Learning Graph-based Embedding For Time-Aware Product Recommendation.
CIKM(2017)
摘要
In this paper, we propose a novel Product Graph Embedding (PGE) model to investigate time-aware product recommendation by leveraging the network representation learning technique. Our model captures the sequential influences of products by transforming the historical purchase records into a product graph. Then the product can be transformed into a low dimensional vector by the network embedding model. Once products are projected into the latent space, we present a novel method to compute user's latest preferences, which projects users into the same latent space as products. This method is based on time-decay functions and the embedding of sequential products that the user purchased. Thus, relatedness between a product and a user can be measured by the similarity between the embedding vectors which represent the product and the user's preferences. The experimental results on purchase records crawled from JINGDONG, show the superiority of our proposed framework for personalized product recommendation.
更多查看译文
关键词
Network Embedding, Product Recommendation, Dynamic User Embedding, Time Aware
AI 理解论文
溯源树
样例
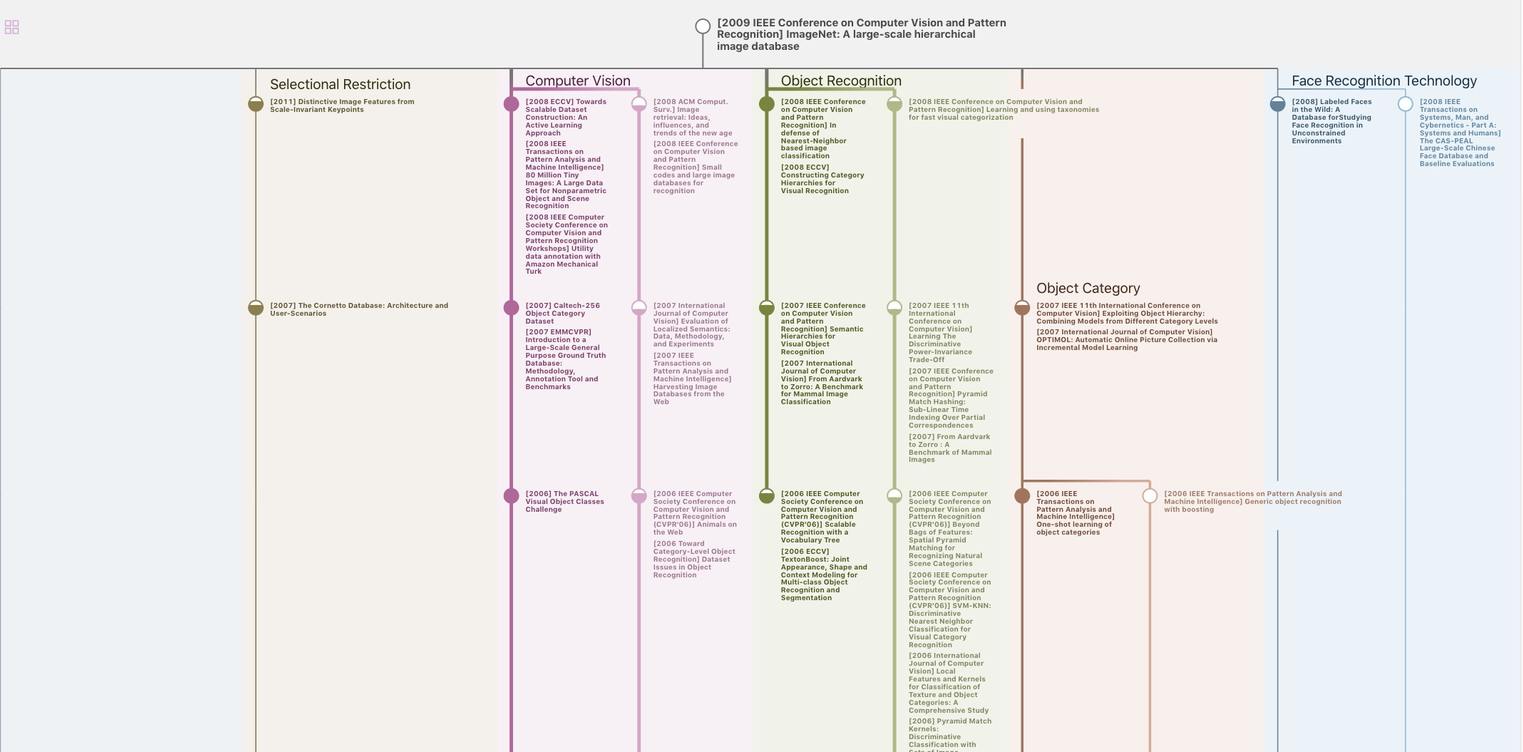
生成溯源树,研究论文发展脉络
Chat Paper
正在生成论文摘要