Automatic detection of bird species from audio field recordings using HMM-based modelling of frequency tracks.
European Signal Processing Conference(2017)
摘要
This paper presents an automatic system for detection of bird species in field recordings. A sinusoidal detection algorithm is employed to segment the acoustic scene into isolated spectro-temporal segments. Each segment is represented as a temporal sequence of frequencies of the detected sinusoid, referred to as frequency track. Each bird species is represented by a set of hidden Markov models (HMMs), each HMM modelling an individual type of bird vocalisation element. These HMMs are obtained in an unsupervised manner. The detection is based on a likelihood ratio of the test utterance against the target bird species and non-target background model. We explore on selection of cohort for modelling the background model, z-norm and t-norm score normalisation techniques and score compensation to deal with outlier data. Experiments are performed using over 40 hours of audio field recordings from 48 bird species plus an additional 16 hours of field recordings as impostor trials. Evaluations are performed using detection error trade-off plots. The equal error rate of 5% is achieved when impostor trials are non-target bird species vocalisations and 1.2% when using field recordings which do not contain bird vocalisations.
更多查看译文
关键词
bird species detection,field recording,hidden Markov model,HMM,score normalisation,cohort,outlier,vocalisation,element,unsupervised training,sinusoid detection,sinusoidal modelling,frequency track
AI 理解论文
溯源树
样例
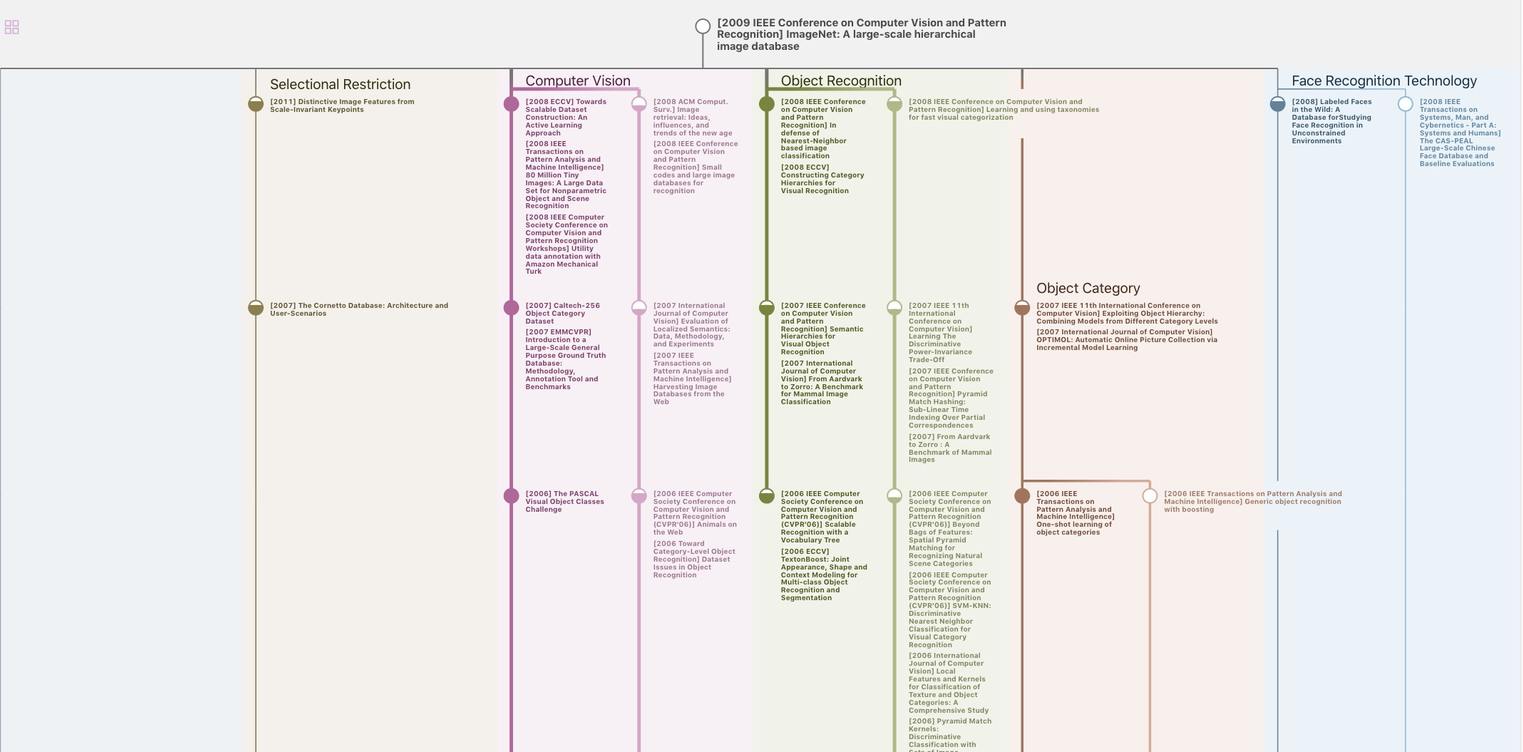
生成溯源树,研究论文发展脉络
Chat Paper
正在生成论文摘要