A Constant-Size Signature Scheme with Tighter Reduction from CDH Assumption
ISC(2017)
摘要
We present a signature scheme with the tightest security-reduction among known constant-size signature schemes secure under the computational Diffie-Hellman (CDH) assumption. It is important to reduce the security-reduction loss of a cryptosystem, which enables choosing of a smaller security parameter without compromising security; hence, enabling constant-size signatures for cryptosystems and faster computation. The tightest security reduction thus far from the CDH assumption is \(\mathcal {O}(q)\), presented by Hofheinz et al., where q is the number of signing queries. They also proved that the security loss of \(\mathcal {O}(q)\) is optimal if signature schemes are “re-randomizable”. In this paper, we revisit the non-re-randomizable signature scheme proposed by Böhl et al. Their signature scheme is the first that is fully secure under the CDH assumption and has a compact public key. However, they constructed the scheme with polynomial-order security-reduction loss. We first constructed a new existentially unforgeable against extended random-message attack (EUF-XRMA) secure scheme based on Böhl et al.’s scheme, which has tighter security reduction of \(\mathcal {O}(q/d)\) to the CDH assumption, where d is the number of group elements in a verification key. We then transformed the EUF-XRMA secure signature scheme into an existentially unforgeable against adaptively chosen-message attack (EUF-CMA) secure one using Abe et al.’s technique. In this construction, no pseudorandom function, which results in increase of reduction loss, is used, and the above reduction loss can be achieved. Moreover, a tag can be generated more efficiently than Böhl et al.’s signature scheme, which results in smaller computation. Consequently, our EUF-CMA secure scheme has tighter security reduction to the CDH assumption than any previous schemes.
更多查看译文
关键词
Digital signatures,CDH assumption,Trapdoor commitment,Tight security reduction
AI 理解论文
溯源树
样例
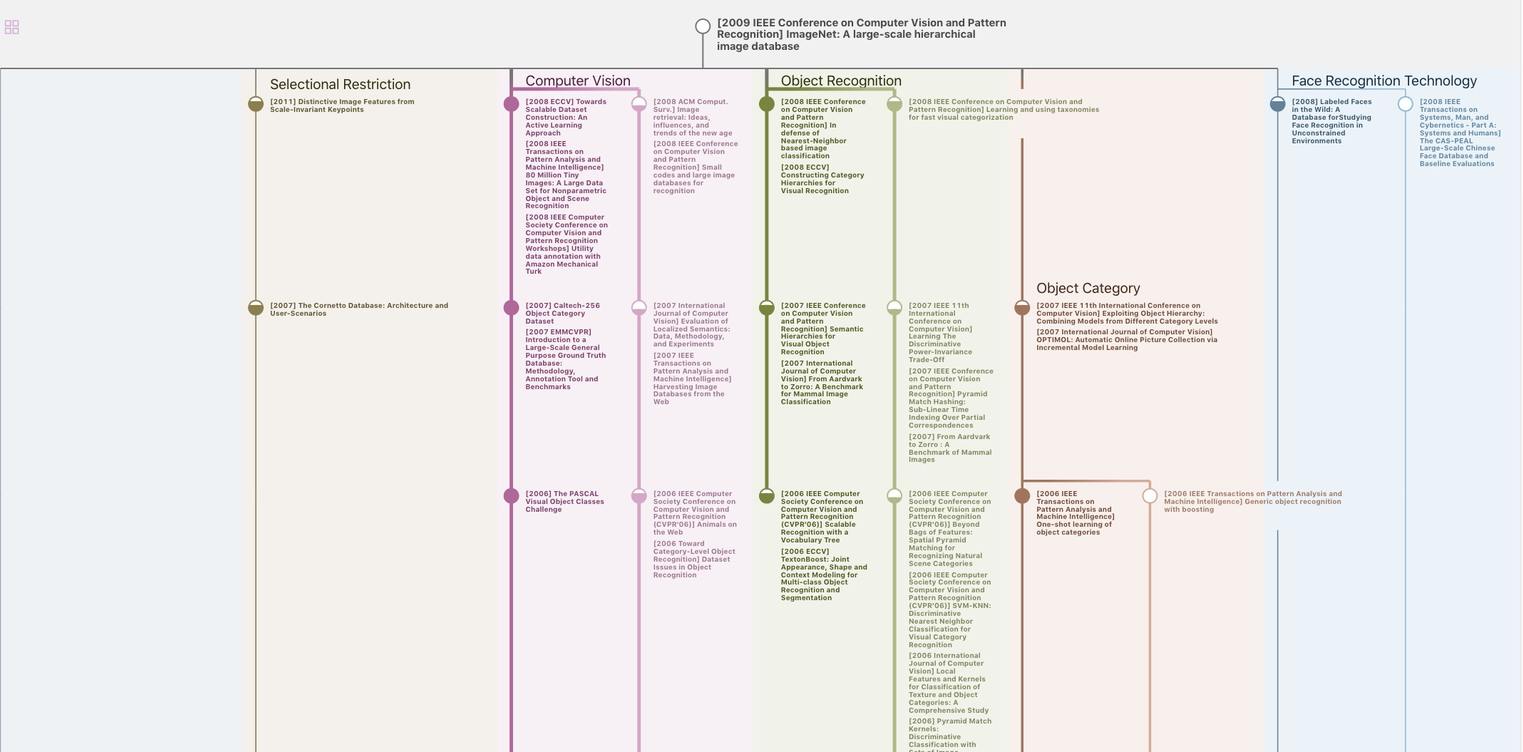
生成溯源树,研究论文发展脉络
Chat Paper
正在生成论文摘要