Complex Representations For Learning Statistical Shape Priors
2017 25TH EUROPEAN SIGNAL PROCESSING CONFERENCE (EUSIPCO)(2017)
摘要
Parametrisation of the shape of deformable objects is of paramount importance in many computer vision applications. Many state-of-the-art statistical deformable models perform landmark localisation via optimising an objective function over a certain parametrisation of the object's shape. Arguably, the most popular way is by employing statistical techniques. The points of shape samples of an object lie in a 2D lattice and they are normally represented by concatenating the 2D coordinates into a vector. As the 2D coordinates can be naturally represented as a complex number, in this paper we study statistical complex number representations of an object's shape. In particular, we show that the real representation provides a similar statistical prior as the widely linear complex model, while the circular complex representation results in a much more condensed encoding.
更多查看译文
关键词
complex representations,statistical shape priors,parametrisation,deformable objects,computer vision applications,statistical deformable models,landmark localisation,objective function,statistical techniques,shape samples,statistical complex number representations,widely linear complex model,circular complex representation results
AI 理解论文
溯源树
样例
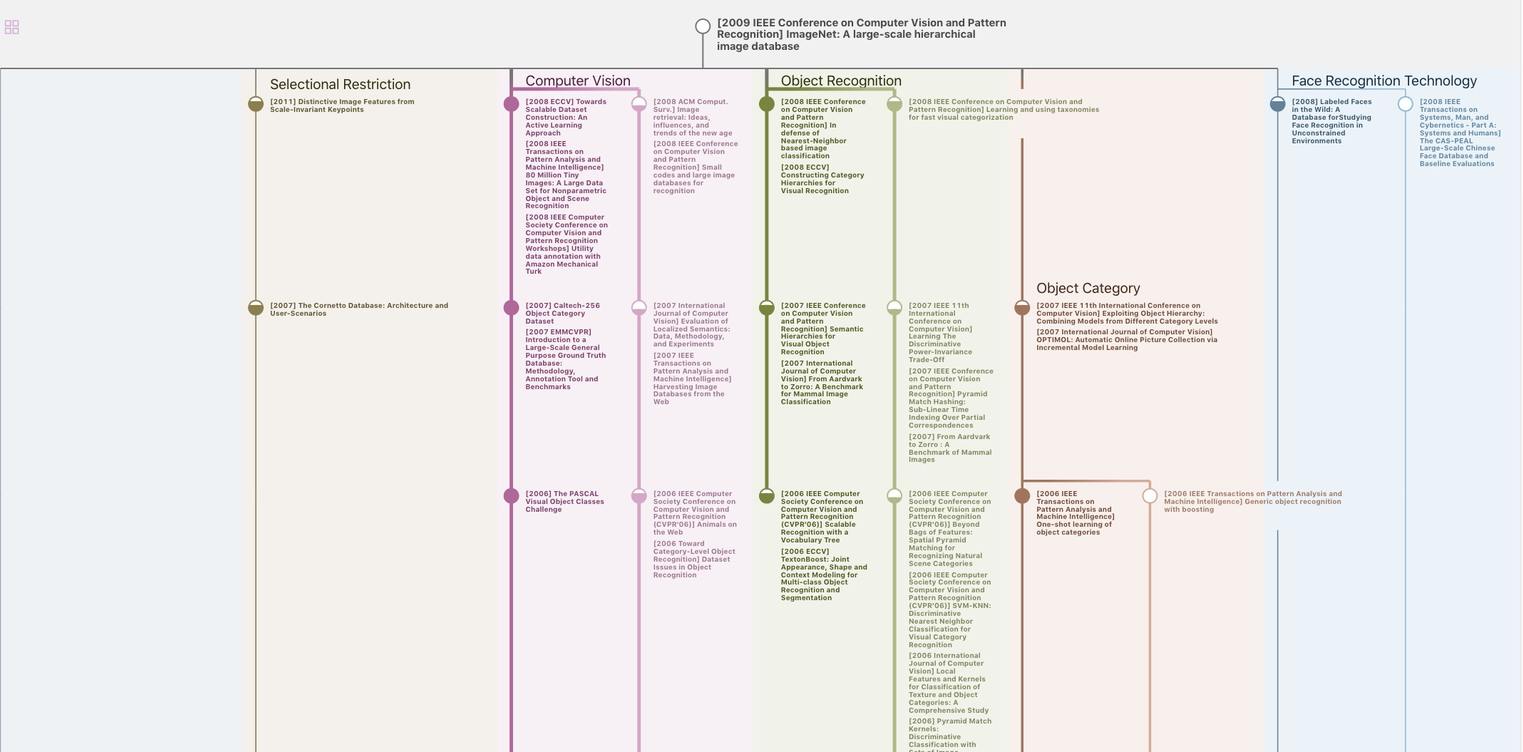
生成溯源树,研究论文发展脉络
Chat Paper
正在生成论文摘要