Accurate Sentence Matching with Hybrid Siamese Networks.
CIKM(2017)
摘要
Recent neural network approaches to sentence matching compute the probability of two sentences being similar by minimizing a logistic loss. In this paper, we learn sentence representations by means of a siamese network, which: (i) uses encoders that share parameters; and (ii) enables the comparison between two sentences in terms of their euclidean distance, by minimizing a contrastive loss. Moreover, we add a multilayer perceptron in the architecture to simultaneously optimize the contrastive and the logistic losses. This way, our network can exploit a more informative feedback, given by the logistic loss, which is also quantified by the distance that the two sentences have according to their representation in the euclidean space. We show that jointly minimizing the two losses yields higher accuracy than minimizing them independently. We verify this finding by evaluating several baseline architectures in two sentence matching tasks: question paraphrasing and textual entailment recognition. Our network approaches the state of the art, while being much simpler and faster to train, and with less parameters than its competitors.
更多查看译文
AI 理解论文
溯源树
样例
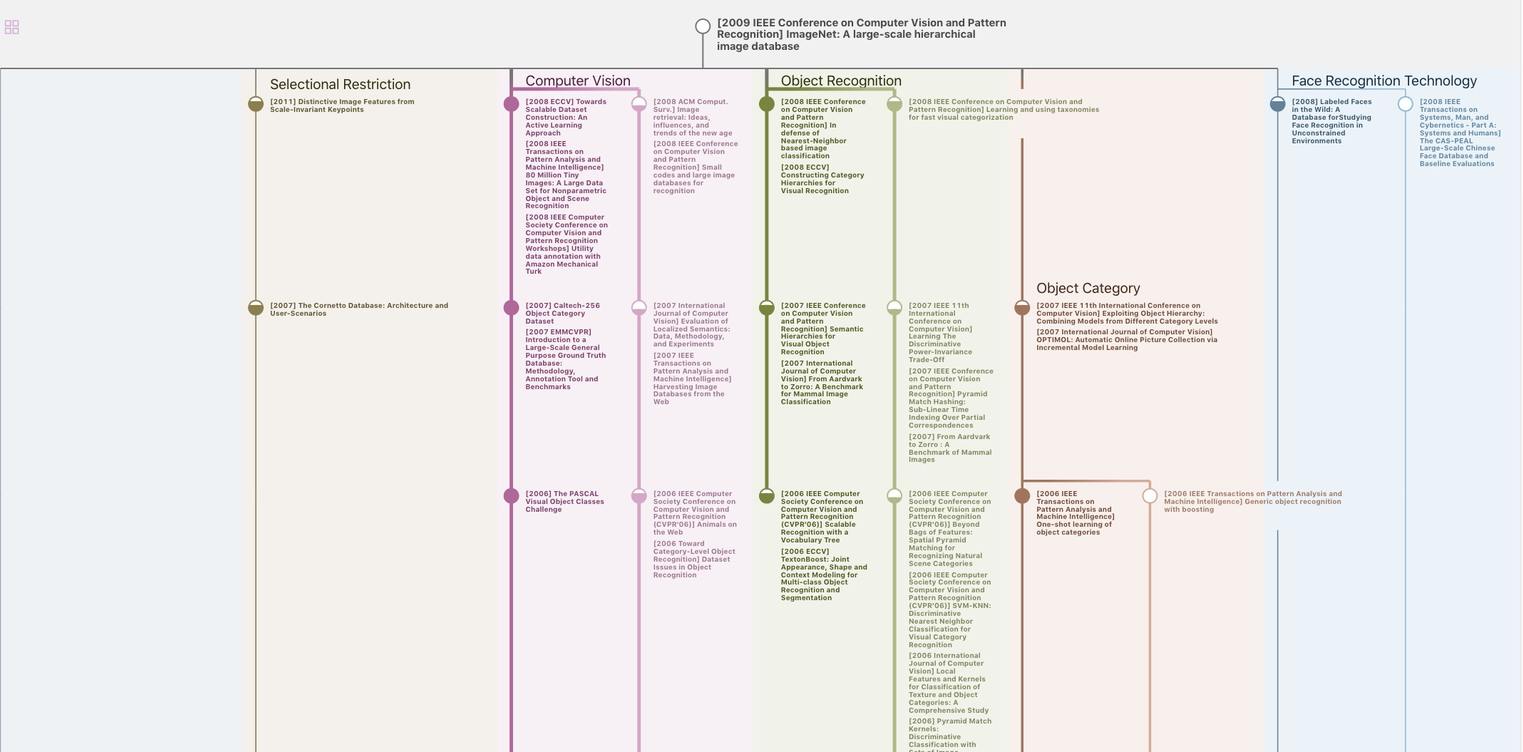
生成溯源树,研究论文发展脉络
Chat Paper
正在生成论文摘要