Matrix Factorization on Semantic Trajectories for Predicting Future Semantic Locations
2017 IEEE 13th International Conference on Wireless and Mobile Computing, Networking and Communications (WiMob)(2017)
摘要
With over 1 billion vehicles in operation over the world(1) and steadily growing cities, intelligent traffic management has become inevitable in order to preserve quality of life as we know it. Analyzing and predicting the movement behavior of traffic participants helps providing forward-looking solutions and plays a major role in intelligent traffic systems (ITS), in the field of location and handoff management, and in location aware systems in general. In this paper, we introduce a novel semantic location prediction approach that provides user-specific predictions based on their past semantic trajectories. For this purpose, we adopt and adapt an item recommendation method called FPMC. FPMC relies on a combination of Matrix Factorization and Markov Chains. We evaluate our algorithm against the user-independent standard Matrix Factorization (MF) and the Factorized Markov Chains (FMC) and show that our approach clearly surpasses the performance of the former mentioned methods.
更多查看译文
关键词
Intelligent transport systems,Proactive behavior,Mobility patterns,Context and location aware systems,Smart cities,Location prediction,Semantic trajectories,Matrix factorization,Markov Chains,Sequential pattern mining
AI 理解论文
溯源树
样例
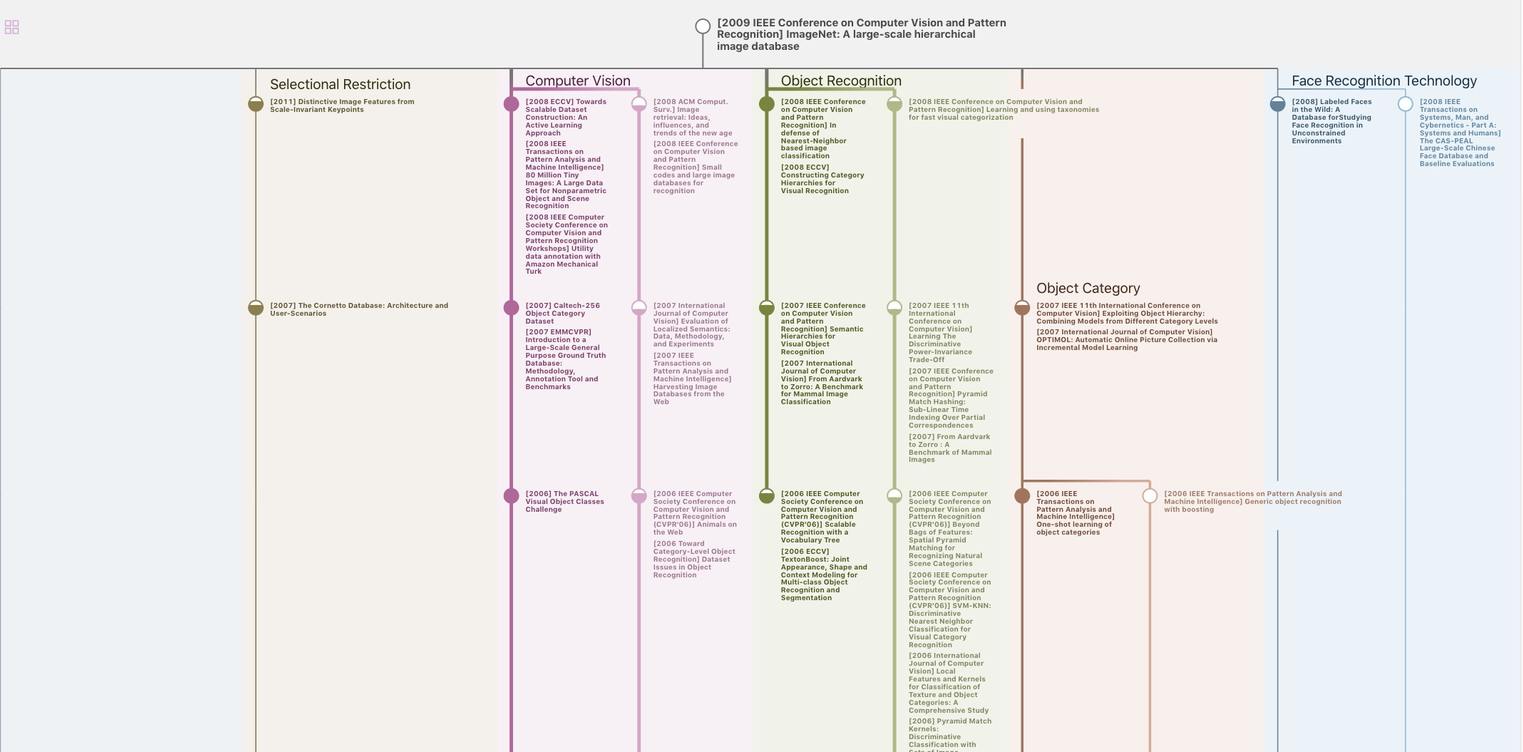
生成溯源树,研究论文发展脉络
Chat Paper
正在生成论文摘要