Efficient Privacy-preserving Adversarial Learning in Decentralized Online Social Networks.
ASONAM '17: Advances in Social Networks Analysis and Mining 2017 Sydney Australia July, 2017(2017)
摘要
In the last decade we have witnessed a more than prolific growth of online social media content in sites designed for online social interactions. These systems have been traditionally designed as centralized silos, which unfortunately suffer from abusive behavior ranging from spam, cyberbullying to even censorship. This paper investigates the utility of supervised learning techniques for abuse detection in future decentralized settings, where less metadata remains available for use in learning algorithms. We present a method that uses a privacy-preserving protocol to exchange a fingerprint of the neighborhood of a pair of nodes, namely sender and receiver. Our method extracts social graph metadata to form a subset of key features, namely neighborhood knowledge, some of which we approximate to reduce communication and computational requirements of such a protocol. In our benchmarking we show that a data minimization approach can obtain features 13% faster while providing similar or, as with the SVM classifier, even better abuse detection rates with just approximated Private Set Intersection.
更多查看译文
关键词
decentralized online social networks,adversarial learning,social networks,privacy-preserving
AI 理解论文
溯源树
样例
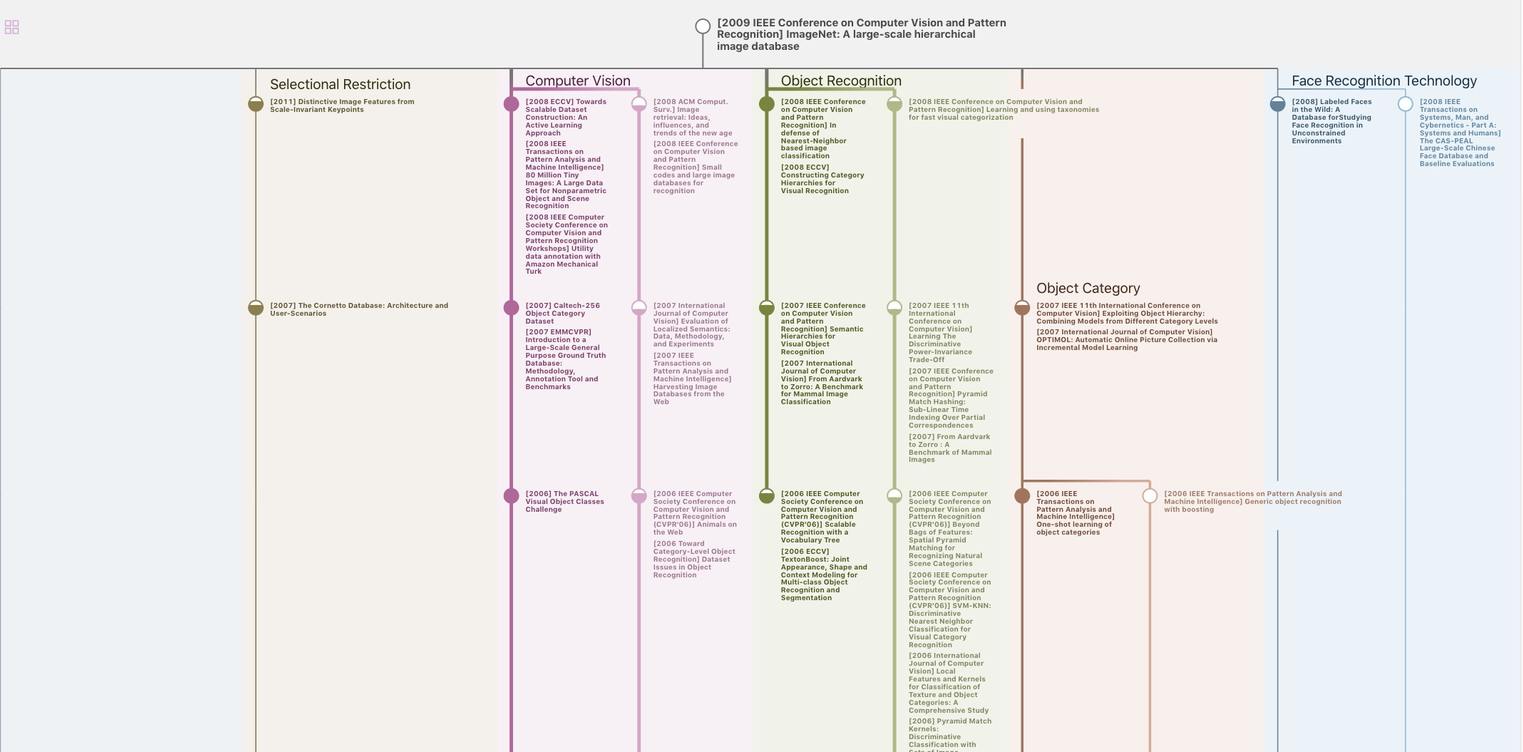
生成溯源树,研究论文发展脉络
Chat Paper
正在生成论文摘要