Behavior In Social Learning Networks: Early Detection For Online Short-Courses
IEEE INFOCOM 2017 - IEEE CONFERENCE ON COMPUTER COMMUNICATIONS(2017)
摘要
We study learning outcome prediction for online courses. Whereas prior work has focused on semester-long courses with frequent student assessments, we focus on short-courses that have single outcomes assigned by instructors at the end. The lack of performance data makes the behavior of learners, captured as they interact with course content and with one another in Social Learning Networks (SLN), essential for prediction. Our method defines several (machine) learning features based on behaviors collected on the modes of (human) learning in a course, and uses them in appropriate classifiers. Through evaluation on data captured from three two-week courses hosted through our delivery platforms, we make three key observations: (i) behavioral data is predictive of learning outcomes in short-courses (our classifiers achieving AUCs >= 0.8 after the two weeks), (ii) it has an early detection capability (AUCs >= 0.7 with the first week of data), and (iii) the content features have an "earliest" detection capability (with higher AUC in the first few days), while the SLN features become the more predictive set over time, as the network matures. We also discuss how our method can generate behavioral analytics for instructors.
更多查看译文
关键词
social learning networks,outcome prediction,semester-long courses,performance data,course content,two-week courses,behavioral data,early detection capability,behavioral analytics,student assessment,online short-courses
AI 理解论文
溯源树
样例
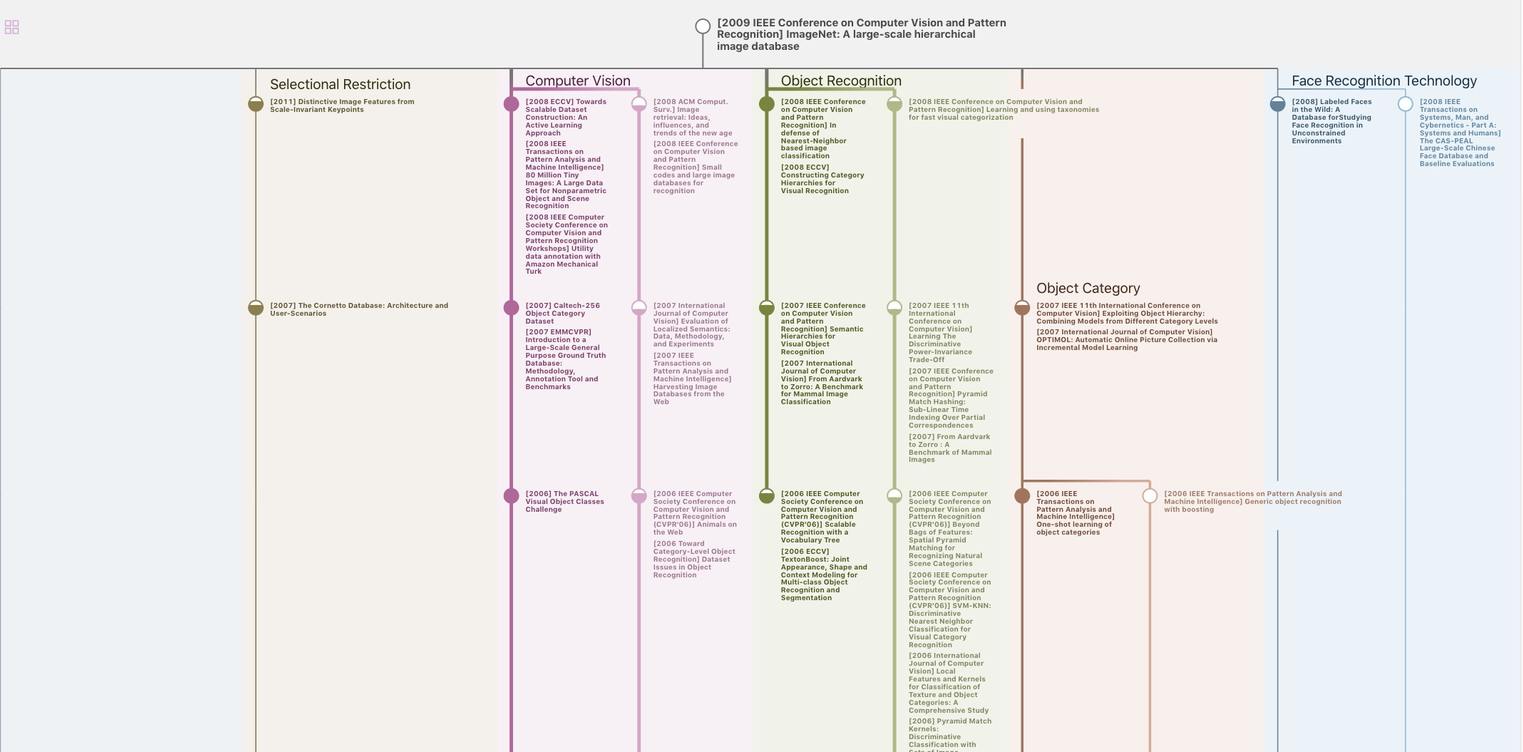
生成溯源树,研究论文发展脉络
Chat Paper
正在生成论文摘要