Multi-level structured hybrid forest for joint head detection and pose estimation.
Neurocomputing(2017)
摘要
In real-world applications, factors such as illumination variation, occlusion, and poor image quality, etc. make head detection and pose estimation much more challenging. In this paper, we propose a multi-level structured hybrid forest (MSHF) for joint head detection and pose estimation. Our method extends the hybrid framework of classification and regression forests by introducing multi-level splitting functions and multi-structural features. Multi-level splitting functions are used to construct trees in different layers of MSHF. Multi-structured features are extracted from randomly selected image patches, which are either head region or the background. The head contour is derived from these patches using the signed distance of the patch center to the head contour by MSHF regression. The randomly selected sub-regions from the patches within the head contour are used to develop the MSHF for head pose estimation in a coarse-to-fine manner. The weighted neighbor structured aggregation integrates votes from trees to achieve an estimation of continuous pose angles. Experiments were conducted using public datasets and video streams. Compared to the state-of-the-art methods, MSHF achieved improved performance and great robustness with an average accuracy of 90% and the average angular error of 6.6°. The averaged time for performing a joint head detection and pose estimation is about 0.44 s.
更多查看译文
关键词
Multi-level structured hybrid forest,Head pose estimation,Head detection,Joint detection-estimation,Multiple structured features
AI 理解论文
溯源树
样例
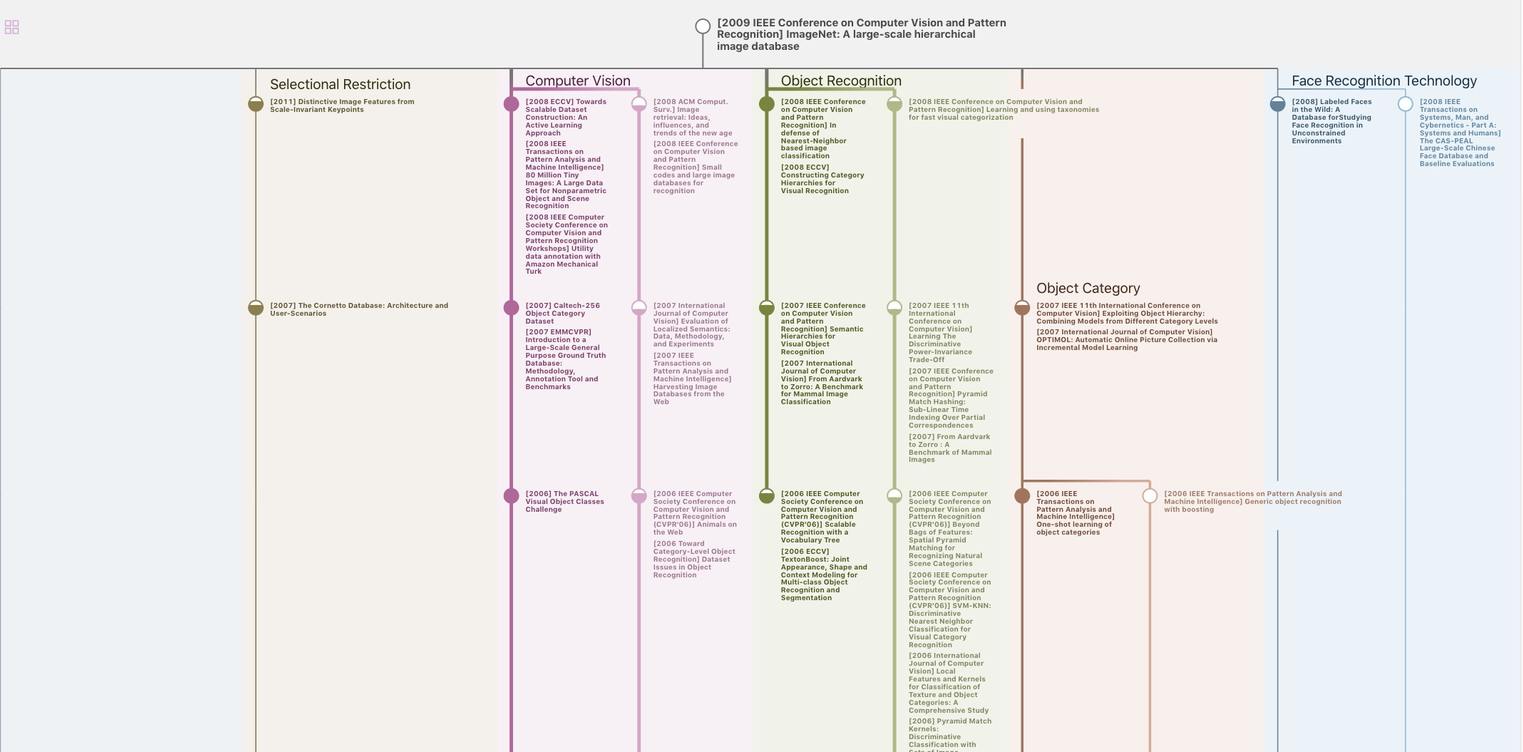
生成溯源树,研究论文发展脉络
Chat Paper
正在生成论文摘要