Study Of Electroencephalographic Signal Regularity For Automatic Emotion Recognition
UBIQUITOUS COMPUTING AND AMBIENT INTELLIGENCE, UCAMI 2017(2017)
摘要
Nowadays, emotional intelligence plays a key role in improving human-machine interaction (HMI). The main objective of HMI is to fill the gap between human emotional states and the reaction of a computer in accordance with this feeling. However, there is a lack of mathematical emotional models to implement affective computing systems into real applications. Consequently, this paper explores the properties of the nonlinear methodology based on Quadratic Sample Entropy (QSE) for the recognition of different emotional subspaces. Precisely, 665 segments of 32-channel electroencephalographic recordings from 32 subjects elicited with different emotional stimuli have been analyzed to validate the proposed model. Results conclude that QSE is a promising feature to be taken into account. Indeed, this metric has reported a discriminant ability around 72% using a support vector machine classifier. This result is comparable with the outcomes reported by other more complex methodologies which use multi-parametric analysis.
更多查看译文
关键词
Electroencephalography,Emotions,Physiological computing,Nonlinear,Quadratic Sample Entropy
AI 理解论文
溯源树
样例
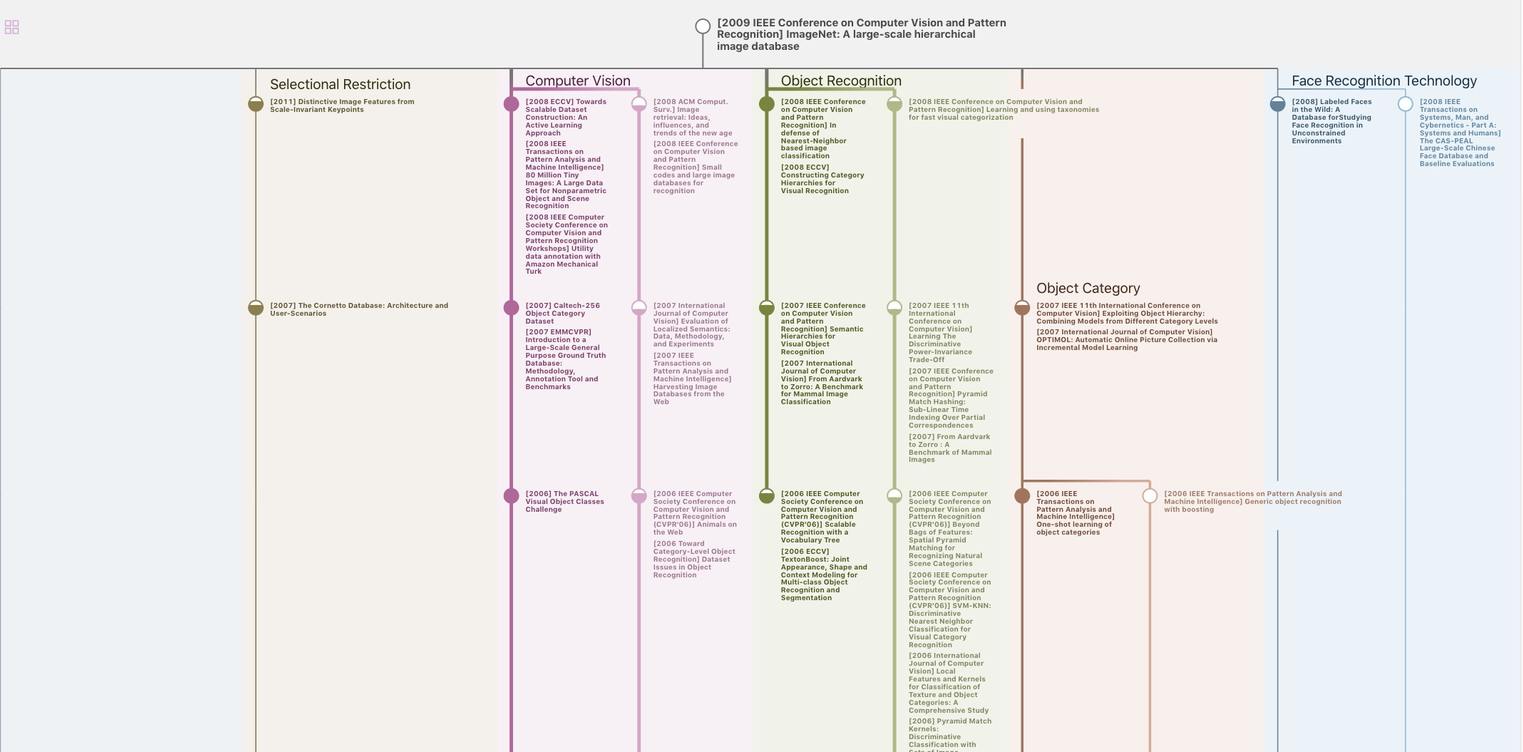
生成溯源树,研究论文发展脉络
Chat Paper
正在生成论文摘要