Compressive multispectral model for spectrum sensing in cognitive radio networks.
European Signal Processing Conference(2017)
摘要
Cognitive Radio (CR) is one of the most promising techniques for optimizing the spectrum usage. However, the large amount of data of spectral information that must be processed to identify and assign spectral resources increases the channel assignment times, therefore worsening the quality of service for the devices using the spectrum. Compressive Sensing (CS) is a digital processing technique that allows the reconstruction of sparse or compressible signals using fewer samples than those required traditionally. This paper presents a model that addresses the Spectral Sensing problem in Cognitive Radio using Compressive Sensing as an effective way of decreasing the number of samples required in the sensing process. This model is based on Compressive Spectral Imaging (CSI) architectures where a centralized spectrum manager selects what power data must be delivered by the different wireless devices using binary patterns, and builds a multispectral data cube image with the geographical and spectral data power information. The results show that this multispectral data cube can be built with only a 50% of the samples generated by the devices and, therefore reducing the data traffic dramatically.
更多查看译文
关键词
Compressive multispectral model,spectrum sensing,cognitive radio networks,spectrum usage,spectral information,spectral resources,channel assignment times,quality of service,Compressive Sensing,digital processing technique,sparse signals,compressible signals,Spectral Sensing problem,Compressive Spectral Imaging architectures,centralized spectrum manager,power data,multispectral data cube image,geographical data power information,spectral data power information,data traffic,wireless devices,binary patterns
AI 理解论文
溯源树
样例
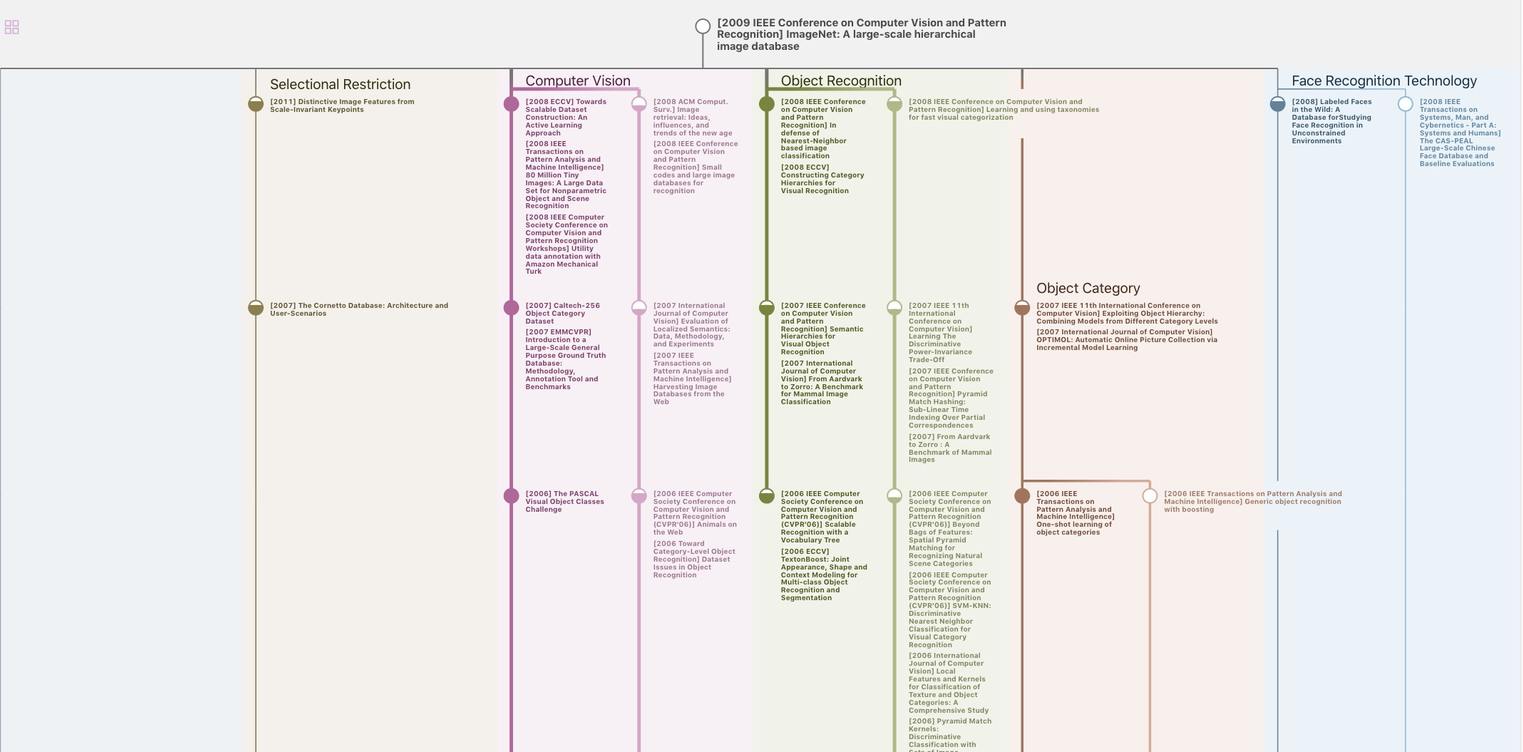
生成溯源树,研究论文发展脉络
Chat Paper
正在生成论文摘要