PULP: Achieving Privacy and Utility Trade-Off in User Mobility Data
2017 IEEE 36th Symposium on Reliable Distributed Systems (SRDS)(2017)
摘要
Leveraging location information in location-based services leads to improving service utility through geocontextualization. However, this raises privacy concerns as new knowledge can be inferred from location records, such as user's home and work places, or personal habits. Although Location Privacy Protection Mechanisms (LPPMs) provide a means to tackle this problem, they often require manual configuration posing significant challenges to service providers and users. Moreover, their impact on data privacy and utility is seldom assessed. In this paper, we present PULP, a model-driven system which automatically provides user-specific privacy protection and contributes to service utility via choosing adequate LPPM and configuring it. At the heart of PULP is nonlinear models that can capture the complex dependency of data privacy and utility for each individual user under given LPPM considered, i.e., Geo-Indistinguishability and Promesse. According to users' preferences on privacy and utility, PULP efficiently recommends suitable LPPM and corresponding configuration. We evaluate the accuracy of PULP's models and its effectiveness to achieve the privacy-utility trade-off per user, using four real-world mobility traces of 770 users in total. Our extensive experimentation shows that PULP ensures the contribution to location service while adhering to privacy constraints for a great percentage of users, and is orders of magnitude faster than non-model based alternatives.
更多查看译文
关键词
data privacy,model-driven system,user-specific privacy protection,user mobility data,location-based services,geocontextualization,LPPM,PULP models,Location Privacy Protection Mechanisms
AI 理解论文
溯源树
样例
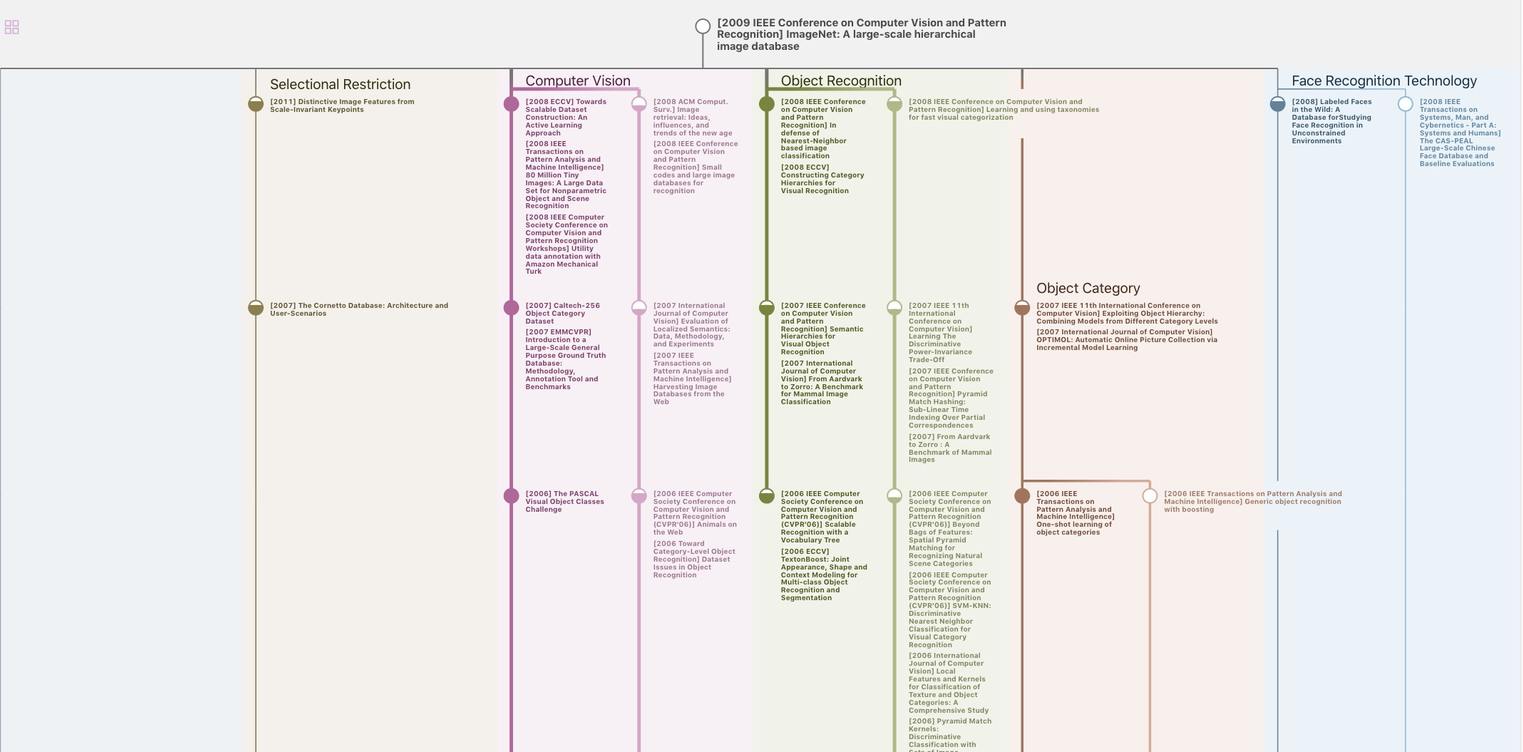
生成溯源树,研究论文发展脉络
Chat Paper
正在生成论文摘要