An Ad CTR Prediction Method Based on Feature Learning of Deep and Shallow Layers.
CIKM(2017)
摘要
In online advertising, Click-Through Rate (CTR) prediction is a crucial task, as it may benefit the ranking and pricing of online ads. To the best of our knowledge, most of the existing CTR prediction methods are shallow layer models (e.g., Logistic Regression and Factorization Machines) or deep layer models (e.g., Neural Networks). Unfortunately, the shallow layer models cannot capture or utilize high-order nonlinear features in ad data. On the other side, the deep layer models cannot satisfy the necessity of updating CTR models online efficiently due to their high computational complexity. To address the shortcomings above, in this paper, we propose a novel hybrid method based on feature learning of both Deep and Shallow Layers (DSL). In DSL, we utilize Deep Neural Network as a deep layer model trained offline to learn high-order nonlinear features and use Factorization Machines as a shallow layer model for CTR prediction. Furthermore, we also develop an online learning implementation based on DSL, i.e., onlineDSL. Extensive experiments on large-scale real-world datasets clearly validate the effectiveness of our DSL method and onlineDSL algorithm compared with several state-of-the-art baselines.
更多查看译文
关键词
Online Advertising, CTR Prediction, Feature Learning
AI 理解论文
溯源树
样例
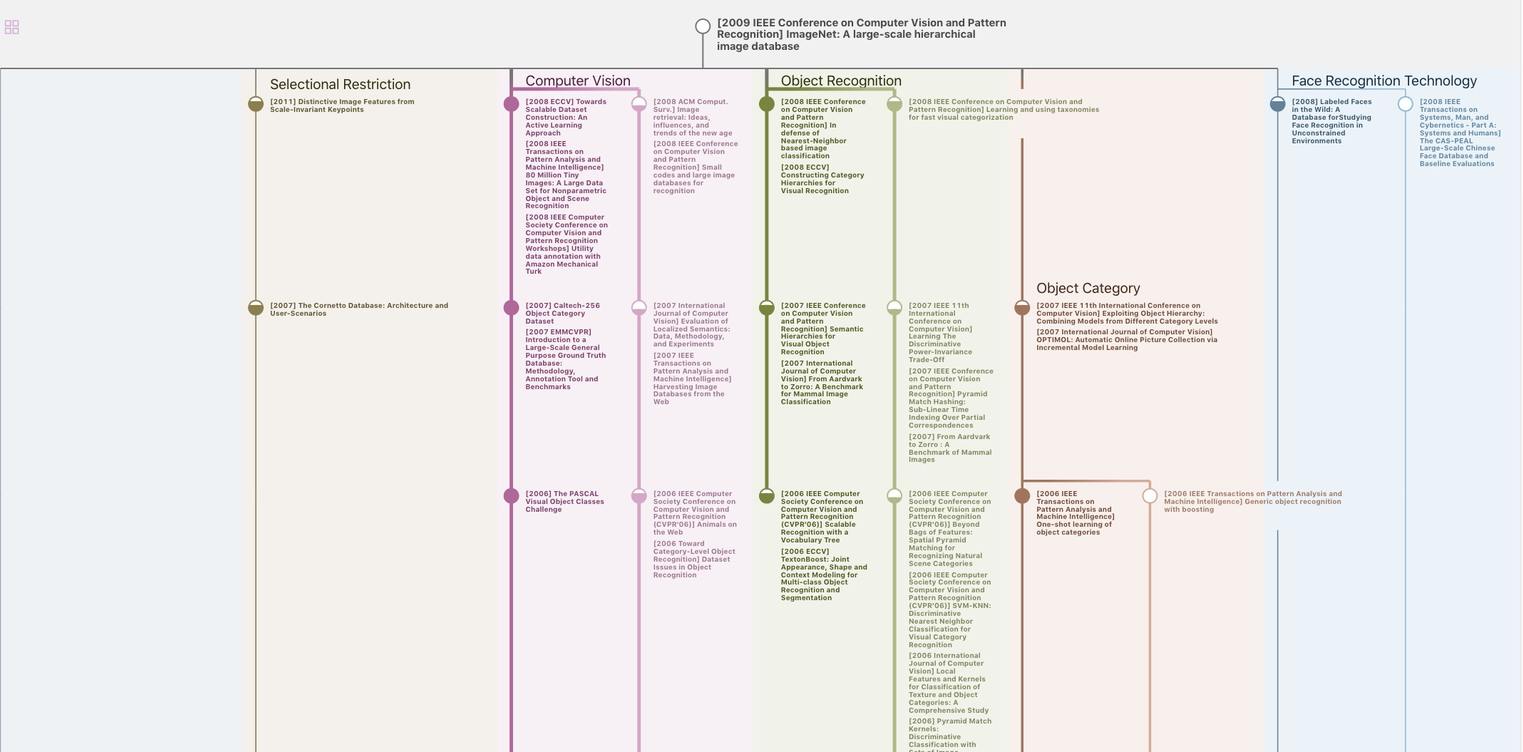
生成溯源树,研究论文发展脉络
Chat Paper
正在生成论文摘要