Distributed particle filtering under real-time constraints.
European Signal Processing Conference(2017)
摘要
Particle filters are powerful methods for state estimation in nonlinear/non-Gaussian dynamical systems. However due to the heavy computational requirements, they may not satisfy the real-time constraints in many applications requiring a large number of particles. By means of distributed implementation, real-time particle filtering can be achieved. However, the resampling stage in particle filters requires particle interaction which causes communication overhead. In this work, we propose a distributed resampling algorithm based on Butterfly Resampling previously described in the literature. We describe three interaction schemes (i) the complete interaction, (ii) the pairwise interaction where the nodes are constrained to communicate in pairs and (iii) the partial pairwise interaction in which only one pair is allowed to communicate. The goal is to diminish the communication cost in exchange for negligible loss of effective sample size. We conduct experiments on a cluster environment and compare our methods in terms of execution time, communication time and effective sample size. We find that the sparse interaction schemes show better performance for distributed systems and they keep the effective sample size nearly as high as the complete interaction scheme does.
更多查看译文
关键词
communication cost,execution time,communication time,sparse interaction schemes,distributed systems,complete interaction scheme,real-time constraints,state estimation,distributed implementation,real-time particle filtering,resampling stage,particle interaction,communication overhead,distributed resampling algorithm,Butterfly Resampling,partial pairwise interaction,distributed particle filtering,nonlinear-non-Gaussian dynamical systems
AI 理解论文
溯源树
样例
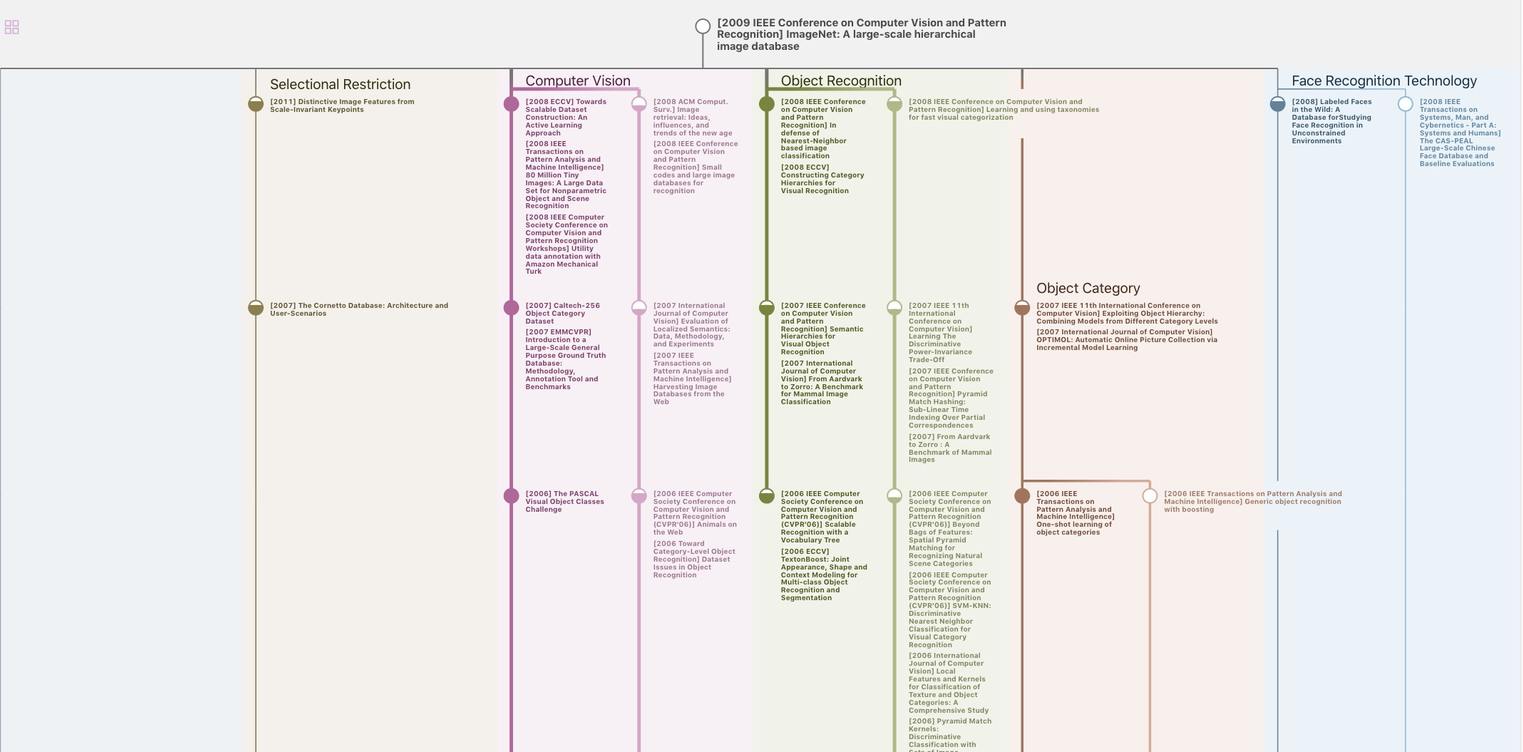
生成溯源树,研究论文发展脉络
Chat Paper
正在生成论文摘要