Comparison Of Similarity Approaches For Indoor Localization
2017 IEEE 13TH INTERNATIONAL CONFERENCE ON WIRELESS AND MOBILE COMPUTING, NETWORKING AND COMMUNICATIONS (WIMOB)(2017)
摘要
This paper presents a comparison study of different similarity metrics used for RSSI fingerprint based indoor localization. These metrics are used for nearest neighbor search which is a crucial step in fingerprint localization system. Including Euclidean distance, Manhattan distance and Gauss distance, the present study compares the localization error respect to a proposed parameter named "error density". This latter is related to the location error and the size of the studied area. In addition, different methods of combining the locations of neighbors have been introduced to estimate the current position and their performances have been compared. Extensive implementation details are discussed and simulation is conducted to compare them. Obtained results show that the Kernel method combined with the weighted average exhibits the localization accuracy on the studied dataset.
更多查看译文
关键词
Positioning, WiFi fingerprinting, KNN, Kernel method, Euclidean distance, Manhattan distance, Nadaraya Watson Kernel Estimator
AI 理解论文
溯源树
样例
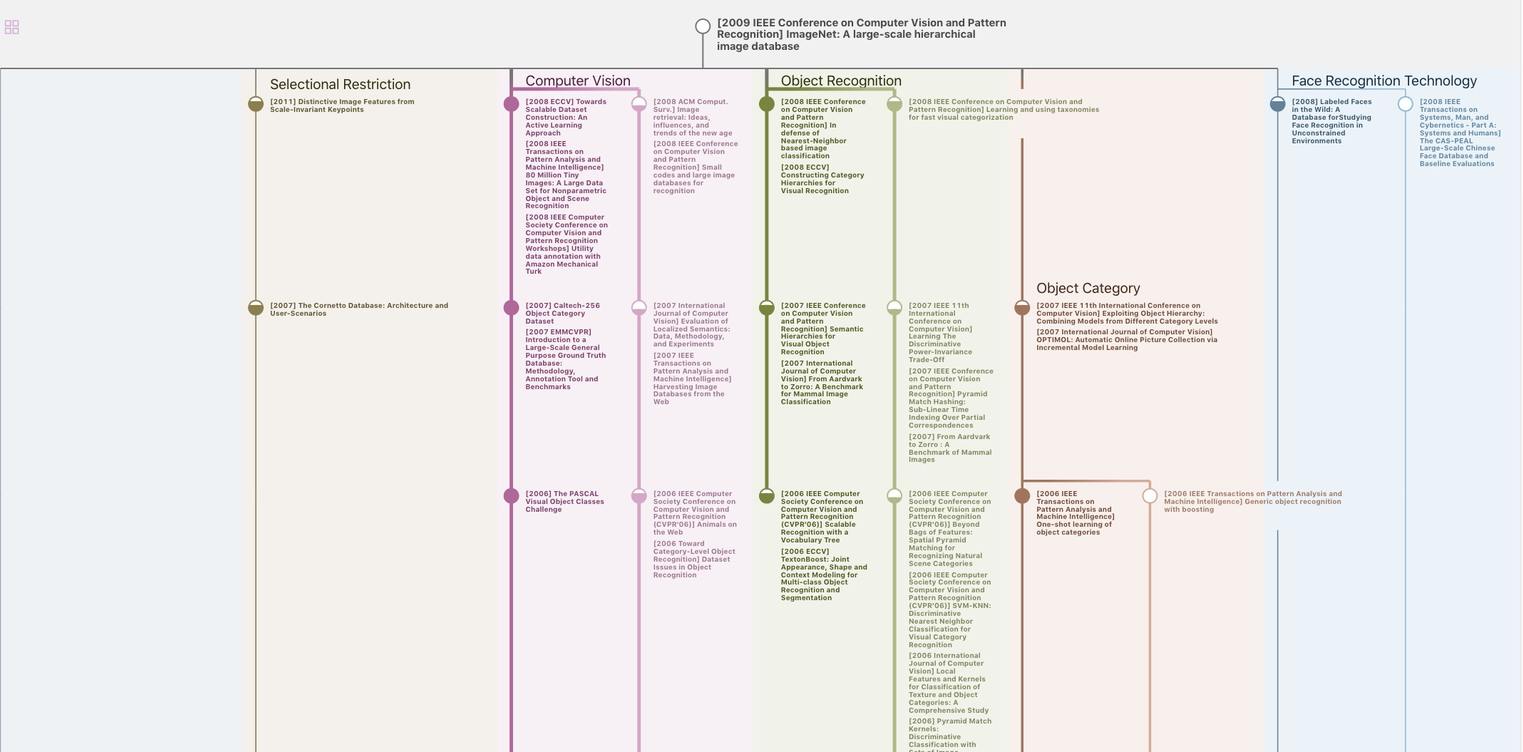
生成溯源树,研究论文发展脉络
Chat Paper
正在生成论文摘要