DepthRank: Exploiting Temporality to Uncover Important Network Nodes
SocInfo(2017)
摘要
Identifying important network nodes is very crucial for a variety of applications, such as the spread of an idea or an innovation. The majority of the publications so far assume that the interactions between nodes are static. However, this approach neglects that real-world phenomena evolve in time. Thus, there is a need for tools and techniques which account for evolution over time. Towards this direction, we present a novel graph-based method, named DepthRank (DR) that incorporates the temporal characteristics of the underlying datasets. We compare our approach against two baseline methods and find that it efficiently recovers important nodes on three real world datasets, as indicated by the numerical simulations. Moreover, we perform our analysis on a modified version of the DBLP dataset and verify its correctness using ground truth data.
更多查看译文
关键词
Influence detection,Network analysis,Temporal awareness
AI 理解论文
溯源树
样例
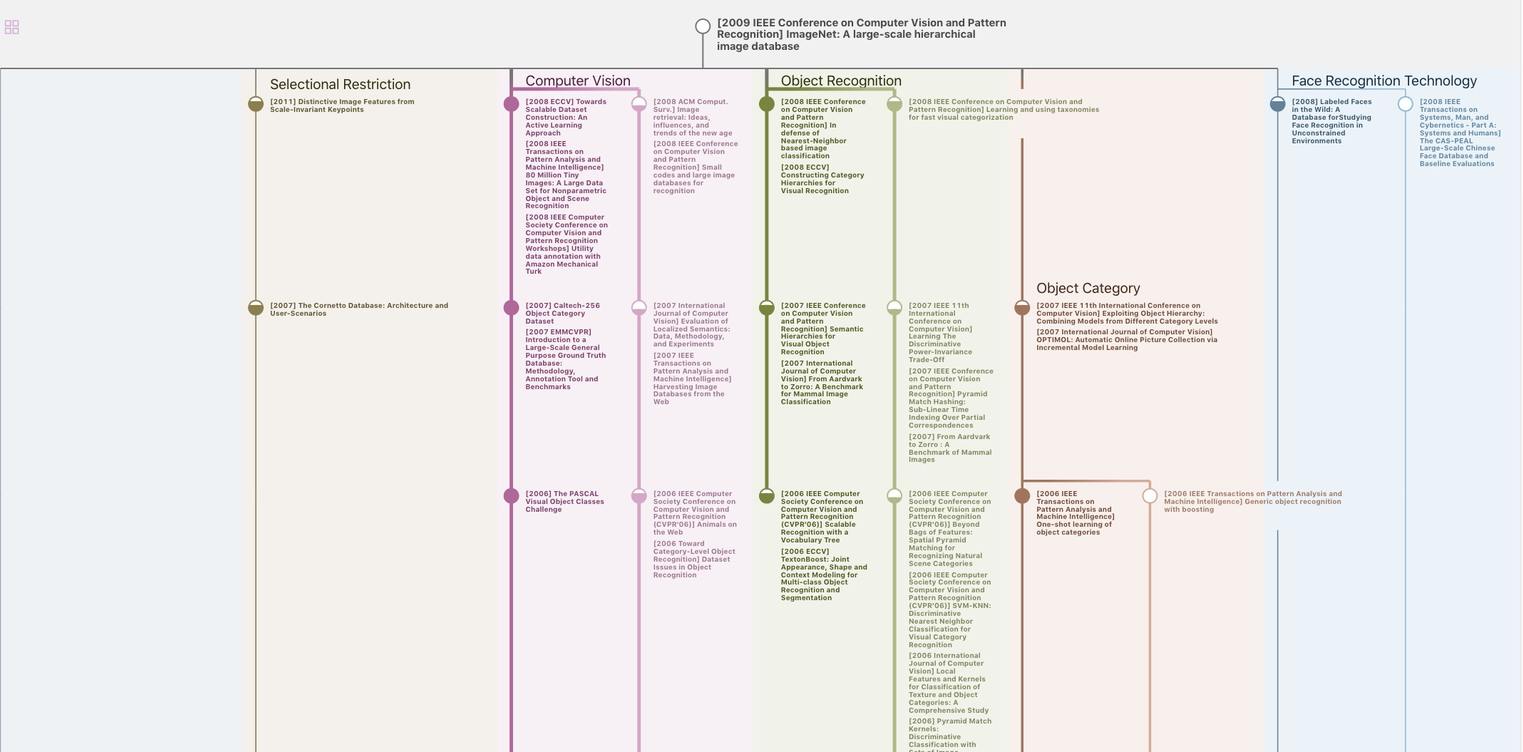
生成溯源树,研究论文发展脉络
Chat Paper
正在生成论文摘要