A Deep Learning Approach For Optimizing Content Delivering In Cache-Enabled Hetnet
2017 INTERNATIONAL SYMPOSIUM ON WIRELESS COMMUNICATION SYSTEMS (ISWCS)(2017)
摘要
In ultra-dense heterogeneous networks, caching popular contents at small base stations is considered as an effective way to reduce latency and redundant data transmission. Optimization of caching placement/replacement and content delivering can be computationally heavy, especially for large-scale networks. The provision of both time-efficient and high-quality solutions is challenging. Conventional iterative optimization methods, either optimal or heuristic, typically require a large number of iterations to achieve satisfactory performance, and result in considerable computational delay. This may limit their applications in practical network operations where online decisions have to be made. In this paper, we provide a viable alternative to the conventional methods for caching optimization, from a deep learning perspective. The idea is to train the optimization algorithms through a deep neural network (DNN) in advance, instead of directly applying them in real-time caching or scheduling. This allows significant complexity reduction in the delay-sensitive operation phase since the computational burden is shifted to the DDN training phase. Numerical results demonstrate that the DNN is of high computational efficiency. By training the designed DNN over a massive number of instances, the solution quality of the energy-efficient content delivering can be progressively approximated to around 90% of the optimum.
更多查看译文
关键词
Deep neural network, caching, energy optimization, user clustering, heterogeneous network
AI 理解论文
溯源树
样例
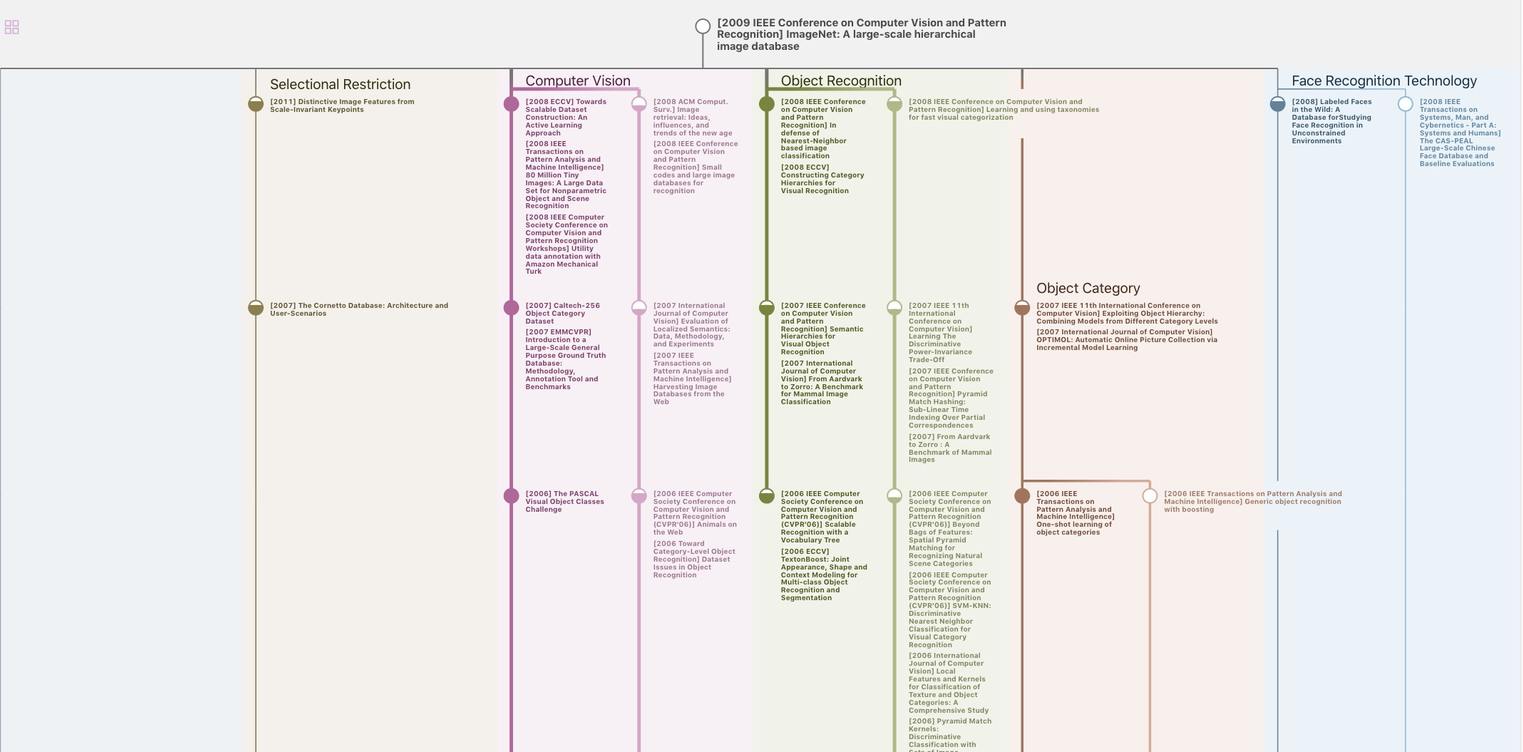
生成溯源树,研究论文发展脉络
Chat Paper
正在生成论文摘要