Measuring Ambient Population from Location-Based Social Networks to Describe Urban Crime.
SocInfo(2017)
摘要
Recently, a lot of attention has been given to crime prediction, both by the general public and by the research community. Most of the latest work has concentrated on showing the potential of novel data sources like social media, mobile phone data, points of interest, or transportation data for the crime prediction task and researchers have focused mostly on techniques from supervised machine learning to show their predictive potential. Yet, the question remains if indeed this data can be used to better describe urban crime. In this paper, we investigate the potential of data harvested from location-based social networks (specifically Foursquare) to describe urban crime. Towards this end, we apply techniques from spatial econometrics. We show that this data, seen as a measurement for the ambient population of a neighborhood, is able to further describe crime levels in comparison to models built solely on census data, seen as measurement for the resident population of a neighborhood. In an analysis of crime on census tract level in New York City, the total number of incidents can be described by our models with up to (R^2 = 56%), while the best model for the different crime subtypes is achieved for larcenies with roughly (67%) of the variance explained.
更多查看译文
关键词
Urban computing, Social computing, Computational social science, Crime analysis, Spatial econometrics, Location-based social networks
AI 理解论文
溯源树
样例
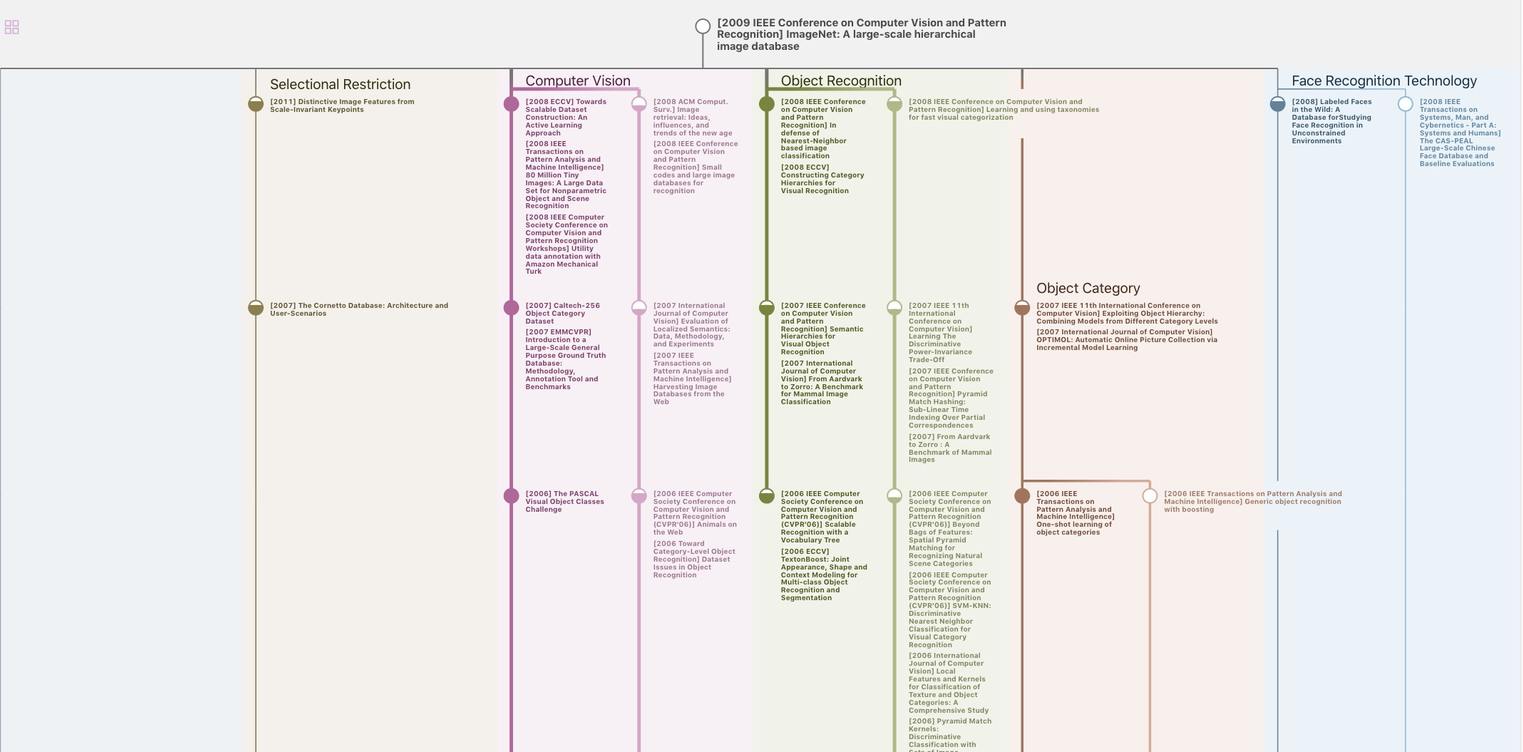
生成溯源树,研究论文发展脉络
Chat Paper
正在生成论文摘要