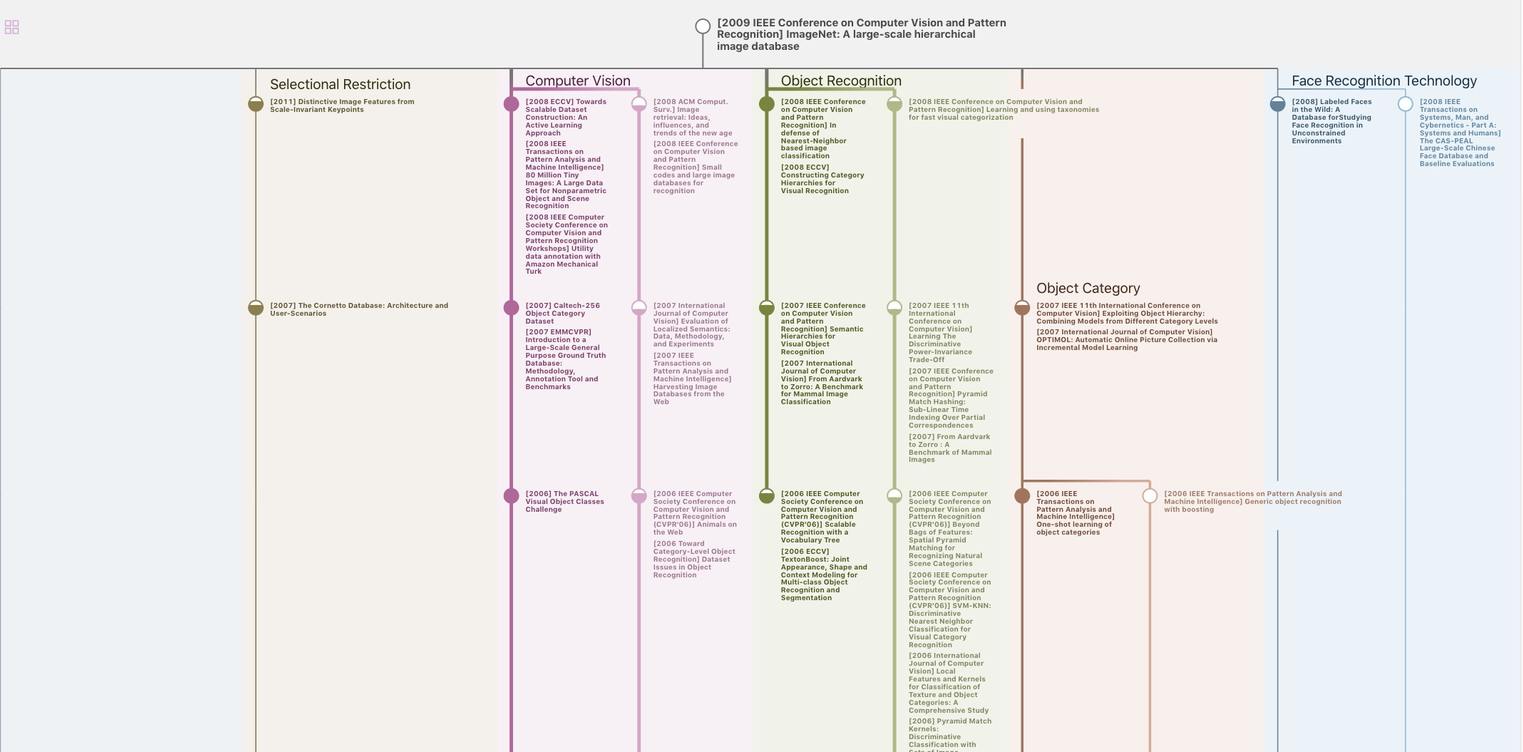
生成溯源树,研究论文发展脉络
Chat Paper
正在生成论文摘要