Region Representation Learning via Mobility Flow.
CIKM(2017)
摘要
Increasing amount of urban data are being accumulated and released to public; this enables us to study the urban dynamics and address urban issues such as crime, traffic, and quality of living. In this paper, we are interested in learning vector representations for regions using the large-scale taxi flow data. These representations could help us better measure the relationship strengths between regions, and the relationships can be used to better model the region properties. Different from existing studies, we propose to consider both temporal dynamics and multi-hop transitions in learning the region representations. We propose to jointly learn the representations from a flow graph and a spatial graph. Such a combined graph could simulate individual movements and also addresses the data sparsity issue. We demonstrate the effectiveness of our method using three different real datasets.
更多查看译文
关键词
mobility flow, graph embedding, spatial-temporal data
AI 理解论文
溯源树
样例
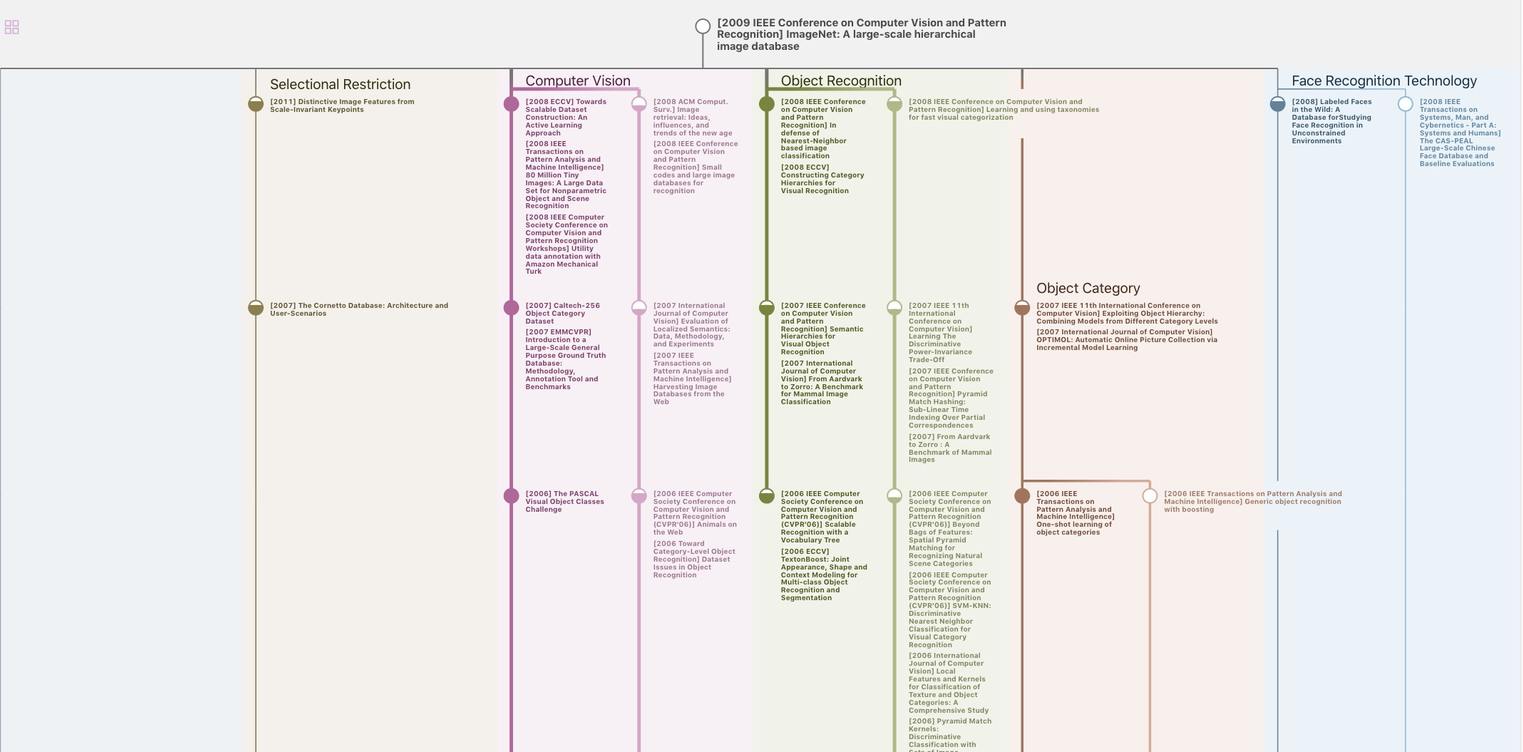
生成溯源树,研究论文发展脉络
Chat Paper
正在生成论文摘要