Dendritic Spine Classification Based On Two-Photon Microscopic Images Using Sparse Representation
2016 24TH SIGNAL PROCESSING AND COMMUNICATION APPLICATION CONFERENCE (SIU)(2016)
摘要
Dendritic spines, membranous protrusions of neurons, are one of the few prominent characteristics of neurons. Their shapes change with variations in neuron activity. Spine shape analysis plays a significant role in inferring the inherent relationship between neuron activity and spine morphology variations. First step towards integrating rich shape information is to classify spines into four shape classes reported in literature. This analysis is currently performed manually due to the deficiency of fully automated and reliable tools, which is a time intensive task with subjective results. Availability of automated analysis tools can expedite the analysis process. In this paper, we compare l(1) norm-based sparse representation based classification approach to the least squares method, and the l(2) -norm method for dendritic spine classification as well as to a morphological feature-based approach. On a dataset of 242 automatically segmented stubby and mushroom spines, l(1) representation with non-negativity constraint resulted in classification accuracy of 88.02%, which is the highest performance among the techniques considered here.
更多查看译文
关键词
Dendritic Spines,Classification,Sparse Representation,l(1),l(2),least-squares,Neuroimaging
AI 理解论文
溯源树
样例
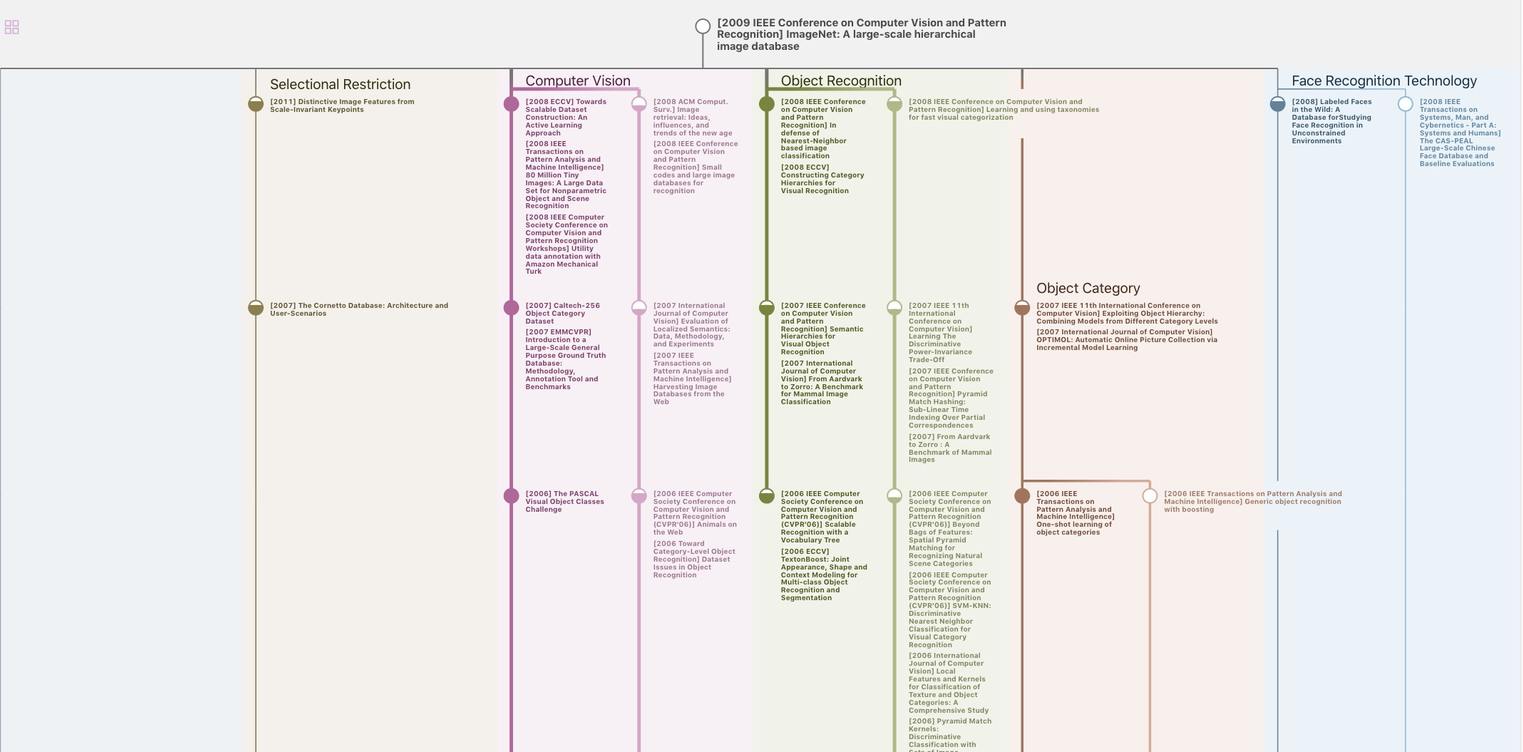
生成溯源树,研究论文发展脉络
Chat Paper
正在生成论文摘要